State-dependent importance sampling for estimating expectations of functionals of sums of independent random variables
arxiv(2023)
摘要
Estimating the expectations of functionals applied to sums of random variables (RVs) is a well-known problem encountered in many challenging applications. Generally, closed-form expressions of these quantities are out of reach. A naive Monte Carlo simulation is an alternative approach. However, this method requires numerous samples for rare event problems. Therefore, it is paramount to use variance reduction techniques to develop fast and efficient estimation methods. In this work, we use importance sampling (IS), known for its efficiency in requiring fewer computations to achieve the same accuracy requirements. We propose a state-dependent IS scheme based on a stochastic optimal control formulation, where the control is dependent on state and time. We aim to calculate rare event quantities that could be written as an expectation of a functional of the sums of independent RVs. The proposed algorithm is generic and can be applied without restrictions on the univariate distributions of RVs or the functional applied to the sum. We apply this approach to the log-normal distribution to compute the left tail and cumulative distribution of the ratio of independent RVs. For each case, we numerically demonstrate that the proposed state-dependent IS algorithm compares favorably to most well-known estimators dealing with similar problems.
更多查看译文
关键词
Monte Carlo,Rare event,Importance sampling,Stochastic optimal control
AI 理解论文
溯源树
样例
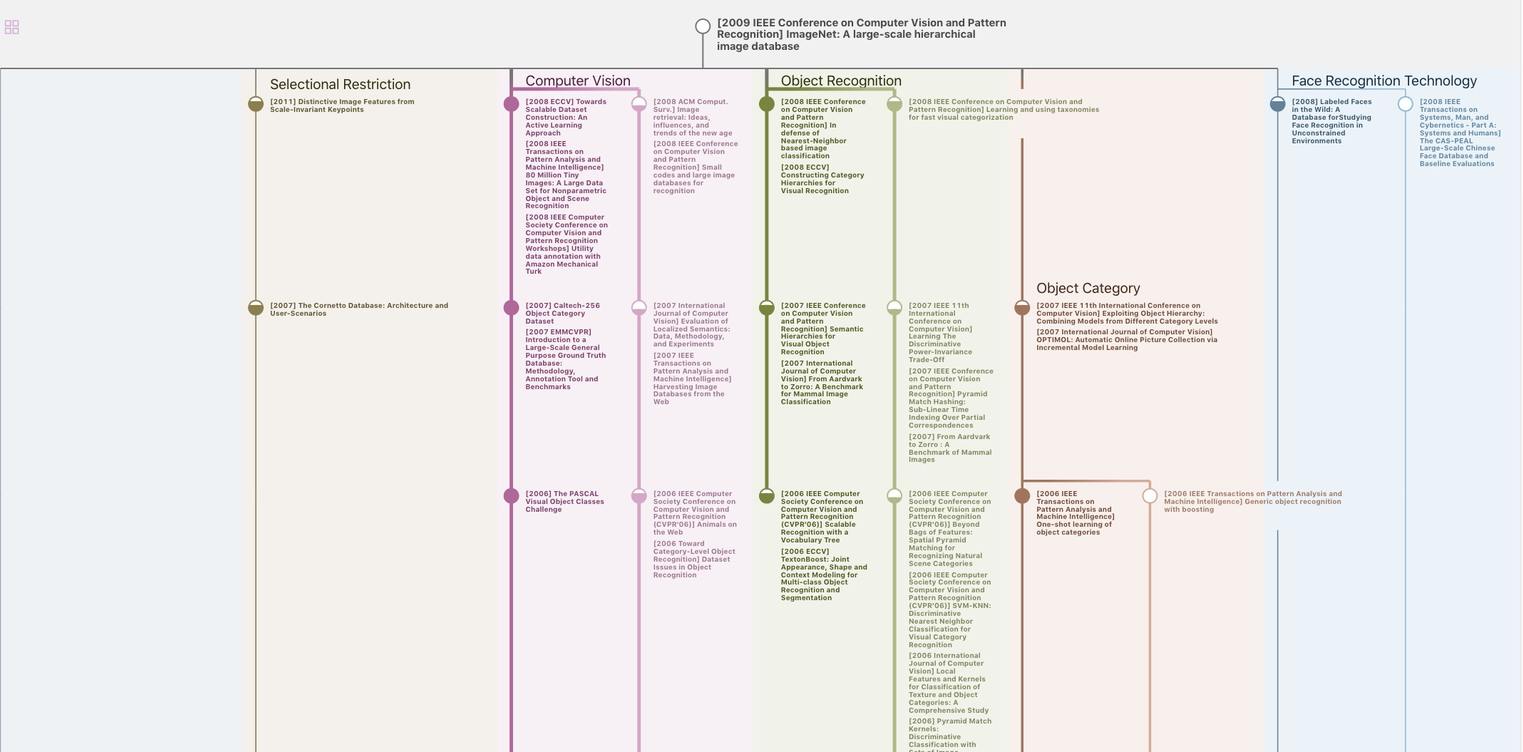
生成溯源树,研究论文发展脉络
Chat Paper
正在生成论文摘要