LightSleepNet: Design of a Personalized Portable Sleep Staging System Based on Single-Channel EEG
IEEE transactions on circuits and systems II, Express briefs(2022)
Abstract
This brief proposed LightSleepNet - a light-weight, 1-d Convolutional Neural Network (CNN) based personalized architecture for real-time sleep staging, which can be implemented on various mobile platforms with limited hardware resources. The proposed architecture only requires an input of 30s single-channel EEG signal for the classification. Two residual blocks consisting of group 1-d convolution are used instead of the traditional convolution layers to remove the redundancy in the CNN. Channel shuffles are inserted into each convolution layer to improve the accuracy. In order to avoid over-fitting to the training set, a Global Average Pooling (GAP) layer is used to replace the fully connected layer, which further reduces the total number of the model parameters significantly. A personalized algorithm combining Adaptive Batch Normalization (AdaBN) and gradient re-weighting is proposed for unsupervised domain adaptation. A higher priority is given to examples that are easy to transfer to the new subject, and the algorithm could be personalized for new subjects without re-training. Experimental results show a state-of-the-art overall accuracy of 83.8% with only 45.76 Million Floating-point Operations per Second (MFLOPs) computation and 43.08 K parameters.
MoreTranslated text
Key words
Sleep,Training,Convolution,Electroencephalography,Real-time systems,Neurons,Feature extraction,Sleep staging,light-weight architecture,channel shuffle,CNN,personalized healthy equipment
AI Read Science
Must-Reading Tree
Example
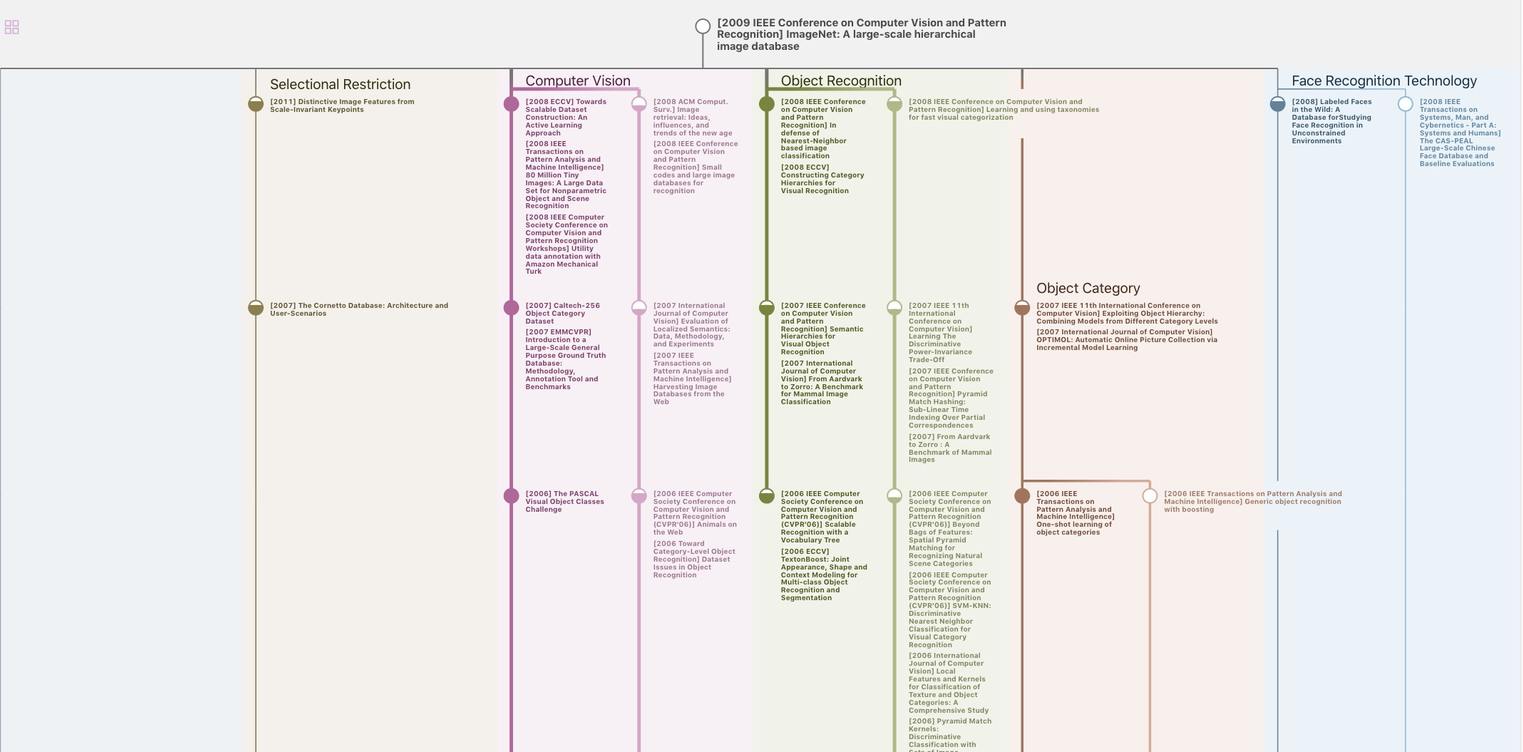
Generate MRT to find the research sequence of this paper
Chat Paper
Summary is being generated by the instructions you defined