A Deeper Understanding of State-Based Critics in Multi-Agent Reinforcement Learning.
AAAI Conference on Artificial Intelligence(2022)
摘要
Centralized Training for Decentralized Execution, where training is done in a centralized offline fashion, has become a popular solution paradigm in Multi-Agent Reinforcement Learning.Many such methods take the form of actor-critic with state-based critics, since centralized training allows access to the true system state, which can be useful during training despite not being available at execution time.State-based critics have become a common empirical choice, albeit one which has had limited theoretical justification or analysis.In this paper, we show that state-based critics can introduce bias in the policy gradient estimates, potentially undermining the asymptotic guarantees of the algorithm.We also show that, even if the state-based critics do not introduce any bias, they can still result in a larger gradient variance, contrary to the common intuition.Finally, we show the effects of the theories in practice by comparing different forms of centralized critics on a wide range of common benchmarks, and detail how various environmental properties are related to the effectiveness of different types of critics.
更多查看译文
关键词
Multiagent Systems (MAS)
AI 理解论文
溯源树
样例
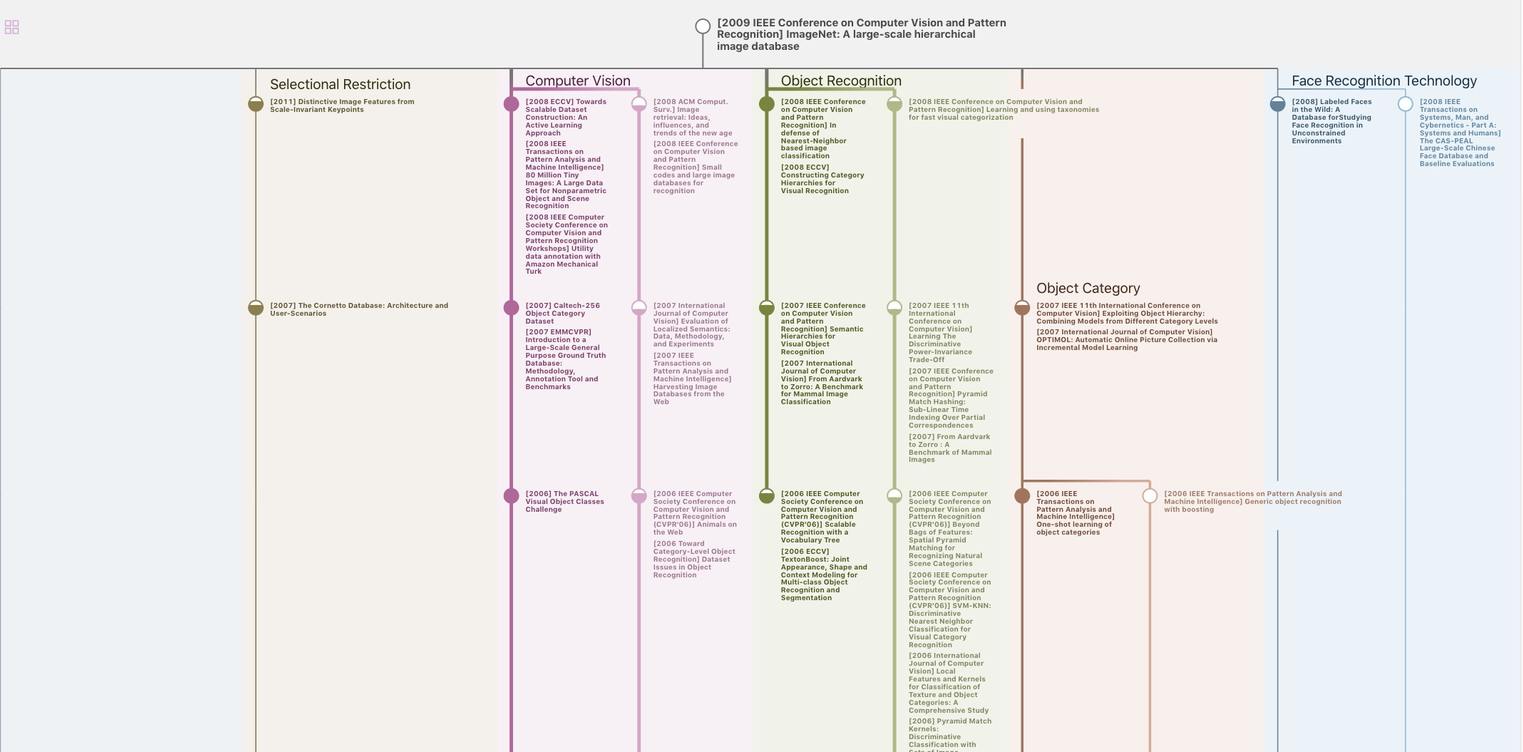
生成溯源树,研究论文发展脉络
Chat Paper
正在生成论文摘要