AutoDES: AutoML Pipeline Generation of Classification with Dynamic Ensemble Strategy Selection
arxiv(2022)
摘要
Automating machine learning has achieved remarkable technological developments in recent years, and building an automated machine learning pipeline is now an essential task. The model ensemble is the technique of combining multiple models to get a better and more robust model. However, existing automated machine learning tends to be simplistic in handling the model ensemble, where the ensemble strategy is fixed, such as stacked generalization. There have been many techniques on different ensemble methods, especially ensemble selection, and the fixed ensemble strategy limits the upper limit of the model's performance. In this article, we present a novel framework for automated machine learning. Our framework incorporates advances in dynamic ensemble selection, and to our best knowledge, our approach is the first in the field of AutoML to search and optimize ensemble strategies. In the comparison experiments, our method outperforms the state-of-the-art automated machine learning frameworks with the same CPU time in 42 classification datasets from the OpenML platform. Ablation experiments on our framework validate the effectiveness of our proposed method.
更多查看译文
关键词
automl pipeline generation,ensemble strategy selection,classification
AI 理解论文
溯源树
样例
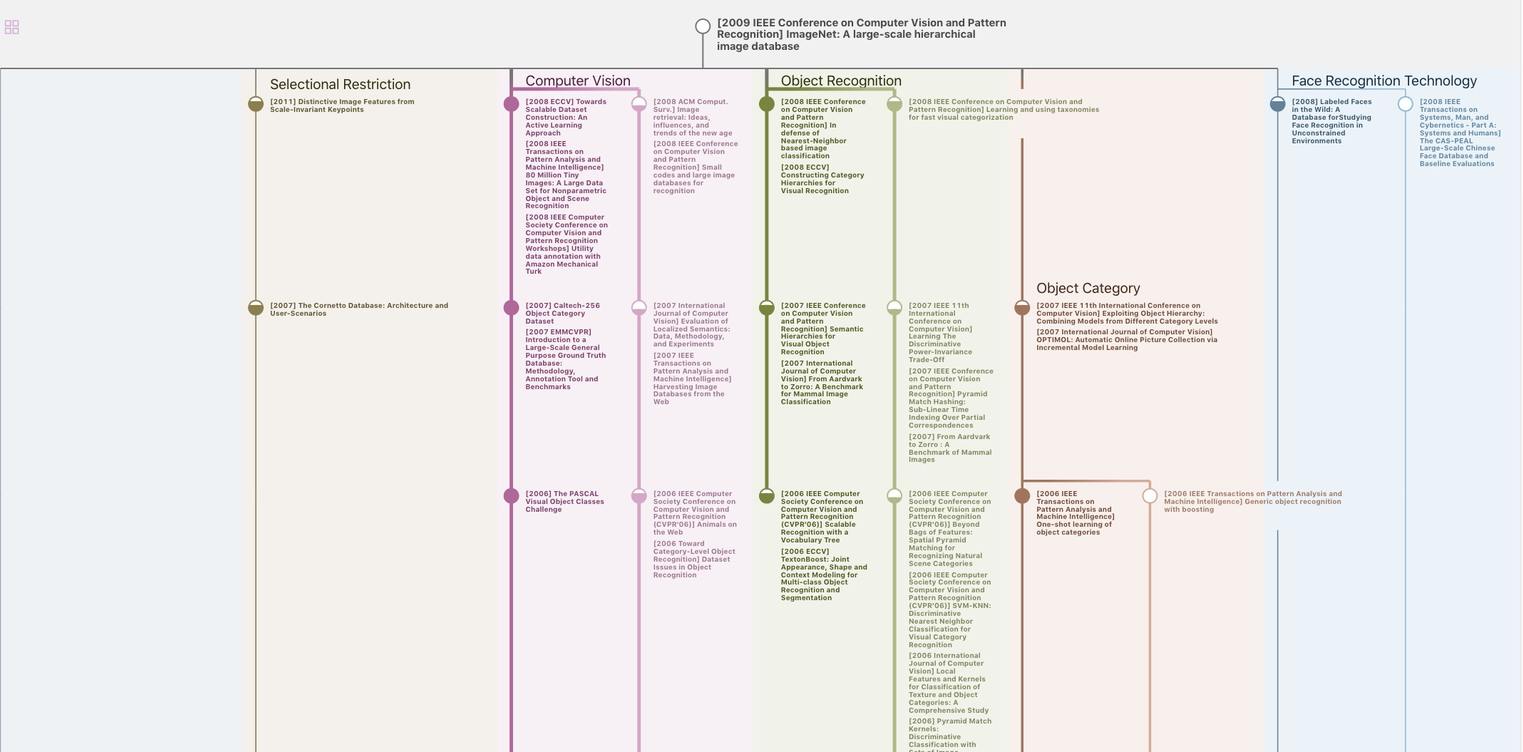
生成溯源树,研究论文发展脉络
Chat Paper
正在生成论文摘要