Machine Learning Analysis of Cocaine Addiction Informed by DAT, SERT, and NET-Based Interactome Networks
JOURNAL OF CHEMICAL THEORY AND COMPUTATION(2022)
Abstract
Cocaine addiction is a psychosocial disorder induced by the chronicuse of cocaine and causes a large number of deaths around the world. Despitedecades of effort, no drugs have been approved by the Food and DrugAdministration (FDA) for the treatment of cocaine dependence. Cocainedependence is neurological and involves many interacting proteins in theinteractome. Among them, the dopamine (DAT), serotonin (SERT), andnorepinephrine (NET) transporters are three major targets. Each of these targetshas a large protein-protein interaction (PPI) network, which must be considered inthe anticocaine addiction drug discovery. This work presents DAT, SERT, and NETinteractome network-informed machine learning/deep learning (ML/DL) studies ofcocaine addiction. We collected and analyzed 61 protein targets out of 460 proteinsin the DAT, SERT, and NET PPI networks that have sufficiently large existinginhibitor datasets. Utilizing autoencoder (AE) and other ML/DL algorithms,including gradient boosting decision tree (GBDT) and multitask deep neuralnetwork (MT-DNN), we built predictive models for these targets with 115 407 inhibitors to predict drug repurposing potential andpossible side effects. We further screened their absorption, distribution, metabolism, and excretion, and toxicity (ADMET)properties to search for leads having potential for developing treatments for cocaine addiction. Our approach offers a new systematicprotocol for artificial intelligence (AI)-based anticocaine addiction lead discovery.
MoreTranslated text
Key words
cocaine addiction,machine learning,networks,sert,net-based
AI Read Science
Must-Reading Tree
Example
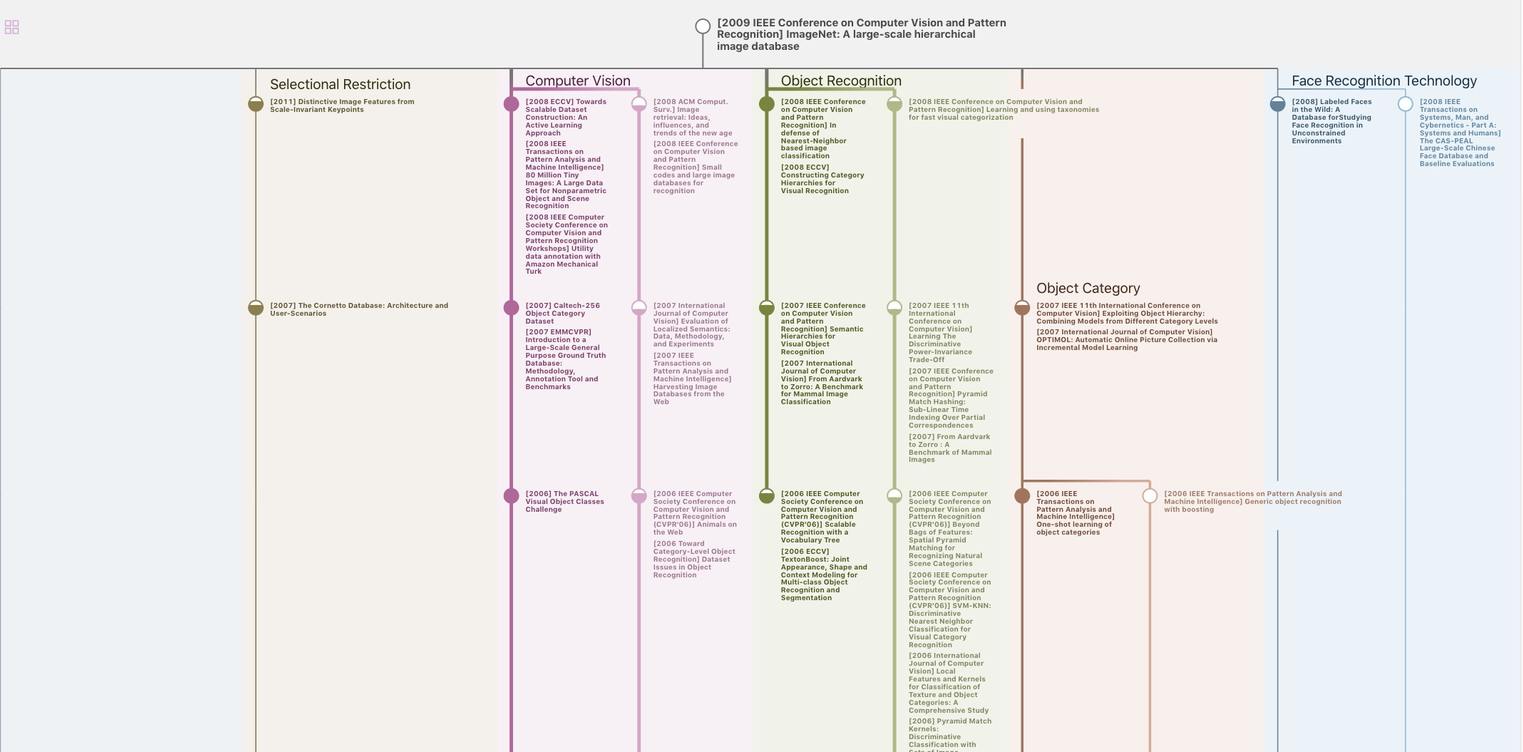
Generate MRT to find the research sequence of this paper
Chat Paper
Summary is being generated by the instructions you defined