Statistical scalability and approximate inference in distributed computing environments
arxiv(2021)
摘要
Harnessing distributed computing environments to build scalable inference algorithms for very large data sets is a core challenge across the broad mathematical sciences. Here we provide a theoretical framework to do so along with fully implemented examples of scalable algorithms with performance guarantees. We begin by formalizing the class of statistics which admit straightforward calculation in such environments through independent parallelization. We then show how to use such statistics to approximate arbitrary functional operators, thereby providing practitioners with a generic approximate inference procedure that does not require data to reside entirely in memory. We characterize the $L^2$ approximation properties of our approach, and then use it to treat two canonical examples that arise in large-scale statistical analyses: sample quantile calculation and local polynomial regression. A variety of avenues and extensions remain open for future work.
更多查看译文
AI 理解论文
溯源树
样例
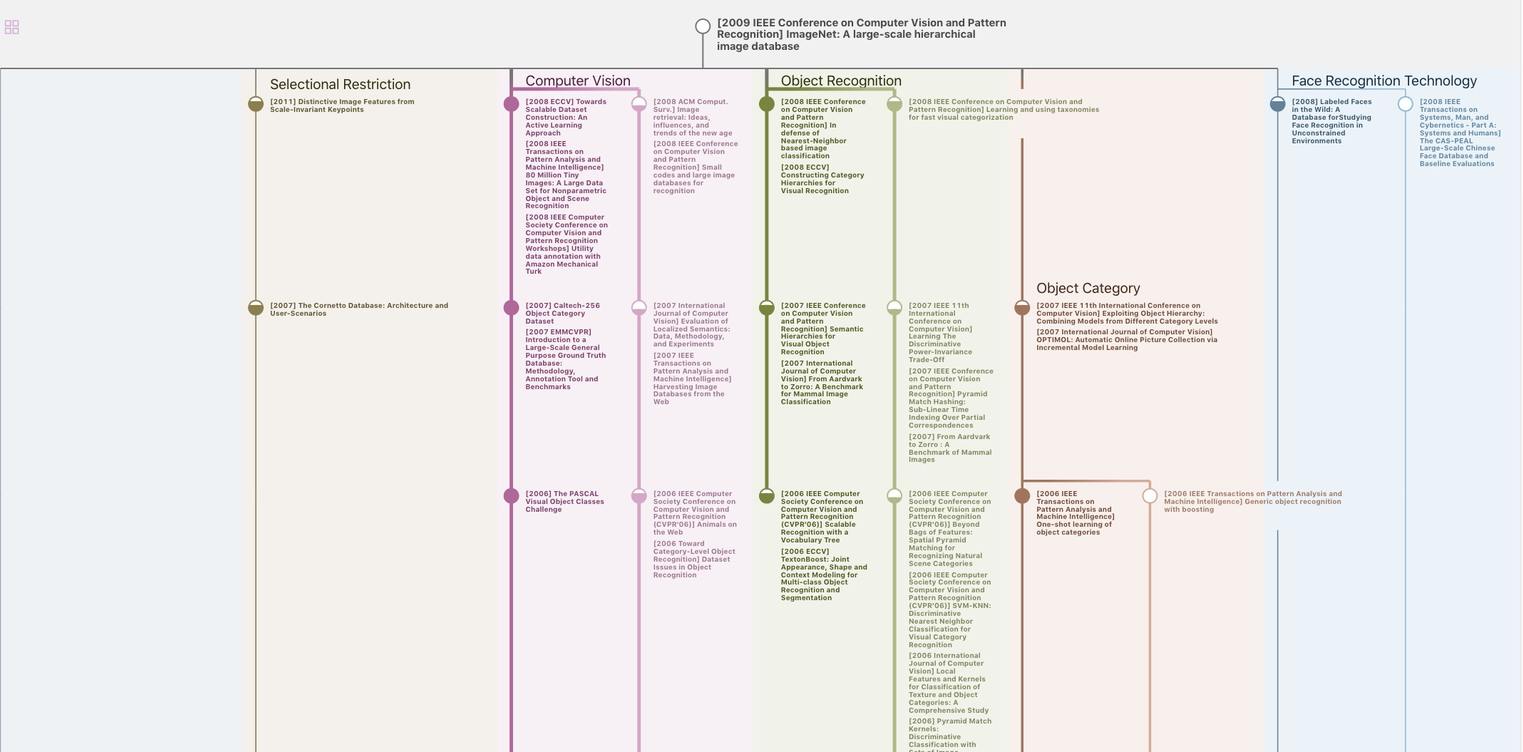
生成溯源树,研究论文发展脉络
Chat Paper
正在生成论文摘要