A causal inference framework for spatial confounding
arXiv (Cornell University)(2021)
摘要
Recently, addressing spatial confounding has become a major topic in spatial
statistics. However, the literature has provided conflicting definitions, and
many proposed definitions do not address the issue of confounding as it is
understood in causal inference. We define spatial confounding as the existence
of an unmeasured causal confounder with a spatial structure. We present a
causal inference framework for nonparametric identification of the causal
effect of a continuous exposure on an outcome in the presence of spatial
confounding. We propose double machine learning (DML), a procedure in which
flexible models are used to regress both the exposure and outcome variables on
confounders to arrive at a causal estimator with favorable robustness
properties and convergence rates, and we prove that this approach is consistent
and asymptotically normal under spatial dependence. As far as we are aware,
this is the first approach to spatial confounding that does not rely on
restrictive parametric assumptions (such as linearity, effect homogeneity, or
Gaussianity) for both identification and estimation. We demonstrate the
advantages of the DML approach analytically and in simulations. We apply our
methods and reasoning to a study of the effect of fine particulate matter
exposure during pregnancy on birthweight in California.
更多查看译文
关键词
causal inference framework,spatial
AI 理解论文
溯源树
样例
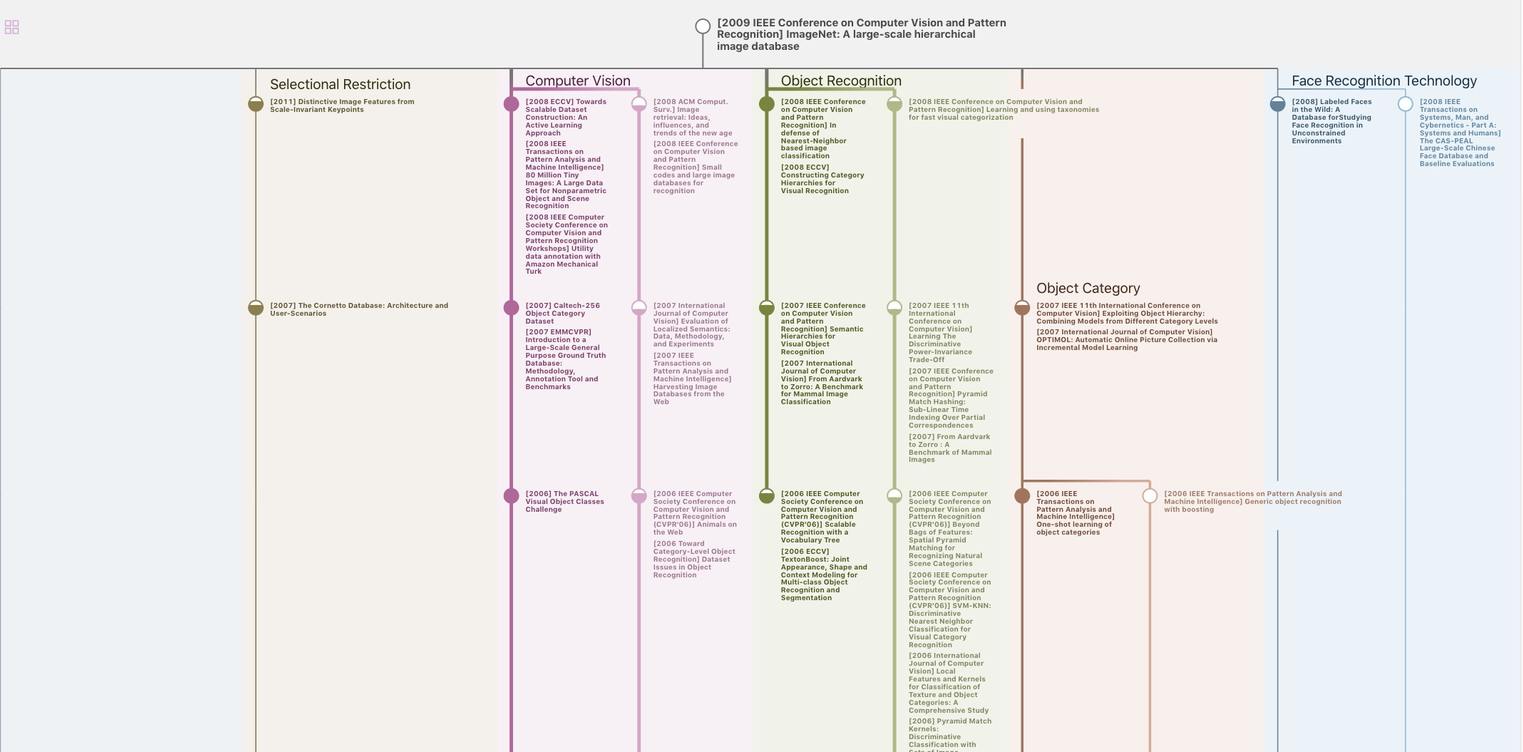
生成溯源树,研究论文发展脉络
Chat Paper
正在生成论文摘要