On the Instability of Relative Pose Estimation and RANSAC's Role
IEEE Conference on Computer Vision and Pattern Recognition(2022)
摘要
Relative pose estimation using the 5-point or 7-point Random Sample Consensus (RANSAC) algorithms can fail even when no outliers are present and there are enough inliers to support a hypothesis. These cases arise due to numerical instability of the 5- and 7-point minimal problems. This paper characterizes these instabilities, both in terms of minimal world scene configurations that lead to infinite condition number in epipolar estimation, and also in terms of the related minimal image feature pair correspondence configurations. The instability is studied in the context of a novel framework for analyzing the conditioning of minimal problems in multiview geometry, based on Riemannian manifolds. Experiments with synthetic and real-world data reveal that RANSAC does not only serve to filter out outliers, but RANSAC also selects for well-conditioned image data, sufficiently separated from the ill-posed locus that our theory predicts. These findings suggest that, in future work, one could try to accelerate and increase the success of RANSAC by testing only well-conditioned image data.
更多查看译文
关键词
Computer vision theory, 3D from multi-view and sensors
AI 理解论文
溯源树
样例
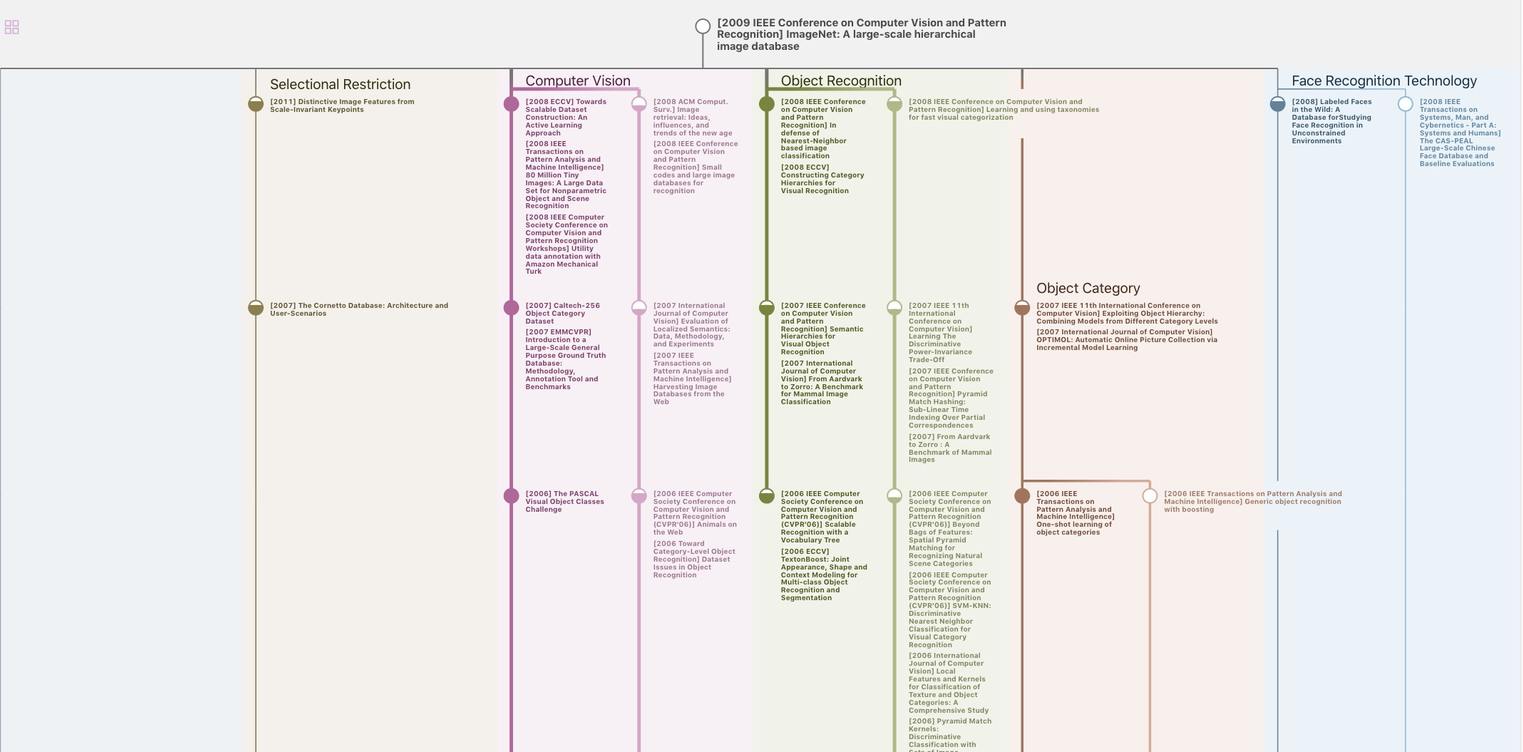
生成溯源树,研究论文发展脉络
Chat Paper
正在生成论文摘要