Variational Learning for the Inverted Beta-Liouville Mixture Model and Its Application to Text Categorization
INTERNATIONAL JOURNAL OF INTERACTIVE MULTIMEDIA AND ARTIFICIAL INTELLIGENCE(2022)
摘要
The finite invert Beta-Liouville mixture model (IBLMM) has recently gained some attention due to its positive data modeling capability. Under the conventional variational inference (VI) framework, the analytically tractable solution to the optimization of the variational posterior distribution cannot be obtained, since the variational object function involves evaluation of intractable moments. With the recently proposed extended variational inference (EVI) framework, a new function is proposed to replace the original variational object function in order to avoid intractable moment computation, so that the analytically tractable solution of the IBLMM can be derived in an effective way. The good performance of the proposed approach is demonstrated by experiments with both synthesized data and a real-world application namely text categorization.
更多查看译文
关键词
Bayesian Inference, Extended Variational Inference, Inverted Beta-Liouville Distribution, Mixture Model, Text Categorization
AI 理解论文
溯源树
样例
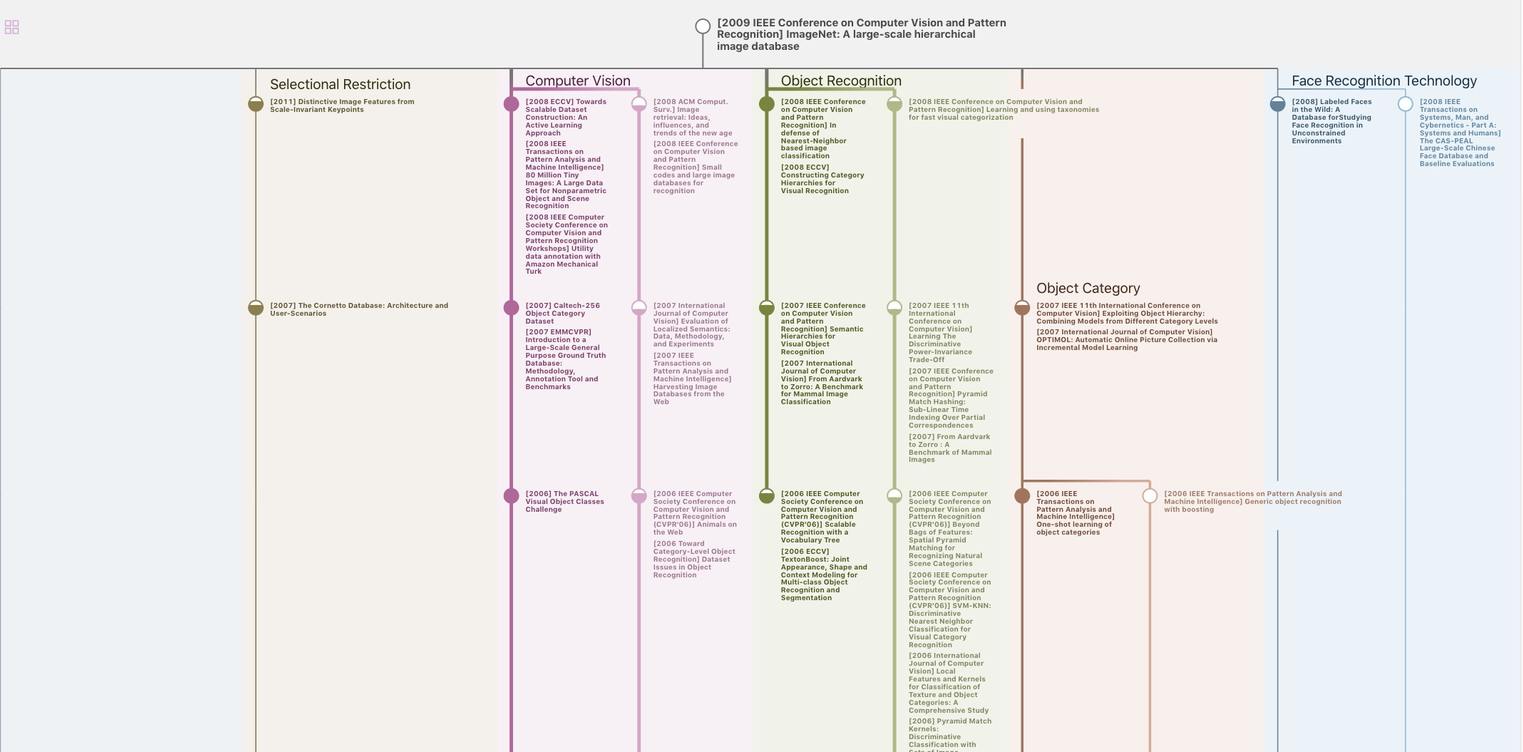
生成溯源树,研究论文发展脉络
Chat Paper
正在生成论文摘要