Machine learning phase transitions of the three-dimensional Ising universality class*
CHINESE PHYSICS C(2023)
摘要
Exploration of the QCD phase diagram and critical point is one of the main goals in current relativistic heavy-ion collisions. The QCD critical point is expected to belong to a three-dimensional (3D) Ising universality class. Machine learning techniques are found to be powerful in distinguishing different phases of matter and provide a new way to study the phase diagram. We investigate phase transitions in the 3D cubic Ising model using supervised learning methods. It is found that a 3D convolutional neural network can be trained to effectively predict physical quantities in different spin configurations. With a uniform neural network architecture, it can encode phases of matter and identify both second- and first-order phase transitions. The important features that discriminate different phases in the classification processes are investigated. These findings can help study and understand QCD phase transitions in relativistic heavy-ion collisions.
更多查看译文
关键词
machine learning,phase transition,QCD critical point,three-dimensional Ising universality class
AI 理解论文
溯源树
样例
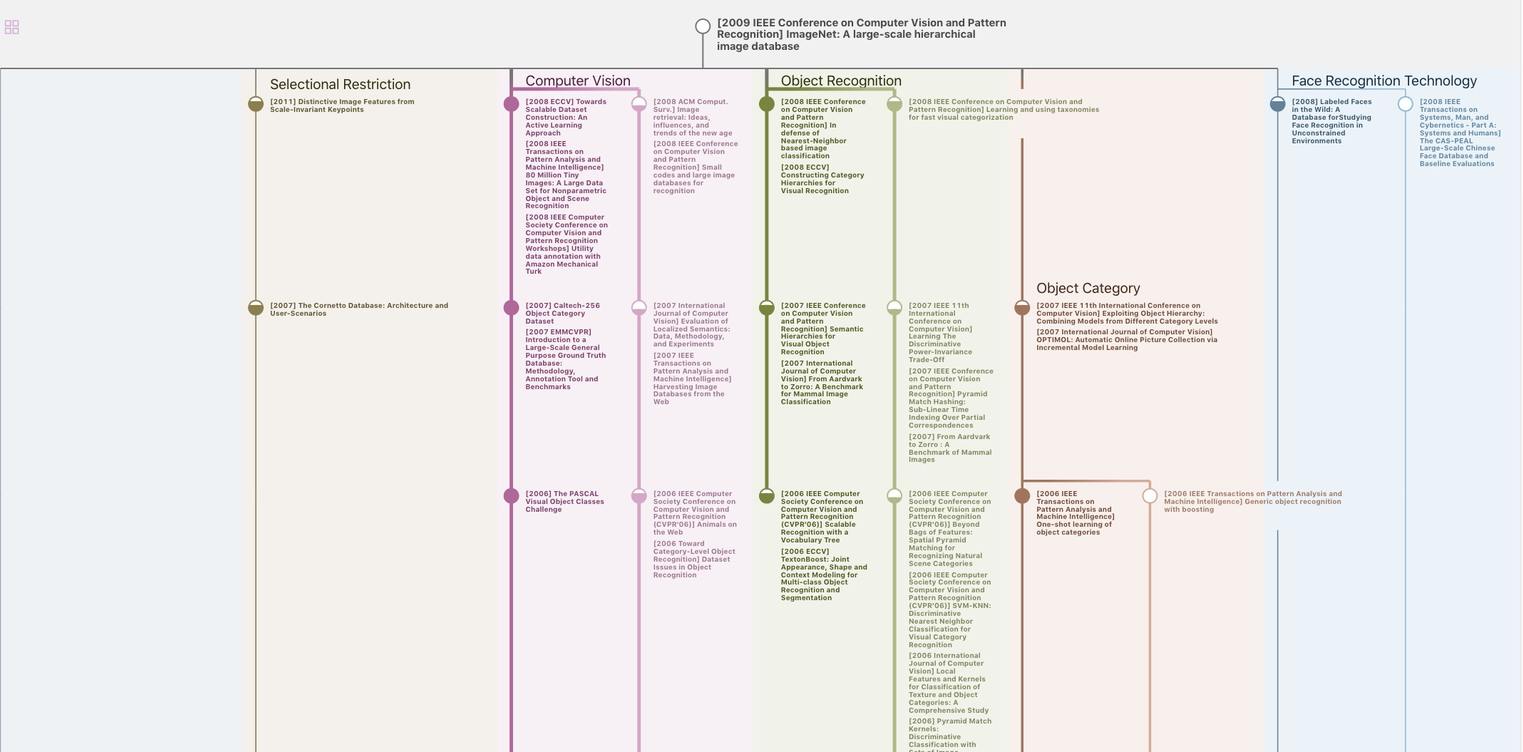
生成溯源树,研究论文发展脉络
Chat Paper
正在生成论文摘要