Transfer Learning of the ResNet-18 and DenseNet-121 Model Used to Diagnose Intracranial Hemorrhage in CT Scanning
CURRENT PHARMACEUTICAL DESIGN(2022)
摘要
Objective: The aim of the study was to verify the ability of the deep learning model to identify five subtypes and normal images in non-contrast enhancement CT of intracranial hemorrhage. Methods: A total of 351 patients (39 patients in the normal group, 312 patients in the intracranial hemorrhage group) who underwent intracranial hemorrhage noncontrast enhanced CT were selected, obtaining 2768 images in total (514 images for the normal group, 398 images for the epidural hemorrhage group, 501 images for the subdural hemorrhage group, 497 images for the intraventricular hemorrhage group, 415 images for the cerebral parenchymal hemorrhage group, and 443 images for the subarachnoid hemorrhage group). Based on the diag-nostic reports of two radiologists with more than 10 years of experience, the ResNet-18 and DenseNet-121 deep learning models were selected. Transfer learning was used. 80% of the data was used for training models, 10% was used for validating model performance against overfitting, and the last 10% was used for the final evaluation of the model. Assessment indicators included accuracy, sensitivity, specificity, and AUC values. Results: The overall accuracy of ResNet-18 and DenseNet-121 models was obtained as 89.64% and 82.5%, re-spectively. The sensitivity and specificity of identifying five subtypes and normal images were above 0.80. The sensitivity of the DenseNet-121 model to recognize intraventricular hemorrhage and cerebral parenchymal hem-orrhage was lower than 0.80, 0.73, and 0.76, respectively. The AUC values of the two deep learning models were found to be above 0.9. Conclusion: The deep learning model can accurately identify the five subtypes of intracranial hemorrhage and normal images, and it can be used as a new tool for clinical diagnosis in the future.
更多查看译文
关键词
Deep learning, transfer learning, intracranial hemorrhage, ResNet, DenseNet diagnosis, CT scanning
AI 理解论文
溯源树
样例
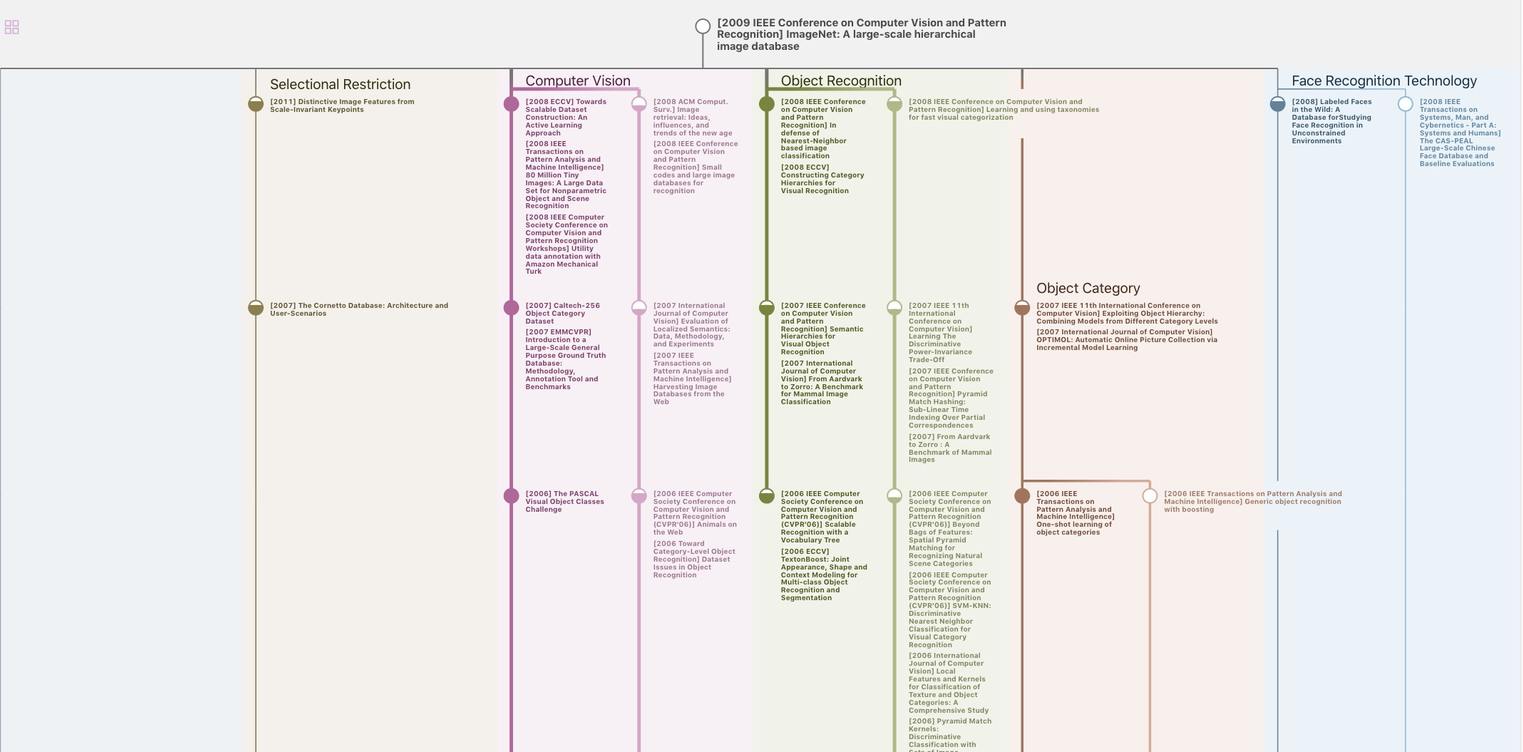
生成溯源树,研究论文发展脉络
Chat Paper
正在生成论文摘要