Utilizing DeepSqueak for automatic detection and classification of mammalian vocalizations: a case study on primate vocalizations
SCIENTIFIC REPORTS(2021)
摘要
Bioacoustic analyses of animal vocalizations are predominantly accomplished through manual scanning, a highly subjective and time-consuming process. Thus, validated automated analyses are needed that are usable for a variety of animal species and easy to handle by non-programing specialists. This study tested and validated whether DeepSqueak, a user-friendly software, developed for rodent ultrasonic vocalizations, can be generalized to automate the detection/segmentation, clustering and classification of high-frequency/ultrasonic vocalizations of a primate species. Our validation procedure showed that the trained detectors for vocalizations of the gray mouse lemur ( Microcebus murinus ) can deal with different call types, individual variation and different recording quality. Implementing additional filters drastically reduced noise signals (4225 events) and call fragments (637 events), resulting in 91% correct detections (N total = 3040). Additionally, the detectors could be used to detect the vocalizations of an evolutionary closely related species, the Goodman’s mouse lemur ( M. lehilahytsara ). An integrated supervised classifier classified 93% of the 2683 calls correctly to the respective call type, and the unsupervised clustering model grouped the calls into clusters matching the published human-made categories. This study shows that DeepSqueak can be successfully utilized to detect, cluster and classify high-frequency/ultrasonic vocalizations of other taxa than rodents, and suggests a validation procedure usable to evaluate further bioacoustics software.
更多查看译文
关键词
Animal behaviour,Behavioural ecology,Classification and taxonomy,Communication and replication,Machine learning,Science,Humanities and Social Sciences,multidisciplinary
AI 理解论文
溯源树
样例
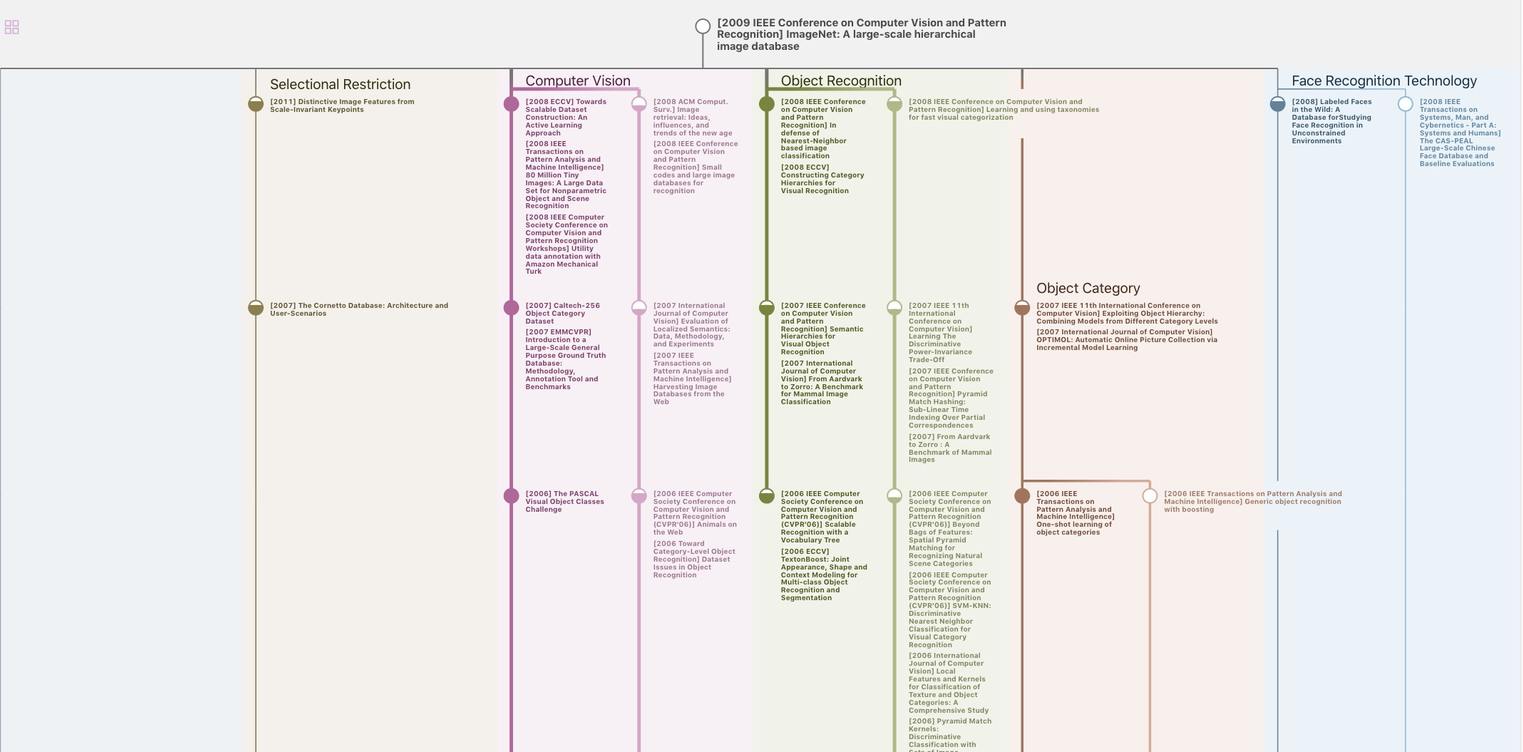
生成溯源树,研究论文发展脉络
Chat Paper
正在生成论文摘要