Deep Auto-Encoder and Deep Forest-Assisted Failure Prognosis for Dynamic Predictive Maintenance Scheduling
SENSORS(2021)
摘要
Prognostics and health management (PHM) with failure prognosis and maintenance decision-making as the core is an advanced technology to improve the safety, reliability, and operational economy of engineering systems. However, studies of failure prognosis and maintenance decision-making have been conducted separately over the past years. Key challenges remain open when the joint problem is considered. The aim of this paper is to develop an integrated strategy for dynamic predictive maintenance scheduling (DPMS) based on a deep auto-encoder and deep forest-assisted failure prognosis method. The proposed DPMS method involves a complete process from performing failure prognosis to making maintenance decisions. The first step is to extract representative features reflecting system degradation from raw sensor data by using a deep auto-encoder. Then, the features are fed into the deep forest to compute the failure probabilities in moving time horizons. Finally, an optimal maintenance-related decision is made through quickly evaluating the costs of different decisions with the failure probabilities. Verification was accomplished using NASA's open datasets of aircraft engines, and the experimental results show that the proposed DPMS method outperforms several state-of-the-art methods, which can benefit precise maintenance decisions and reduce maintenance costs.
更多查看译文
关键词
failure prognosis, maintenance decision-making, deep auto-encoder, deep forest, maintenance cost
AI 理解论文
溯源树
样例
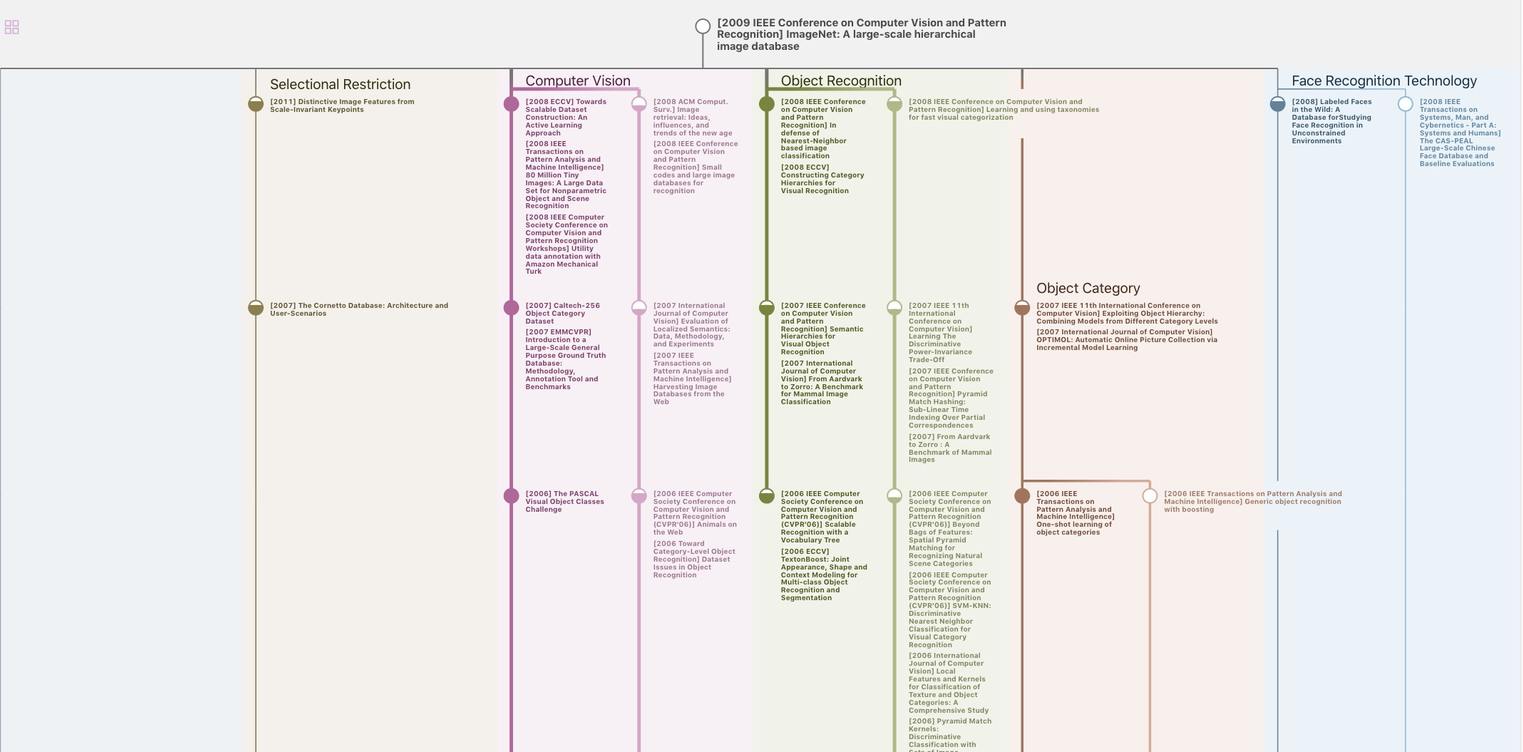
生成溯源树,研究论文发展脉络
Chat Paper
正在生成论文摘要