Daily production prediction for coalbed methane based on Bayesian temporal matrix factorization
Journal of Natural Gas Science and Engineering(2022)
Abstract
Effective and accurate daily production prediction of coalbed methane (CBM) is significant for enhancing CBM recovery and developing economic evaluations of CBM exploitation. As production time increases, CBM well data gradually show some characteristics of big data and is susceptible to incomplete data and noise data. Traditional geological modeling, however, requires a variety of input factors. In the present study, we apply a time series analysis method based on Bayesian temporal matrix factorization (BTMF) without the deletion of exceptional values to investigate the characteristics of CBM development. In addition, different from the widely used data preprocessing, we innovatively scale the multidimensional data to accomplish the analysis and prediction of different attributive data. By studying the temporal and attributive characteristics of the CBM production curve, the model is applied to a CBM block in China to impute the production data and perform a multi-step rolling prediction of daily gas production.
MoreTranslated text
Key words
Bayesian temporal matrix factorization,Time series analysis,Coalbed methane,Prediction of daily production
AI Read Science
Must-Reading Tree
Example
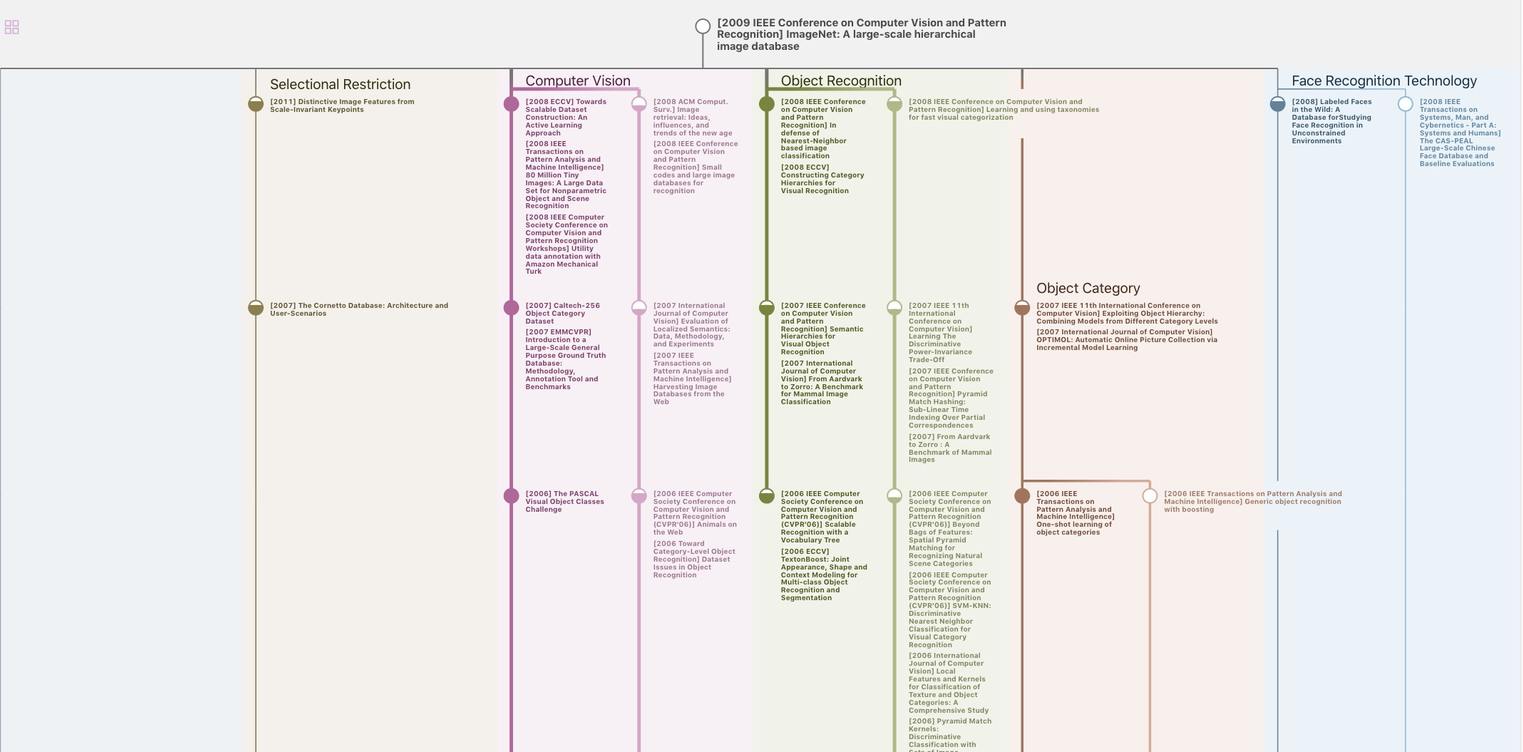
Generate MRT to find the research sequence of this paper
Chat Paper
Summary is being generated by the instructions you defined