Optimizing Requests for Support in Context-Restricted Autonomy
2021 IEEE/RSJ INTERNATIONAL CONFERENCE ON INTELLIGENT ROBOTS AND SYSTEMS (IROS)(2021)
摘要
Adjustable Autonomy is gaining interest as it alleviates robot management costs, which often restrain non-routine applications. Whereas it seems straightforward to account for the availability of helpers when making plans that involve being granted for support in the future, no existing research covers this issue. As a solution, we formalize the first human-centric model that accounts for operator support dynamics when generating adjustable-autonomy plans. We formalize Restricted Autonomy Levels (RAL) within a Markov-based framework for representing when and what level of support the robot should ask for. This model is combined with a formalization of usual aspects of man-machine collaboration: operator availability, risk of denial and withdrawal, effect of teleoperation, risks and consequences for violating RAL restrictions and backup procedures, should violations occur. We empirically demonstrate, through a detailed example and the deployment on a professional-grade security robot, that the generated plans deeply combine the problem-solving activities of the robot with the management of requested human support, leading to improved performance and decreased operator effort. We also analyse the computational costs of computing policies that ensure a zero-chance of RAL violation.
更多查看译文
关键词
robot management costs,human-centric model,operator support dynamics,Markov-based framework,man-machine collaboration,RAL restrictions,professional-grade security robot,RAL violation,context-restricted autonomy,adjustable-autonomy plans,restricted autonomy levels
AI 理解论文
溯源树
样例
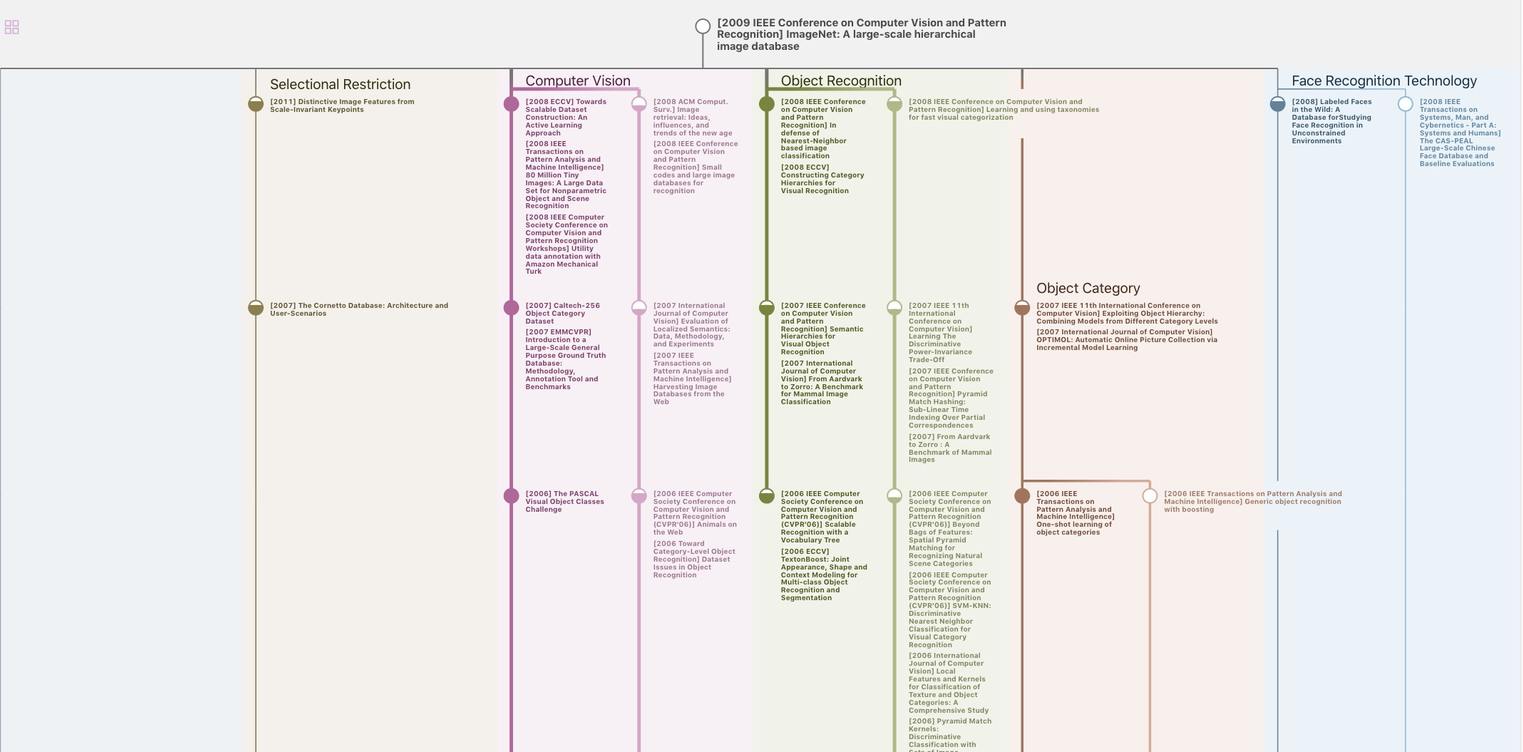
生成溯源树,研究论文发展脉络
Chat Paper
正在生成论文摘要