Map Compressibility Assessment for LiDAR Registration
2021 IEEE/RSJ INTERNATIONAL CONFERENCE ON INTELLIGENT ROBOTS AND SYSTEMS (IROS)(2021)
摘要
We aim to assess the performance of LiDAR-to-map registration on compressive maps. Modern autonomous vehicles utilize pre-built HD (High-Definition) maps to perform sensor-to-map registration, which recovers pose estimation failures and reduces drift in a large-scale environment. However, sensor-to-map registration is usually realized by registering the sensor to a dense 3D model, which occupies massive storage space in the HD map and requires much data processing overhead. Although smaller 3D models are preferable, the optimal compressive map format for preservation of the best registration performance remains unclear. In this paper, we propose a novel and challenging benchmark to evaluate existing LiDAR-to-map registration methods from three perspectives: map compressibility, robustness, and precision. We compared various map formats, including raw points, hierarchical GMMs, and feature points, and show their performance trade-offs between compressibility and robustness on real-world LiDAR datasets: KITTI Odometry Dataset and Argoverse Tracking Dataset. Our benchmark reveals that state-of-the-art deep feature point based methods outperform traditional methods significantly when the map size budget is high. However, when map size budget is low, deep methods are outperformed by the methods using simpler models in Argoverse Tracking Dataset due to poor spatial coverage. In addition, we observe that the recently published TEASER++ significantly outperforms RANSAC for the feature point methods. Our analysis provides a valuable reference for the community to design budgeted real-world systems and find potential research opportunities. We will release the benchmark for public use.
更多查看译文
关键词
map compressibility assessment,LiDAR registration,compressive maps,HD map,sensor-to-map registration,dense 3D model,smaller 3D models,optimal compressive map format,registration performance,LiDAR-to-map registration methods,map formats,performance trade-offs,real-world LiDAR datasets,Argoverse Tracking Dataset,state-of-the-art deep feature point based methods,map size budget,feature point methods
AI 理解论文
溯源树
样例
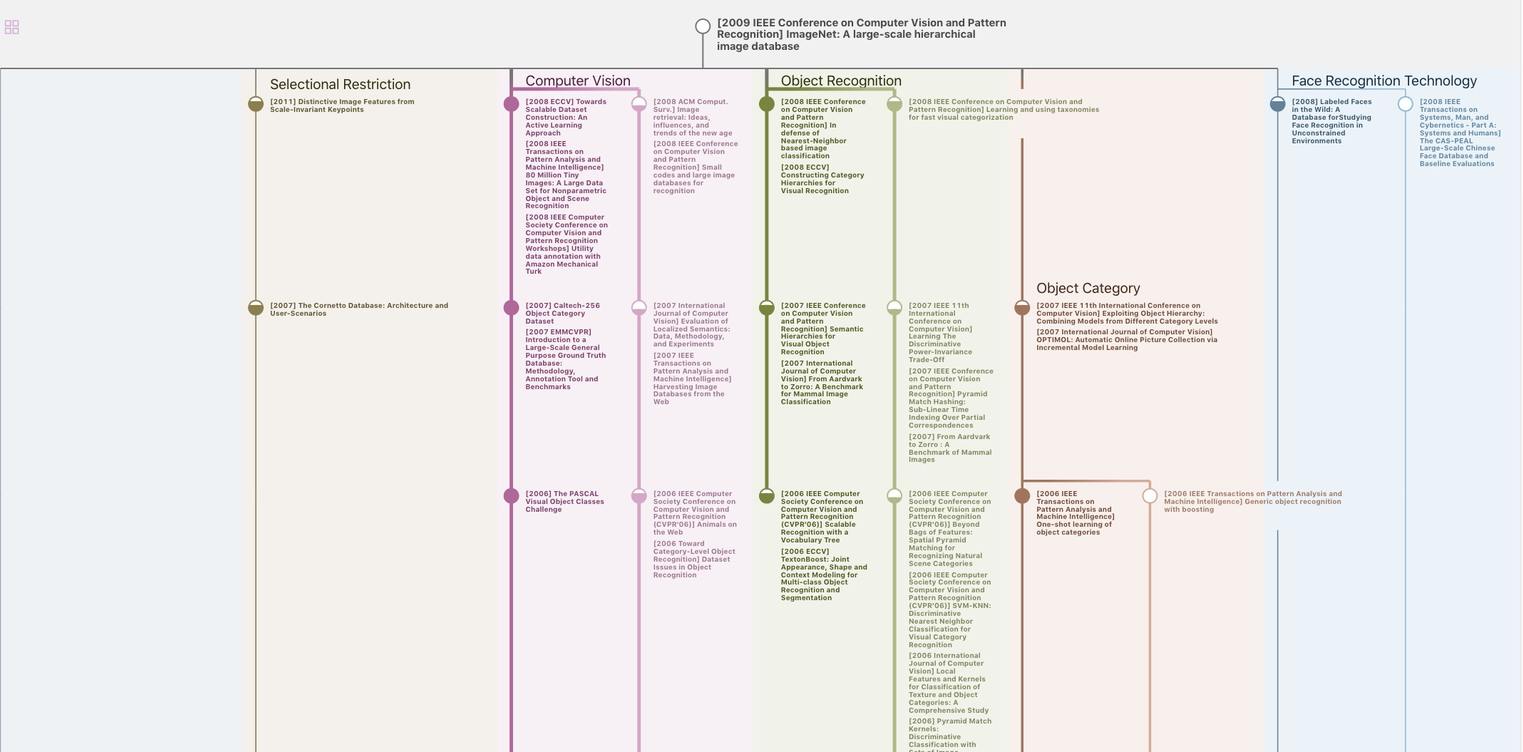
生成溯源树,研究论文发展脉络
Chat Paper
正在生成论文摘要