Pose Estimation from RGB Images of Highly Symmetric Objects using a Novel Multi-Pose Loss and Differential Rendering
2021 IEEE/RSJ INTERNATIONAL CONFERENCE ON INTELLIGENT ROBOTS AND SYSTEMS (IROS)(2021)
摘要
We propose a novel multi-pose loss function to train a neural network for 6D pose estimation, using synthetic data and evaluating it on real images. Our loss is inspired by the VSD (Visible Surface Discrepancy) metric and relies on a differentiable renderer and CAD models. This novel multi-pose approach produces multiple weighted pose estimates to avoid getting stuck in local minima. Our method resolves pose ambiguities without using predefined symmetries. It is trained only on synthetic data. We test on real-world RGB images from the T-LESS dataset, containing highly symmetric objects common in industrial settings. We show that our solution can be used to replace the codebook in a state-of-the-art approach. So far, the codebook approach has had the shortest inference time in the field. Our approach reduces inference time further while a) avoiding discretization, b) requiring a much smaller memory footprint and c) improving pose recall.(3)
更多查看译文
关键词
symmetric objects,differential rendering,rgb images,multi-pose
AI 理解论文
溯源树
样例
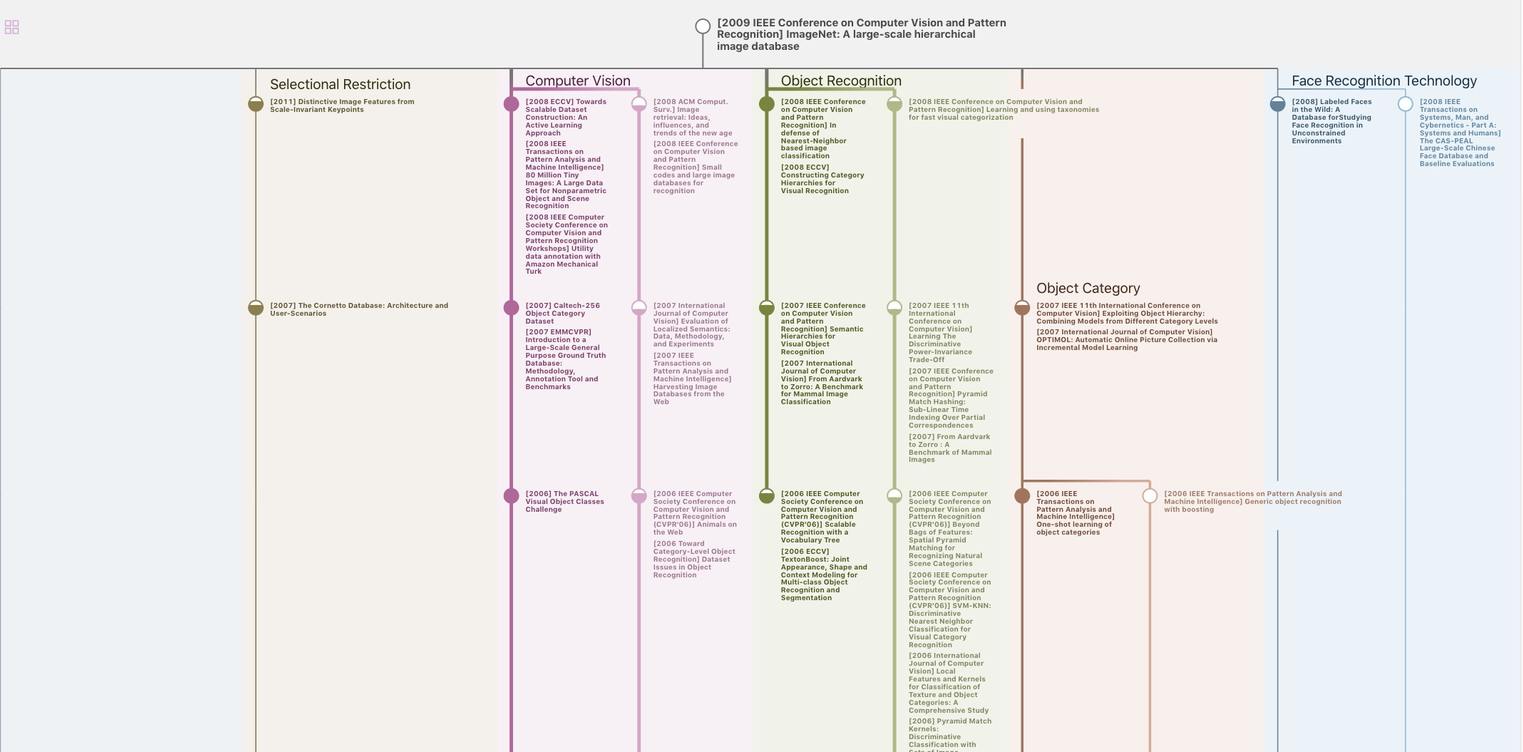
生成溯源树,研究论文发展脉络
Chat Paper
正在生成论文摘要