Scaling Average-Linkage via Sparse Cluster Embeddings.
ACML(2021)
摘要
Average-linkage is one of the most popular hierarchical clustering algorithms. It is well known that average-linkage does not scale to large data sets due to the slow asymptotic running time. The fastest known implementation has running time quadratic in the number of data points. This paper presents a technique that we call cluster embedding. The embedding maps each cluster into a point in slightly higher dimensions. The pairwise distances between the mapped points approximate the average distance between clusters. By utilizing this embedding we scale the task of finding close pairs of clusters, which is a key step in average-linkage clustering. We achieve an approximate, sub-quadratic time implementation of average-linkage. We show theoretically the algorithm proposed in this paper achieves a near-linear running time and scales to large data sets. Moreover, its scalability empirically dominates average-linkage and typically offers 3-10x speed-up on large data sets.
更多查看译文
AI 理解论文
溯源树
样例
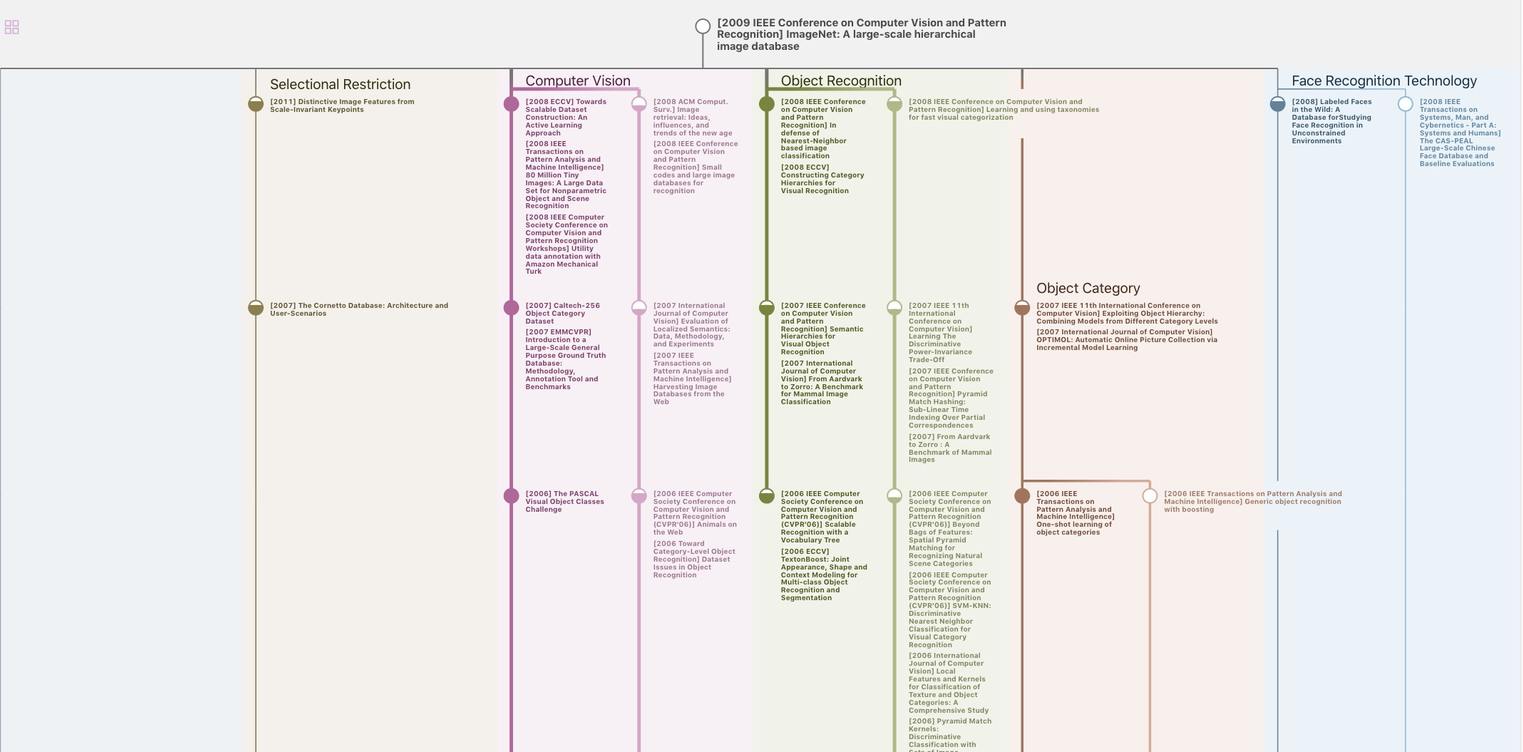
生成溯源树,研究论文发展脉络
Chat Paper
正在生成论文摘要