Category boundary re-decision by component labels to improve generation of class activation map
Neurocomputing(2022)
摘要
Class Activation Maps (CAMs) visualize the pixels within an image that contribute to classify the image to a certain category, and can be used to localize the object regions from images that benefit to many tasks such as image segmentation and object detection. However, the object regions highlighted by the existing methods are usually small and local. We believe such drawback lies in the image-level labels, because the the image-level label prefers the network to capture the common regions intra-class and the discriminative region inter-class. Due to the variations of objects intra-class and the common region widely shared inter class, the regions based on image-level labels are usually small and local. Based on such observation, we propose a new strategy to use a set of lower-level labels called component labels to replace the image-level labels. The advantage is that the component regions have small feature variation intra-class and are non-overlapping inter-class, which leads to better CAMs generation through their simple combination. Furthermore, since the component labels are also shared by unknown classes, the proposed CAMs generation method can be easily extended to unknown classes, which facilitate the improvement of tasks related to new class processing, such as few-shot segmentation and detection. Specifically, the component labels are set based on the WordNet hierarchy firstly, which can also provides the relationships of classes. Besides, graph convolution networks (GCNs) are used as the classifiers, which can exactly describe not only the component, but also their structural relationships. Based on the component features, a feature fusion module is also designed to merge local component features into the global feature. Better CAM is finally obtained. The experiment section shows the effectiveness of our component labels in terms of better subjective and objective results compared with the existing CAM generation methods. Furthermore, we also show good generalization of our component label on unknown classes.
更多查看译文
关键词
Component labels,Image classification,Class activation maps
AI 理解论文
溯源树
样例
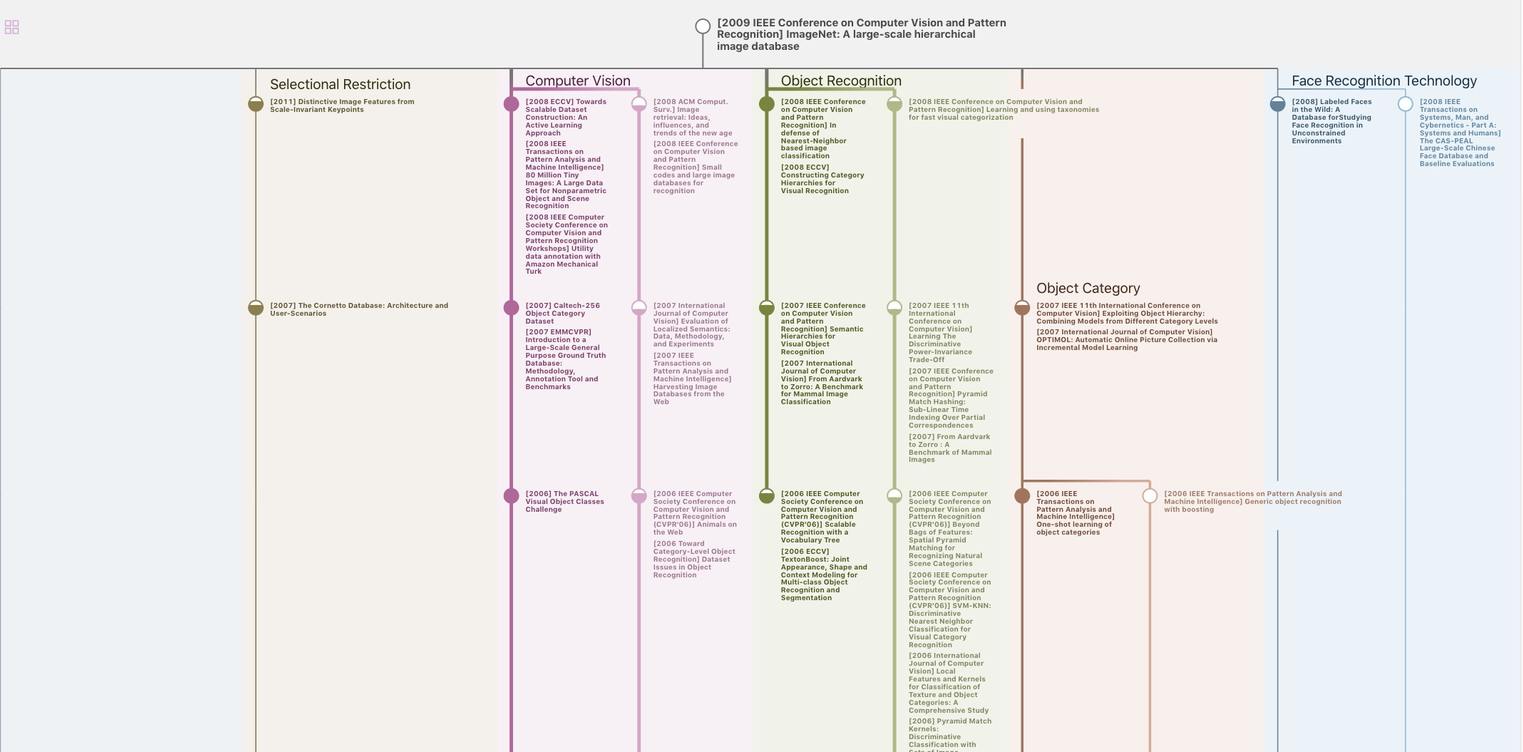
生成溯源树,研究论文发展脉络
Chat Paper
正在生成论文摘要