Improved YOLOv4 for dangerous goods detection in X-ray inspection combined with atrous convolution and transfer learning
Chinese Journal of Optics(2021)
Abstract
In response to the complex backgrounds of X-ray security images, serious overlap and occlusion phenomena, and the large differences in the placement and shape of dangerous goods, this paper improves the network structure of YOLOv4 for dangerous objects detection by combining atrous convolution with the Atrous Space Pyramid Pooling (ASPP) model to increase receptive field and aggregate multi-scale context information. Then, the K-means clustering method is used to generate an initial candidate frame that is more suitable for dangerous goods detection in X-ray inspection images. Cosine annealing is used to optimize the learning rate in model training to further accelerate model convergence and improve model detection accuracy. The experimental results show that the proposed ASPP-YOLOv4 in this paper can obtain an mAP of 85.23% on the SIXRay dataset. The model can effectively reduce the false detection rate of dangerous goods in X-ray security images and improve the detection ability of small targets.
MoreTranslated text
Key words
X-ray security images, YOLOv4, atrous convolution, spatial pyramid pooling, cosine annealing
AI Read Science
Must-Reading Tree
Example
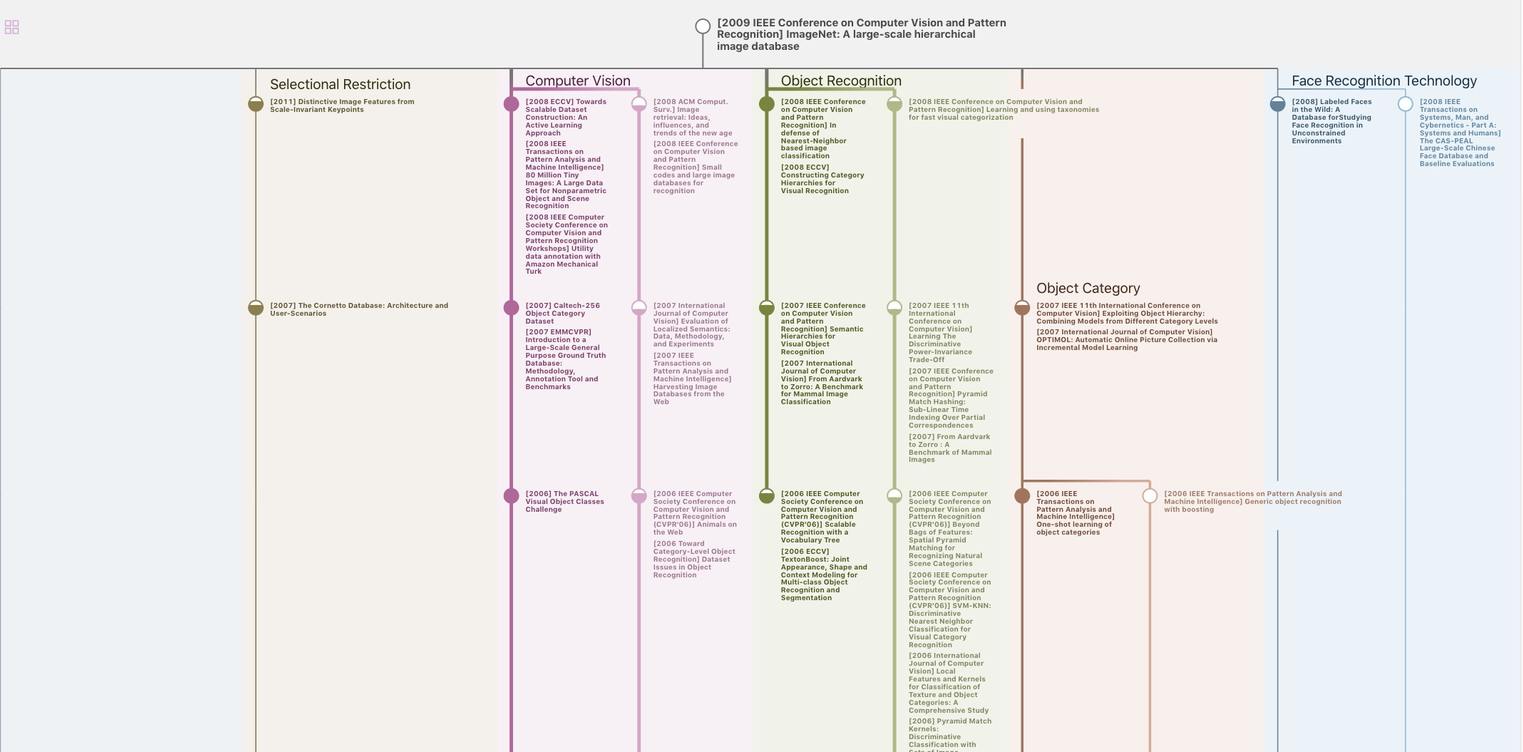
Generate MRT to find the research sequence of this paper
Chat Paper
Summary is being generated by the instructions you defined