Study on Soil Salinity Estimation Method of "Moisture Resistance" Using Visible-Near Infrared Spectroscopy in Coastal Region
Spectroscopy and Spectral Analysis(2021)
摘要
Rapid and accurate monitoring of soil salinity in the coastal regions are of great significance to the rational use and protection of land. Visible-near infrared spectroscopy has been widely used for the efficient estimation of soil properties. However, the interference of soil moisture on the spectrum decreases the estimation accuracy of traditional soil salinity estimation models. This paper aimed to explore the capacity of piecewise direct standardization (PDS) and orthogonal signal correction (OSC) in estimating the soil salt content under the condition of moisture interferedand establishing "moisture resistance" Vis-NIR models in the coastal saline regions. To this end, 114 soil samples were collected from the Yellow River Delta (0 similar to 20 cm) and divided the data into a modeling dataset (17 samples) and a validation dataset (127 samples). A control rewetting process obtained the soil spectral of the modeling dataset with 10 moisture content levels (0% , 1%, 5% , 10% , 15% , 20% , 25%, 30% , 40% and 50%). The soil spectral of the validation dataset was measured after a fully randomized trial, according to the generated 1 similar to 50 random integer. The modeling strategy combining PDS and OSC with partial least squares regression (PLSR) was proposed to build soil salinity estimation models. These models were validated and compared. Results showed that OSC was more effective than PDS in reducing modeling interference of moisture content in soil salinity estimation. Specifically, all of the PLSR models generated before and after spectral correction have achieved a certain level of success in soil salinity estimation (R-P(2) = 0. 79 similar to 0. 91 , RMSEP = 2. 6 similar to 3.98 g . kg(-1), RPD = 1. 98 similar to 2. 37). Compared with PLSR (R-P(2 )= 0. 86 , RMSEP = 3. 02 g . kg(-1), RPD = 2. 21) , OSC-PLSR could effectively improve the soil salinity estimation accuracy with R-P(2) = 0. 91, RMSEP = 2. 6 g . kg(-1), RPD = 2. 37, respectively. However, the PDS-PLSR model was not effective with R-P(2) = 0 . 79, RMSEP = 3. 98 g . kg(-1) and RPD=1. 98, respectively. The representation order of the model was OSC-PLSR>PLSR>PDS-PLSR. Furthermore, the analysis strategy of the variable importance in the projection (VIP) combined with Spearman correlation coefficients (r) were used for exploring the estimative mechanism. The important wavelengths (VIP > 1) of the models overlap with the sensitive wavelengths (vertical bar r vertical bar > 0. 4), which is of great significance for the soil salinity estimation. In comparison, OSC-PLSR accurately refines the wavelengths near 830, 1 940 and 2 050 nm that are important to the estimation model, while general PLSR and PDS-PLSR contain much redundant information. Overall, the OSC-PLSR model has strong moisture resistance in Vis-NIR soil salinity estimation, which provides feasibility for soil salinity study under soil moisture.
更多查看译文
关键词
Vis-NIR spectroscopy, Soil salinity, Moisture correction, Orthogonal signal correction, Coastal saline region
AI 理解论文
溯源树
样例
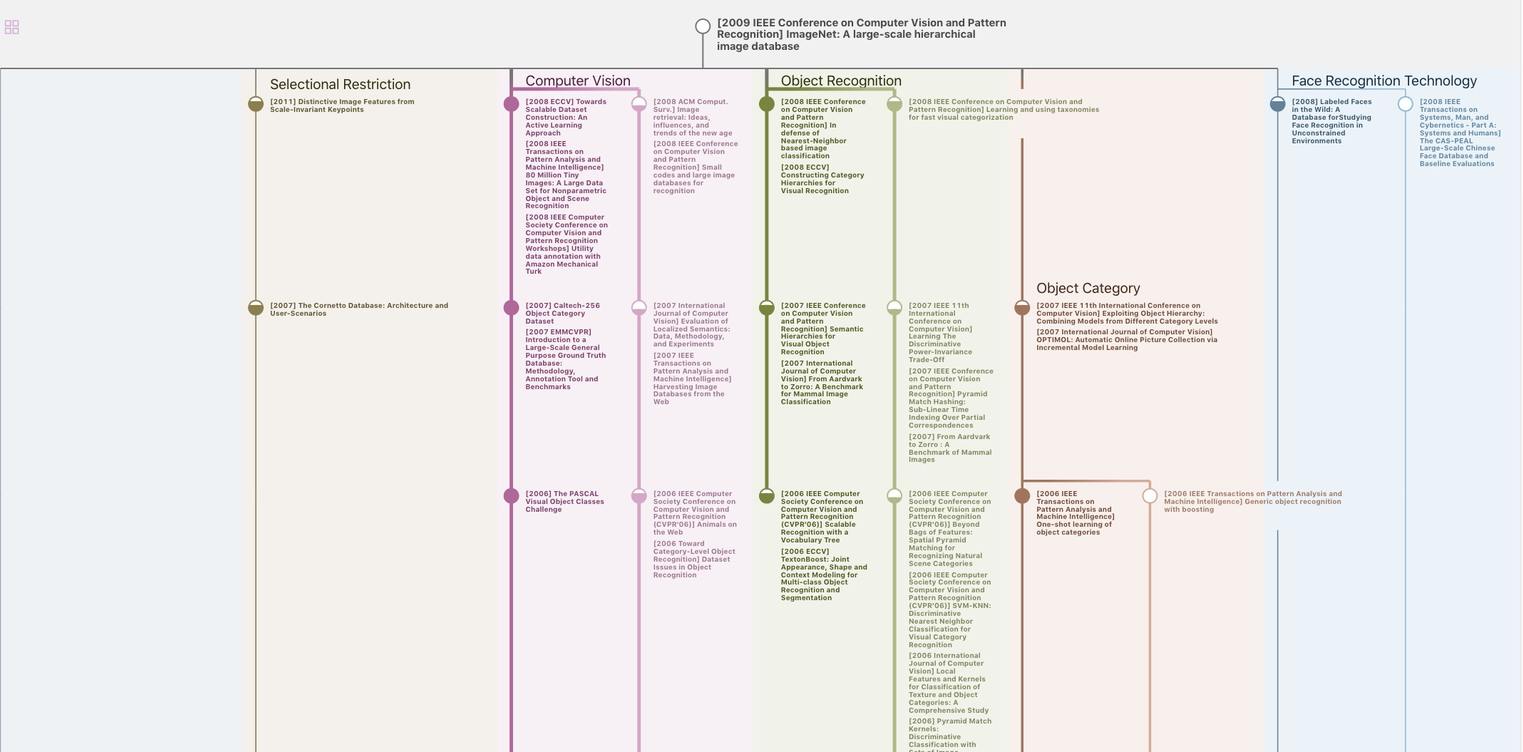
生成溯源树,研究论文发展脉络
Chat Paper
正在生成论文摘要