MDG: A Multi-Task Dynamic Graph Generation Framework for Multivariate Time Series Forecasting
IEEE TRANSACTIONS ON EMERGING TOPICS IN COMPUTATIONAL INTELLIGENCE(2024)
Abstract
For accurate forecasting of multivariate time series, it is essential to consider the relationship between temporal and spatial dimensions. Graph neural networks (GNNs) have gained popularity in recent years due to their ability to capture spatio-temporal correlations in a graph topology from multivariate time series. However, most existing GNN-based algorithms rely on predefined sensor distributions, limiting their applicability. Moreover, models that generate graphs without prior knowledge may overlook local conditions while constructing adjacency matrices. In this paper, we propose a multi-task learning approach that utilizes an AutoEncoder architecture for multivariate time series forecasting. Our method dynamically generates an adjacency matrix that is more focused on local changes in addition to a global graph structure that captures overall trends. The dynamic graph generation mechanism adaptively generates the adjacency matrix, and the two generated graphs are fused to avoid the issue of fixed matrices. We conducted experiments on publicly available datasets and introduced two new civil engineering datasets on tunnel boring machines to validate the effectiveness of our proposed model. Results demonstrate that our approach outperforms other baselines in terms of predictive performance.
MoreTranslated text
Key words
Multi-task learning,multivariate time series,time series forecasting,graph neural network
AI Read Science
Must-Reading Tree
Example
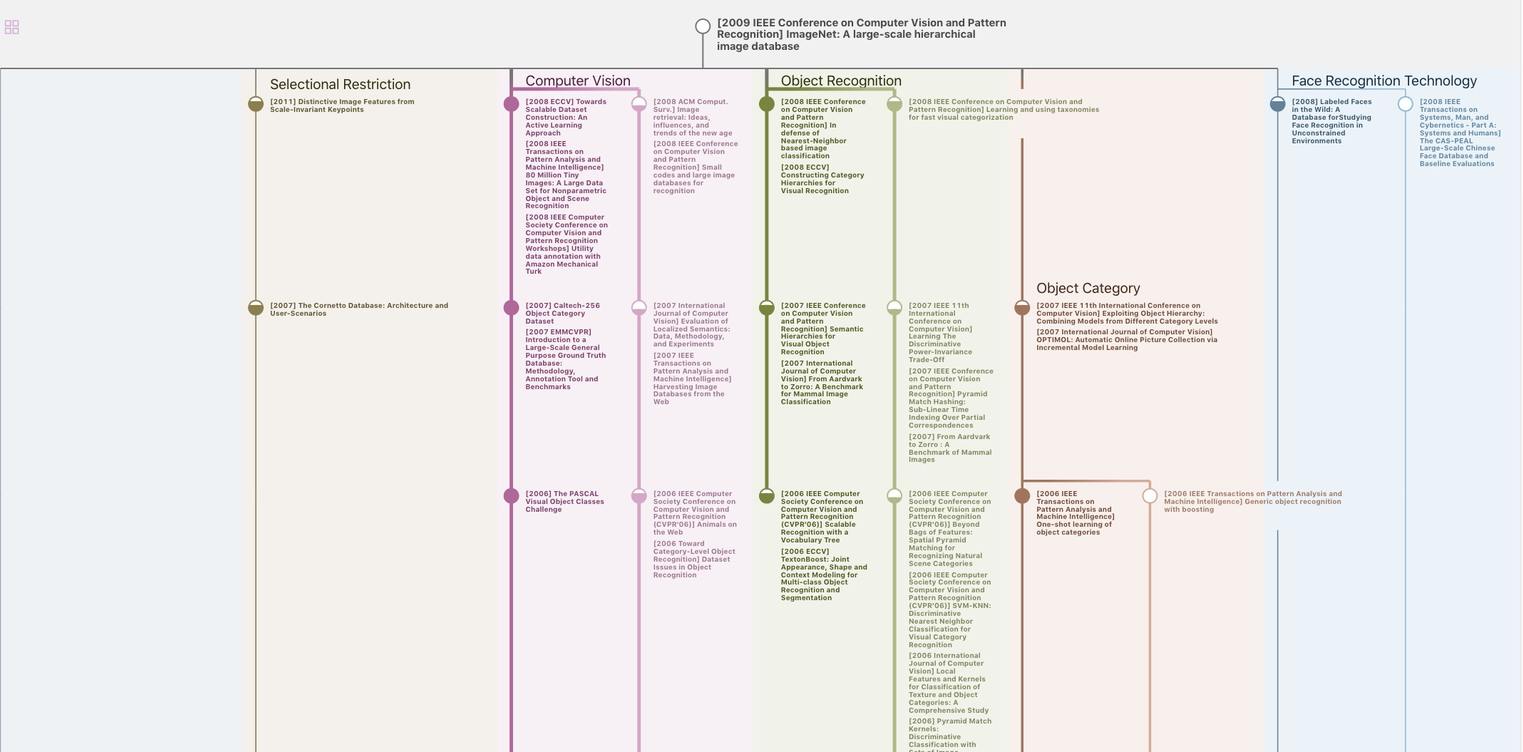
Generate MRT to find the research sequence of this paper
Chat Paper
Summary is being generated by the instructions you defined