A machine learning assisted optical multistage interconnection network: Performance analysis and hardware demonstration
ETRI JOURNAL(2023)
Abstract
Integration of the machine learning (ML) technique in all-optical networks can enhance the effectiveness of resource utilization, quality of service assurances, and scalability in optical networks. All-optical multistage interconnection networks (MINs) are implicitly designed to withstand the increasing high-volume traffic demands at data centers. However, the contention resolution mechanism in MINs becomes a bottleneck in handling such data traffic. In this paper, a select list of ML algorithms replaces the traditional electronic signal processing methods used to resolve contention in MIN. The suitability of these algorithms in improving the performance of the entire network is assessed in terms of injection rate, average latency, and latency distribution. Our findings showed that the ML module is recommended for improving the performance of the network. The improved performance and traffic grooming capabilities of the module are also validated by using a hardware testbed.
MoreTranslated text
Key words
contention resolution,hardware testbed,latency,machine learning
AI Read Science
Must-Reading Tree
Example
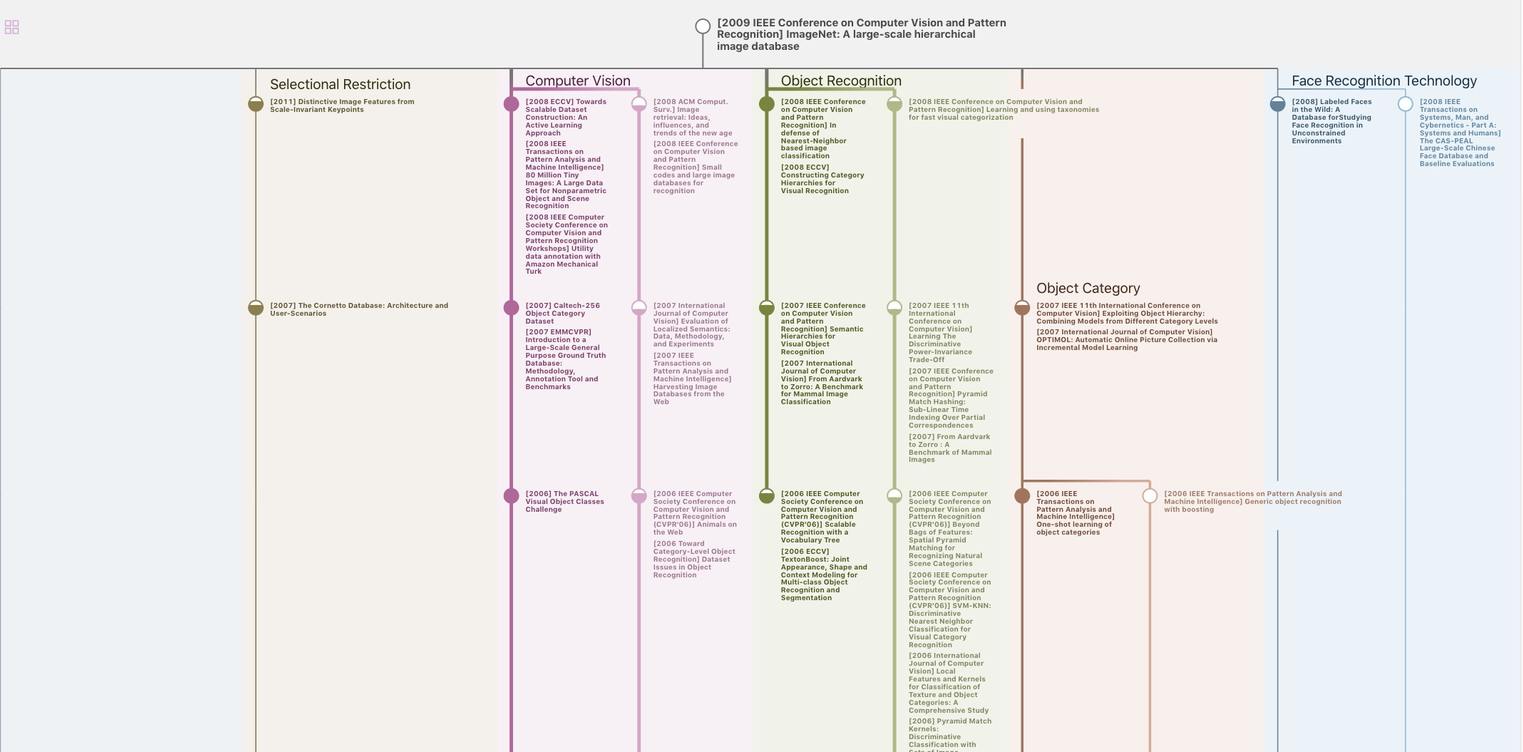
Generate MRT to find the research sequence of this paper
Chat Paper
Summary is being generated by the instructions you defined