Auto Machine Learning Approaches to Refine the Identification of High-Quality Clinical Research Articles from The Biomedical Literature: A Study Protocol (Preprint)
semanticscholar(2021)
摘要
UNSTRUCTURED
Due to the continued rapid growth in published biomedical literature, it is increasingly difficult to identify and retrieve high-quality evidence. Machine learning approaches have been applied to address this issue. Some models developed using supervised machine learning approaches have achieved high sensitivity or recall, however precision has been variable.
In a series of experiments, we will assess the performance of machine learning models to retrieve high-quality, high relevance evidence for clinical consideration from the biomedical literature. The models will be trained using an automated approach applied to a database of almost 100, 000 articles that have been tagged by highly trained research staff based on criteria for high-quality and assessed for clinical relevance by clinicians. We will evaluate and report on the effects of various classifiers, preprocessing steps, feature selection, and the use of balanced vs unbalanced datasets applied during model development on the performance of the derived supervised machine learning models.
The series was devised to improve the precision of the retrieval of high-quality articles by applying a machine learning classifier sequentially after using high sensitivity Boolean search filters to an ongoing literature surveillance process. Our multi-level analysis of the various steps of machine learning model development will help expand the existing knowledge base on the effect of each step on the performance of machine learning models.
更多查看译文
AI 理解论文
溯源树
样例
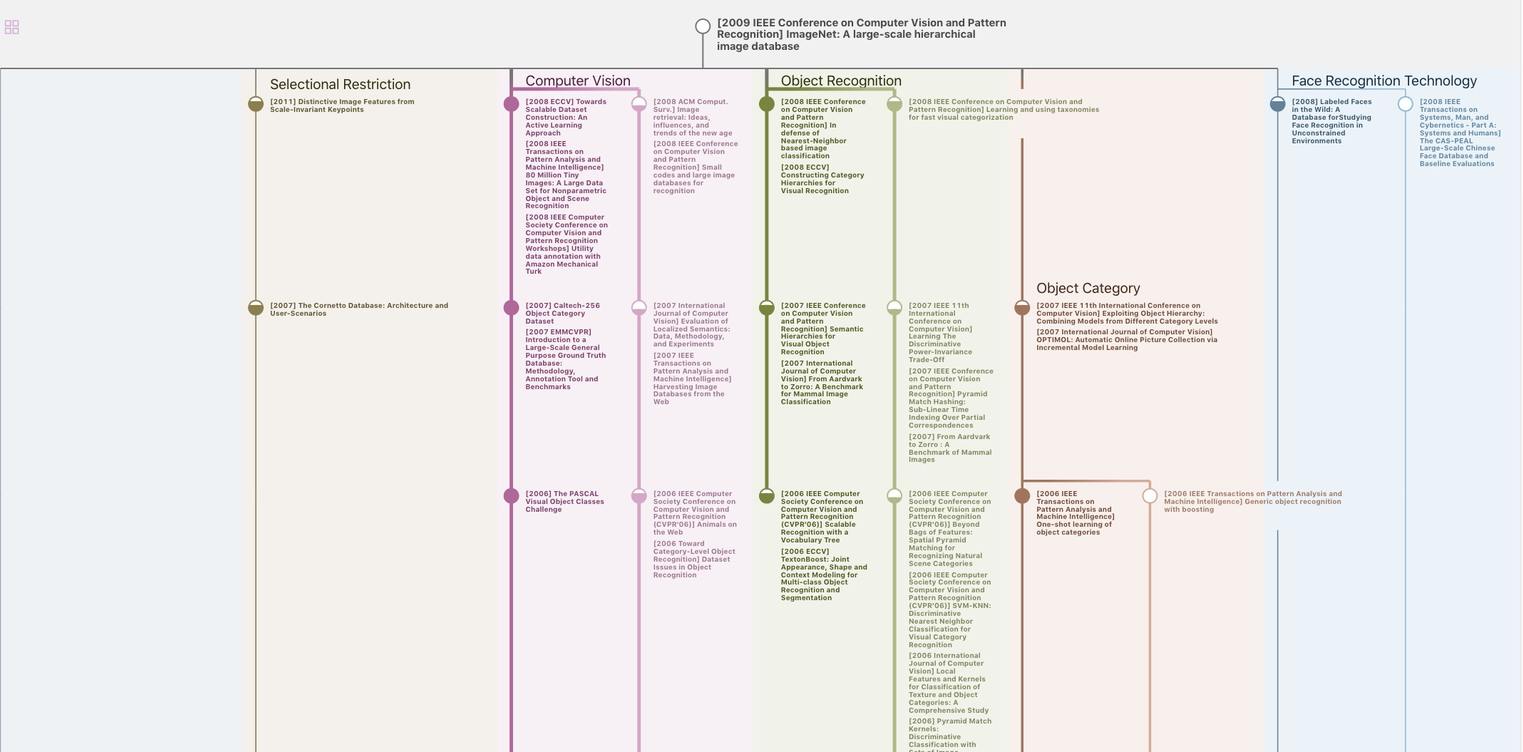
生成溯源树,研究论文发展脉络
Chat Paper
正在生成论文摘要