$\infty$-former: Infinite Memory Transformer
semanticscholar(2021)
摘要
Transformers struggle when attending to long contexts, since the amount of computation grows with the context length, and therefore they cannot model long-term memories effectively. Several variations have been proposed to alleviate this problem, but they all have a finite memory capacity, being forced to drop old information. In this paper, we propose the∞-former, which extends the vanilla transformer with an unbounded long-term memory. By making use of a continuous-space attention mechanism to attend over the long-term memory, the ∞-former’s attention complexity becomes independent of the context length. Thus, it is able to model arbitrarily long contexts and maintain “sticky memories” while keeping a fixed computation budget. Experiments on a synthetic sorting task demonstrate the ability of the ∞-former to retain information from long sequences. We also perform experiments on language modeling, by training a model from scratch and by fine-tuning a pretrained language model, which show benefits of unbounded long-term memories.
更多查看译文
关键词
memory
AI 理解论文
溯源树
样例
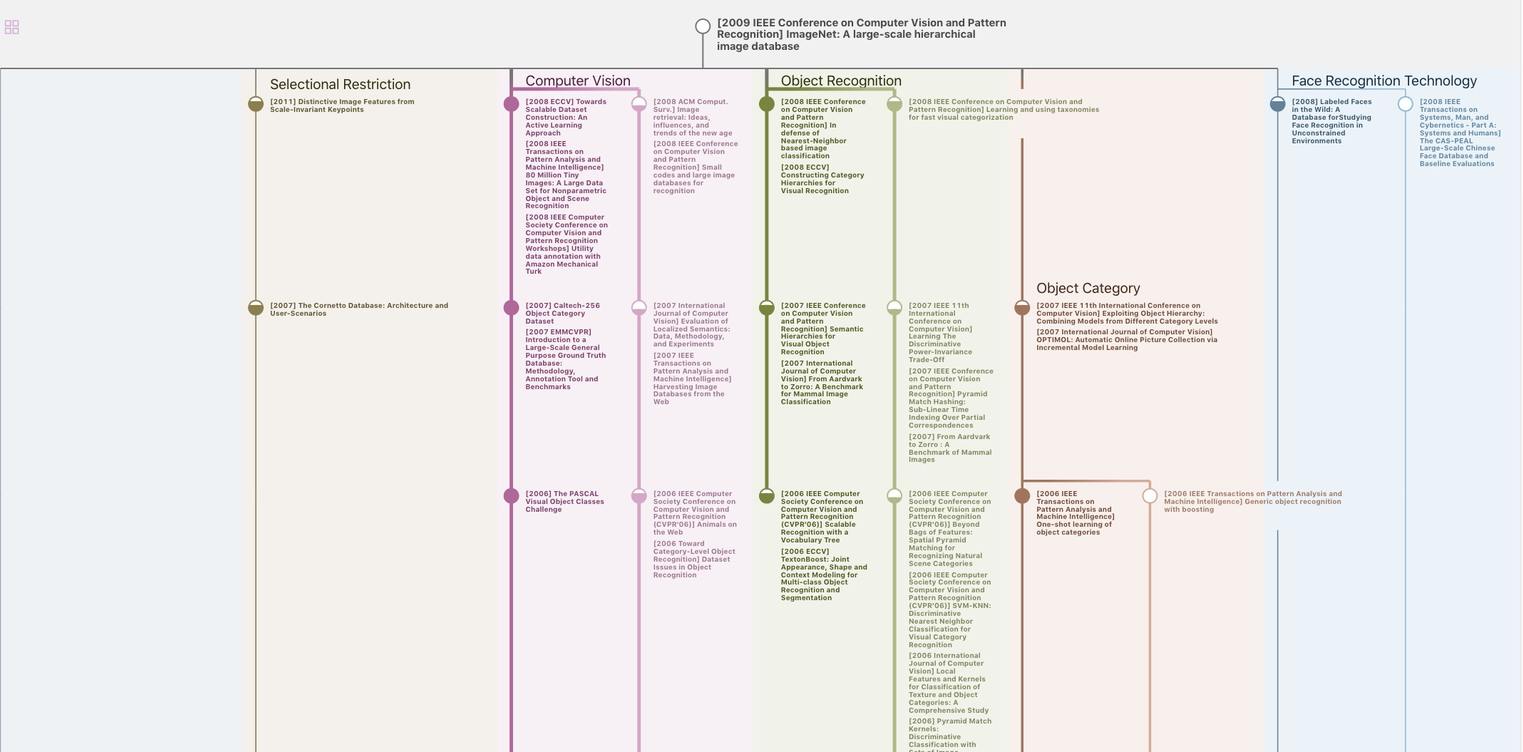
生成溯源树,研究论文发展脉络
Chat Paper
正在生成论文摘要