Approximation Capabilities of Wasserstein Generative Adversarial Networks
semanticscholar(2021)
摘要
In this paper, we study Wasserstein Generative Adversarial Networks (WGANs) using GroupSort neural networks as discriminators. We show that the error bound for the approximation of target distribution depends on both the width/depth (capacity) of generators and discriminators, as well as the number of samples in training. A quantified generalization bound is established for Wasserstein distance between the generated distribution and the target distribution. According to our theoretical results, WGANs have higher requirement for the capacity of discriminators than that of generators, which is consistent with some existing theories. More importantly, overly deep and wide (high capacity) generators may cause worse results (after training) than low capacity generators if discriminators are not strong enough. Numerical results on the synthetic data (swiss roll) and MNIST data confirm our theoretical results, and demonstrate that the performance by using GroupSort neural networks as discriminators is better than that of the original WGAN.
更多查看译文
AI 理解论文
溯源树
样例
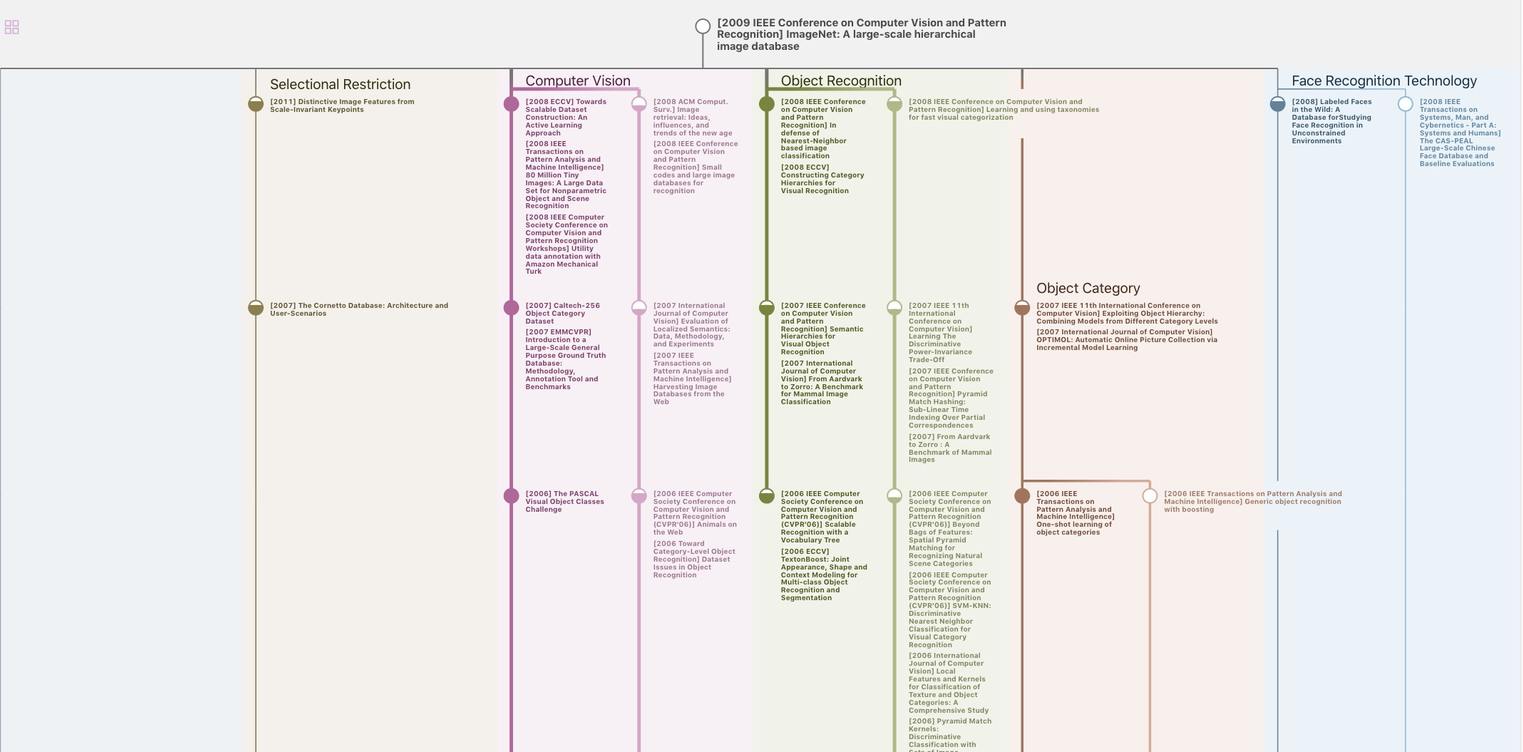
生成溯源树,研究论文发展脉络
Chat Paper
正在生成论文摘要