Strategies for EELS Data Analysis. Introducing UMAP and HDBSCAN for Dimensionality Reduction and Clustering
MICROSCOPY AND MICROANALYSIS(2022)
摘要
Hierarchical density-based spatial clustering of applications with noise (HDBSCAN) and uniform manifold approximation and projection (UMAP), two new state-of-the-art algorithms for clustering analysis, and dimensionality reduction, respectively, are proposed for the segmentation of core-loss electron energy loss spectroscopy (EELS) spectrum images. The performances of UMAP and HDBSCAN are systematically compared to the other clustering analysis approaches used in EELS in the literature using a known synthetic dataset. Better results are found for these new approaches. Furthermore, UMAP and HDBSCAN are showcased in a real experimental dataset from a core-shell nanoparticle of iron and manganese oxides, as well as the triple combination non-negative matrix factorization-UMAP-HDBSCAN. The results obtained indicate how the complementary use of different combinations may be beneficial in a real-case scenario to attain a complete picture, as different algorithms highlight different aspects of the dataset studied.
更多查看译文
关键词
clustering,dimensionality reduction,EELS,HDBSCAN,UMAP
AI 理解论文
溯源树
样例
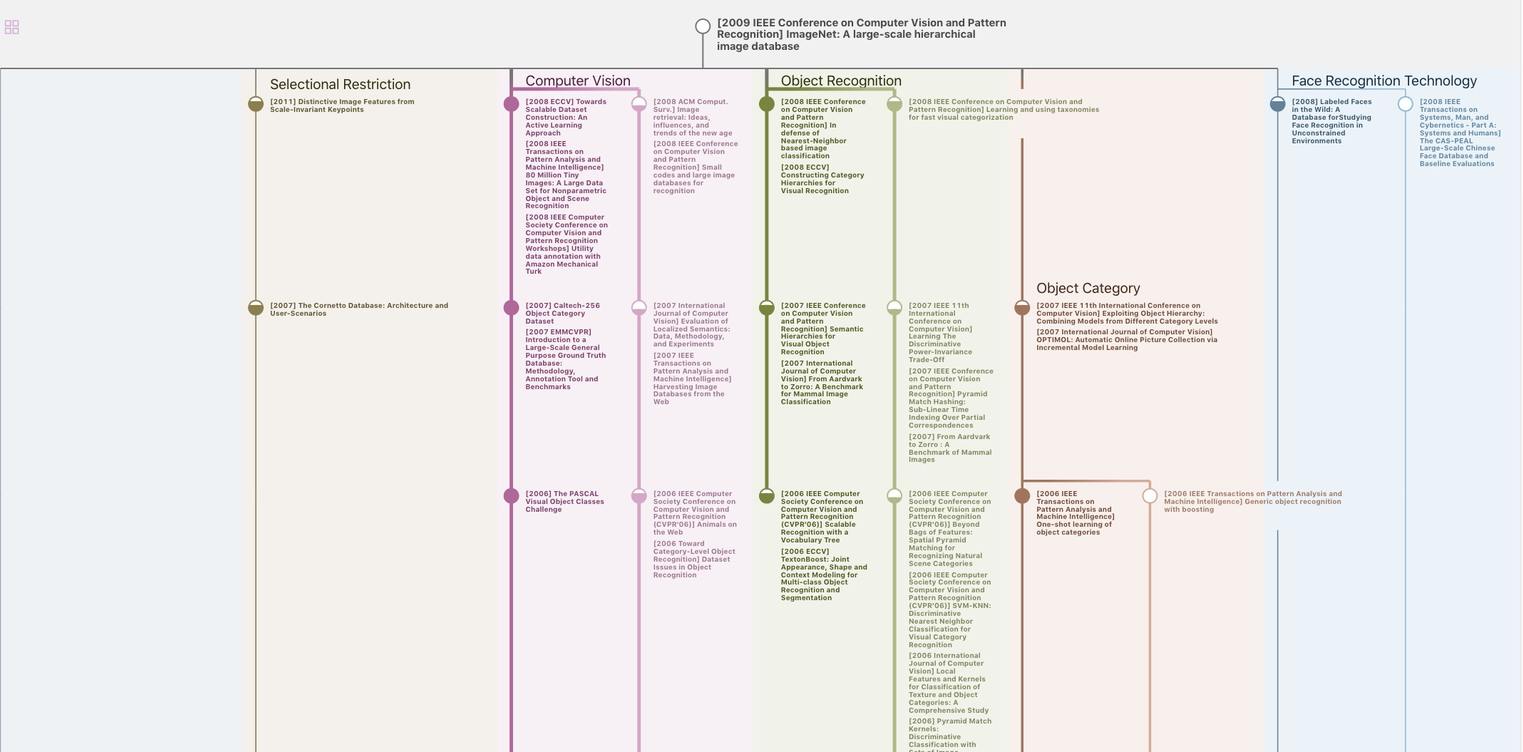
生成溯源树,研究论文发展脉络
Chat Paper
正在生成论文摘要