Fracture Identification in Well Logging Images: Two-Stage Adaptive Network
IEEE TRANSACTIONS ON INSTRUMENTATION AND MEASUREMENT(2022)
Abstract
Automatic fracture identification and segmentation in well logging images is increasingly critical but arduous because of extensive exploration for oil and gas. Domain adaptation for semantic segmentation is an appealing alternative to the lack of semantic fracture annotations. Nevertheless, previous domain adaptation strategies merely focus on a single level (e.g., input, intermediate features, or output), which could result in a large generalization error on new well logging images. In this article, a two-stage network architecture is proposed. In stage 1, a pattern transfer network (PTN) model is utilized to carry out a transformation from one domain to the other domain, making images visually similar between two datasets. Sequentially, in stage 2, an adversarial learning model with a generator and discriminator is introduced to make two domain images generated via PTN producing similar semantic segmentation. Furthermore, an attention module was embedded in the generator to filter irrelevant noise in semantic segmentation results. Real logging images were collected as the tested datasets by an ultrasonic imaging logging instrument. Compared to earlier conventional algorithms and domain adaptation methods, the proposed method is shown to obtain better visual quality and accurate segmentation outcomes.
MoreTranslated text
Key words
Image segmentation,Semantics,Generators,Visualization,Ultrasonic imaging,Oils,Training,Domain adaptation,fracture identification,semantic segmentation,two-stage adaptation,ultrasonic well logging image
AI Read Science
Must-Reading Tree
Example
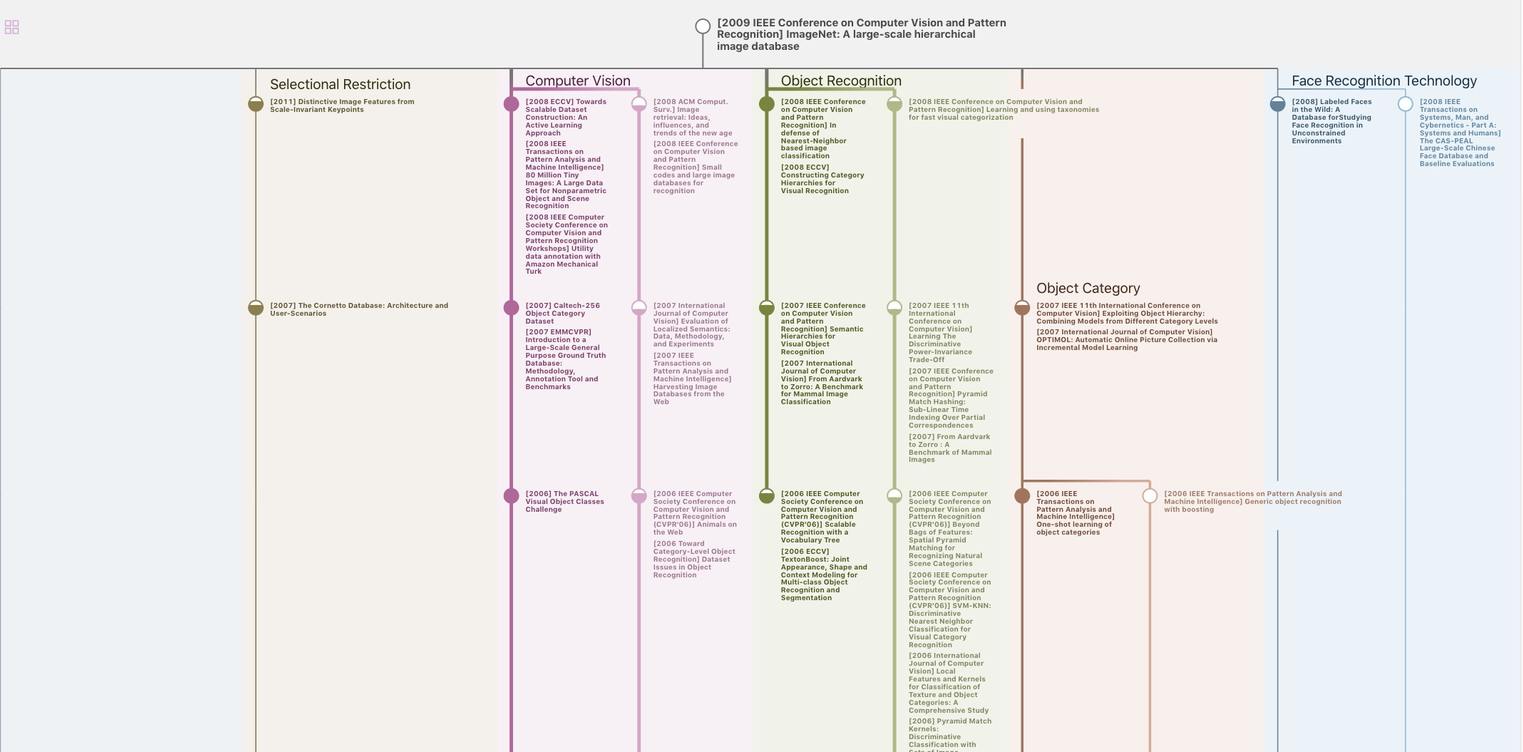
Generate MRT to find the research sequence of this paper
Chat Paper
Summary is being generated by the instructions you defined