Permuted proper orthogonal decomposition for analysis of advecting structures
JOURNAL OF FLUID MECHANICS(2021)
摘要
Flow data are often decomposed using proper orthogonal decomposition (POD) of the space-time separated form, q' (x, t) = Sigma(j) a(j) (t) phi(j) (x), which targets spatially correlated flow structures in an optimal manner. This paper analyses permuted POD (PPOD), which decomposes data as q' (x, t) = Sigma(j) a(j) (n) phi(j) (s, t), where x = (s, n) is a general spatial coordinate system, s is the coordinate along the bulk advection direction and n = (n(1), n(2)) are along mutually orthogonal directions normal to the advection characteristic. This separation of variables is associated with a fundamentally different inner product space for which PPOD is optimal and targets correlations in s, t space. This paper presents mathematical features of PPOD, followed by analysis of three experimental datasets from high-Reynolds-number, turbulent shear flows: a wake, a swirling annular jet and a jet in cross-flow. In the wake and swirling jet cases, the leading PPOD and space-only POD modes focus on similar features but differ in convergence rates and fidelity in capturing spatial and temporal information. In contrast, the leading PPOD and space-only POD modes for the jet in cross-flow capture completely different features - advecting shear layer structures and flapping of the jet column, respectively. This example demonstrates how the different inner product spaces, which order the PPOD and space-only POD modes according to different measures of variance, provide unique 'lenses' into features of advection-dominated flows, allowing complementary insights.
更多查看译文
关键词
turbulent reacting flows, shear layers, big data
AI 理解论文
溯源树
样例
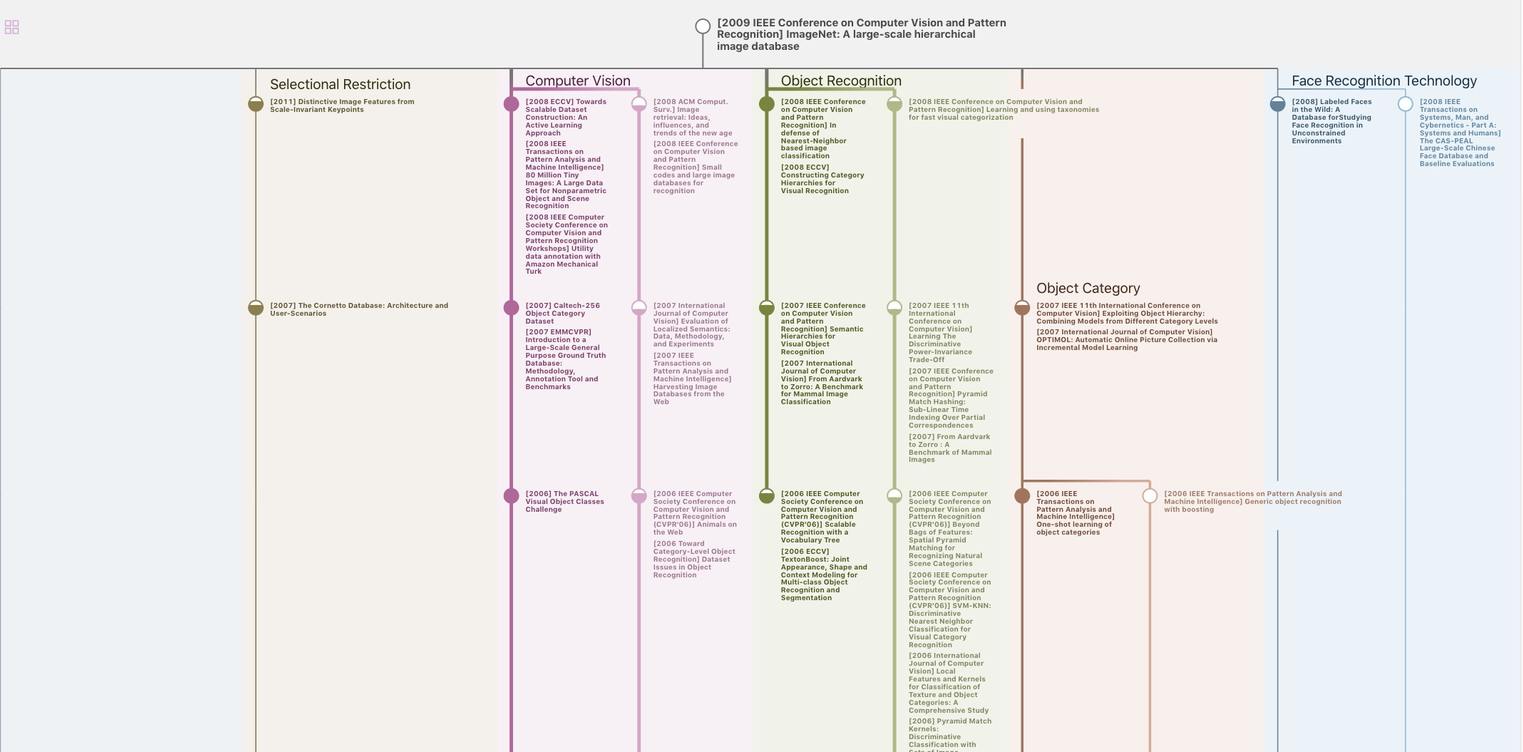
生成溯源树,研究论文发展脉络
Chat Paper
正在生成论文摘要