Improving Precipitation Forecasts with Convolutional Neural Networks
WEATHER AND FORECASTING(2023)
摘要
A machine learning method based on spatial convolution to capture complex spatial precipitation patterns is proposed to identify and reduce biases affecting predictions of a dynamical model. The method is based on a combina-tion of a classification and dual-regression model approach using modified U-Net convolutional neural networks (CNN) to postprocess daily accumulated precipitation over the U.S. West Coast. In this study, we leverage 34 years of high-resolution deterministic Western Weather Research and Forecasting (West-WRF) precipitation reforecasts as training data for the U-Net CNN. The data are split such that the test set contains 4 water years of data that encompass characteristic West Coast precipitation regimes: El Nin similar to o, La Nin similar to a, and dry and wet El Nin similar to o-Southern Oscillation (ENSO neutral) water years. On the unseen 4-yr dataset, the trained CNN yields a 12.9%-15.9% reduction in root-mean-square error (RMSE) and 2.7%-3.4% improvement in Pearson correlation (PC) over West-WRF for lead times of 1-4 days. Compared to an adapted model output statistics correction, the CNN reduces RMSE by 7.4%-8.9% and improves PC by 3.3%-4.2% across all events. Effectively, the CNN adds more than a day of predictive skill when compared to West-WRF. The CNN outper-forms the other methods also for the prediction of extreme events, which we define as the top 10% of events with the great-est average daily accumulated precipitation. The improvement over West-WRF's RMSE (PC) for these events is 19.8%-21.0% (4.9%-5.5%) and MOS's RMSE (PC) is 8.8%-9.7% (4.2%-4.7%). Hence, the proposed U-Net CNN shows significantly im-proved forecast skill over existing methods, highlighting a promising path forward for improving precipitation forecasts.
更多查看译文
关键词
Numerical weather prediction,forecasting,Postprocessing,Machine learning
AI 理解论文
溯源树
样例
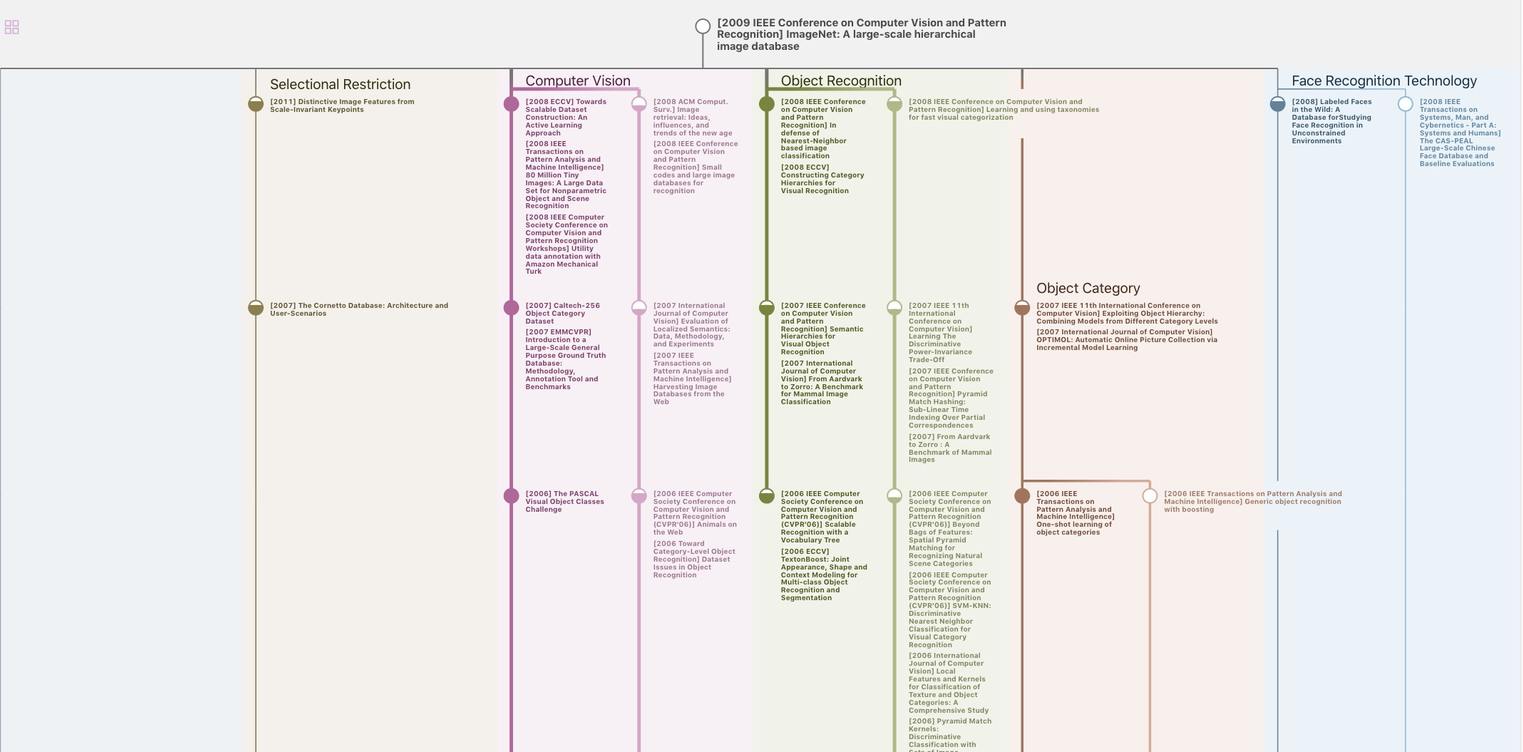
生成溯源树,研究论文发展脉络
Chat Paper
正在生成论文摘要