Iterative Gait Design for Cassie using DeepRL
semanticscholar(2019)
摘要
Deep reinforcement learning (DRL) is a promising approach for developing legged locomotion skills. However, the iterative design process that is inevitable in practice is poorly supported In this paper, we propose a practical method that allows the reward function to be fully redefined on each successive design iteration while limiting the deviation from the previous iteration. We characterize policies via sets of Deterministic Action Stochastic State (DASS) tuples, which represent the deterministic policy state-action pairs as sampled from the states visited by the trained stochastic policy. New policies are trained using a policy gradient algorithm which then mixes RL-based policy gradients with gradient updates defined by the DASS tuples. The tuples also allow for robust policy distillation to new network architectures. We demonstrate the effectiveness of this iterativedesign approach on the bipedal robot Cassie, achieving stable walking with different gait styles at various speeds.
更多查看译文
AI 理解论文
溯源树
样例
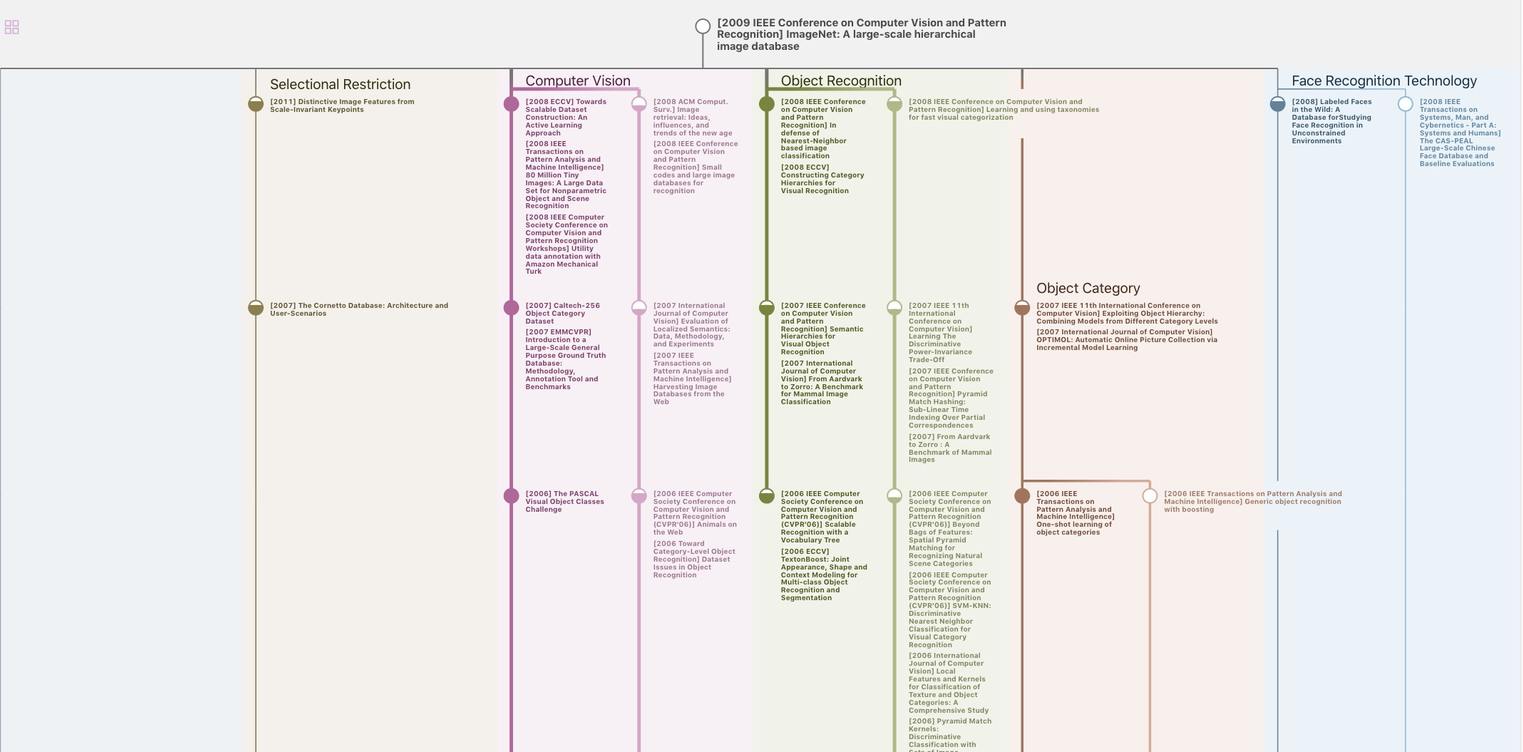
生成溯源树,研究论文发展脉络
Chat Paper
正在生成论文摘要