Learning Graph Representation by Aggregating Subgraphs via Mutual Information Maximization
Neurocomputing(2021)
摘要
In this paper, we introduce a self-supervised learning method to enhance the graph-level representations with the help of a set of subgraphs. For this purpose, we propose a universal framework to generate subgraphs in an auto-regressive way and then using these subgraphs to guide the learning of graph representation by Graph Neural Networks. Under this framework, we can get a comprehensive understanding of the graph structure in a learnable way. And to fully capture enough information of original graphs, we design three information aggregators: \textbf{attribute-conv}, \textbf{layer-conv} and \textbf{subgraph-conv} to gather information from different aspects. And to achieve efficient and effective contrastive learning, a Head-Tail contrastive construction is proposed to provide abundant negative samples. Under all proposed components which can be generalized to any Graph Neural Networks, in the unsupervised case, we achieve new state-of-the-art results in several benchmarks. We also evaluate our model on semi-supervised learning tasks and make a fair comparison to state-of-the-art semi-supervised methods.
更多查看译文
关键词
Graph representation, Mutual information, Self-supervised learning, Subgraphs samplings
AI 理解论文
溯源树
样例
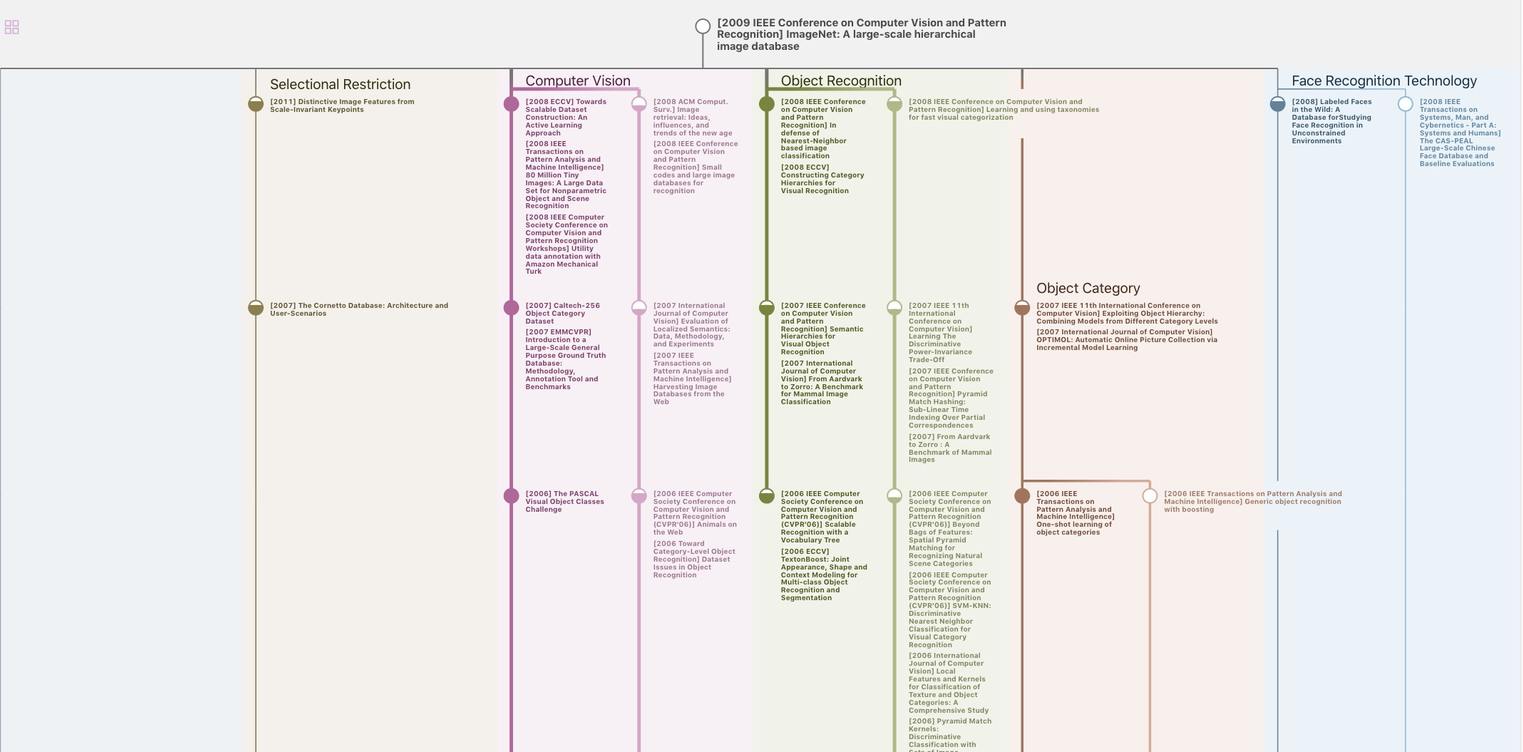
生成溯源树,研究论文发展脉络
Chat Paper
正在生成论文摘要