Global Texture Enhancement for Fake Face Detection In the Wild: Supplementary File
semanticscholar(2020)
摘要
To compare with existing works and evaluate the GramNet when training and testing images are in different semantic classes, we follow the setting in [4], which is also the “leave one out” setting in [5]. We evaluate on CycleGAN [6] dataset in which images of one category are set aside for testing while the remaining for training. There are a total number of 14 categories: Horse (H), Zebra (Z), Yosemite Summer (S), Yosemite Winter (W), Apple (A), Orange (O), Facades (F), CityScape Photo (City), Satellite Image (Map), Ukiyoe (U), Van Gogh (V), Cezanne (C), Monet (M) and Photo (P). Following [5], we also exclude the sketch and pixel-level semantic map from the dataset. We train Gram-Net with the same training strategy as in the main paper. Table 1 shows that Gram-Net achieves 98.49% mean accuracy of all the settings, which outperforms existing works. To be noted, we use ResNet-50 as our backbone compared to DesnetNet-121[3] in [5] and Xception71 [1] in [4]. We expect that deeper backbone networks will further benefit our performance. More importantly, [5] fails when GANs adopted in training and testing are with different upsampling structures. However, as shown in Table 3 cross-GAN setting ( StyleGAN: nearest-upsampling and PGGAN: deconvolution upsampling ) in the main paper, our approach works almost perfectly in this setting.
更多查看译文
AI 理解论文
溯源树
样例
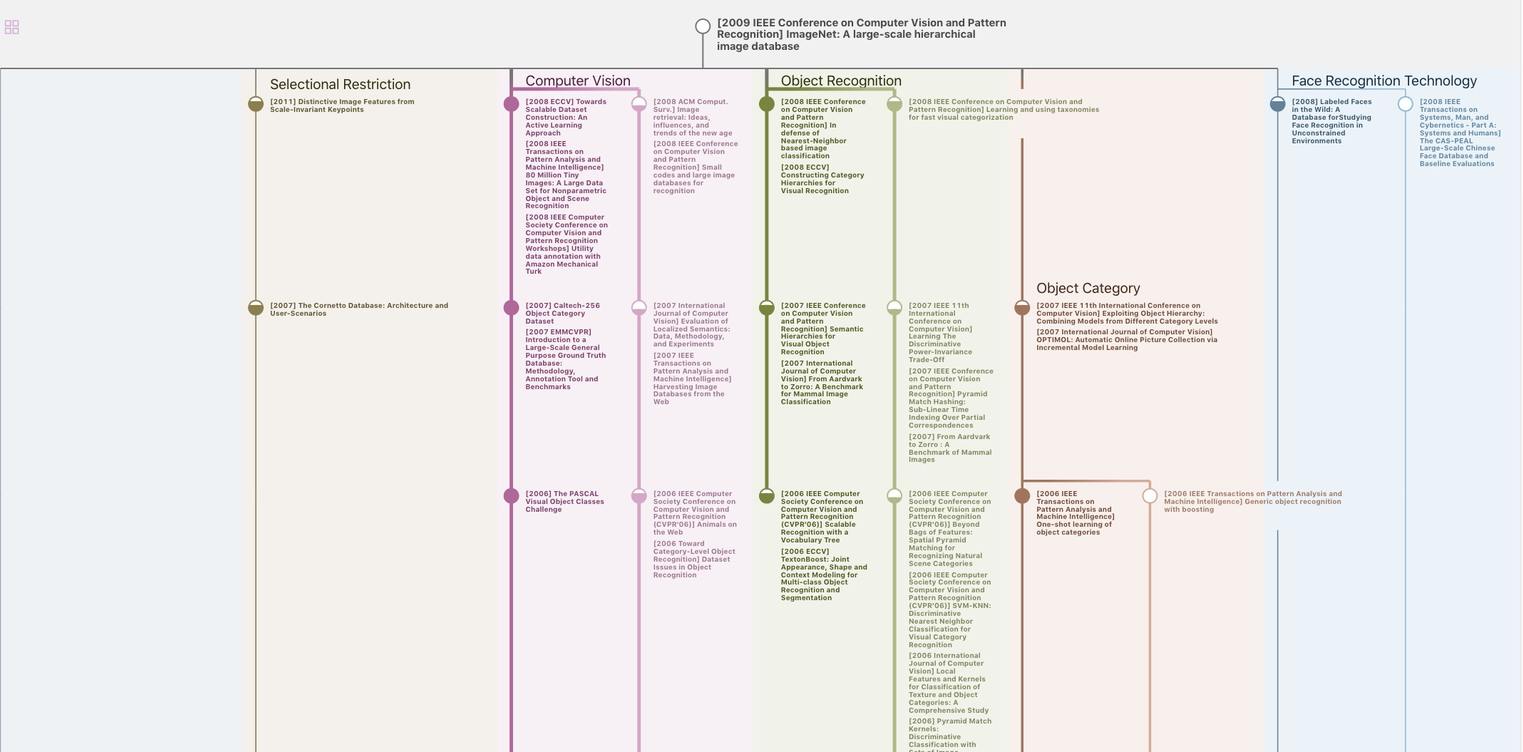
生成溯源树,研究论文发展脉络
Chat Paper
正在生成论文摘要