Auto-FuzzyJoin: Auto-Tune Fuzzy Joins Without Labeled Examples
semanticscholar(2021)
摘要
Fuzzy join is an important database operator that is widely used in practice. So far the research community has focused exclusively on the important problem of efficient fuzzy join algorithms. Today, practitioners also struggle with a daunting space of parameters to optimize join quality (e.g., distance-function, distancethresholds, tokenization-options, etc.), where a manual trial-anderror approach has become a major pain-point. This important parameter-tuning problem has thus far received surprisingly little attention from the research community. Motivated by the observation, we study the problem of “autotune” suitable fuzzy-join parameters on given input tables, without requiring explicit human input such as labelled training data. We develop an Auto-FuzzyJoin framework that, when given two tables L and R, and a user-specified target precision τ (say 0.9), can automatically configure fuzzy-join parameters meeting the precision target τ in expectation, while maximizing fuzzy-join recall (defined as the number of correctly joined records). We study the hardness of this problem, and develop effective algorithms that are extensively tested on both existing benchmarks as well as a new benchmark of 50 fuzzy-join tasks we created from Wikipedia data. Our evaluation suggests that the proposed Auto-FuzzyJoin significantly outperforms existing unsupervised approaches (e.g., Microsoft Excel and ZeroER). It is surprisingly competitive even against supervised approaches (e.g., Magellan and DeepMatcher) when 50% of ground-truth joins are used as training data. We will release our code and benchmark to facilitate future research. ACM Reference Format: Peng Li, Xiang Cheng, Xu Chu, Yeye He, and Surajit Chaudhuri. 2021. AutoFuzzyJoin: Auto-Tune Fuzzy JoinsWithout Labeled Examples. In Proceedings ∗Both authors contributed equally to this research. Permission to make digital or hard copies of all or part of this work for personal or classroom use is granted without fee provided that copies are not made or distributed for profit or commercial advantage and that copies bear this notice and the full citation on the first page. Copyrights for components of this work owned by others than ACM must be honored. Abstracting with credit is permitted. To copy otherwise, or republish, to post on servers or to redistribute to lists, requires prior specific permission and/or a fee. Request permissions from permissions@acm.org. SIGMOD ’21, June 20–25, 2021, Xi’an, Shaanxi, China © 2021 Association for Computing Machinery. ACM ISBN 978-x-xxxx-xxxx-x/YY/MM. . . $15.00 https://doi.org/10.1145/nnnnnnn.nnnnnnn of 2021 International Conference on Management of Data (SIGMOD ’21).ACM, New York, NY, USA, 16 pages. https://doi.org/10.1145/nnnnnnn.nnnnnnn
更多查看译文
AI 理解论文
溯源树
样例
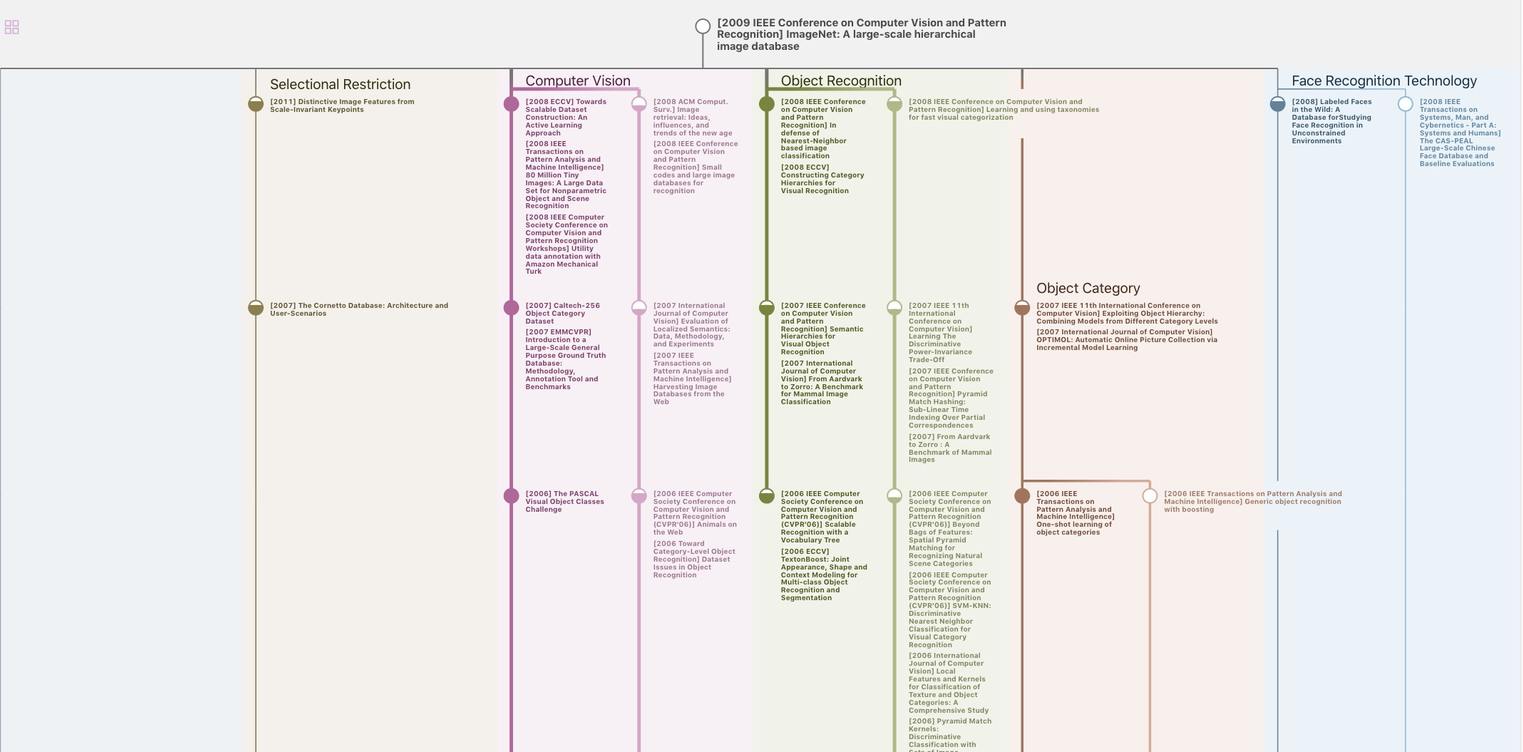
生成溯源树,研究论文发展脉络
Chat Paper
正在生成论文摘要