Federated Feature Selection for Cyber-Physical Systems of Systems
IEEE Transactions on Vehicular Technology(2022)
摘要
Autonomous vehicles (AVs) generate a massive amount of multi-modal data that once collected and processed through Machine Learning algorithms, enable AI-based services at the Edge. In fact, only a subset of the collected data present informative attributes to be exploited at the Edge. Therefore, extracting such a subset is of utmost importance to limit computation and communication workloads. Doing that in a distributed manner imposes the AVs to cooperate in finding an agreement on which attributes should be sent to the Edge. In this work, we address such a problem by proposing a federated feature selection (FFS) algorithm where the AVs collaborate to filter out, iteratively, the less relevant attributes in a distributed manner, without any exchange of raw data, thought two different components: a Mutual-Information-based feature selection algorithm run by the AVs and a novel aggregation function based on the Bayes theorem executed on the Edge. The FFS algorithm has been tested on two reference datasets: MAV with images and inertial measurements of a monitored vehicle, WESAD with a collection of samples from biophysical sensors to monitor a relative passenger. The numerical results show that the AVs converge to a minimum achievable subset of features with both the datasets, i.e., 24 out of 2166 (99%) in MAV and 4 out of 8 (50%) in WESAD, respectively, preserving the informative content of data.
更多查看译文
关键词
Feature extraction,Monitoring,Machine learning algorithms,Distance learning,Computer aided instruction,Training,Distributed databases,Artificial intelligence,autonomous system,feature selection,federated learning,human state monitoring,Internet of things,machine learning
AI 理解论文
溯源树
样例
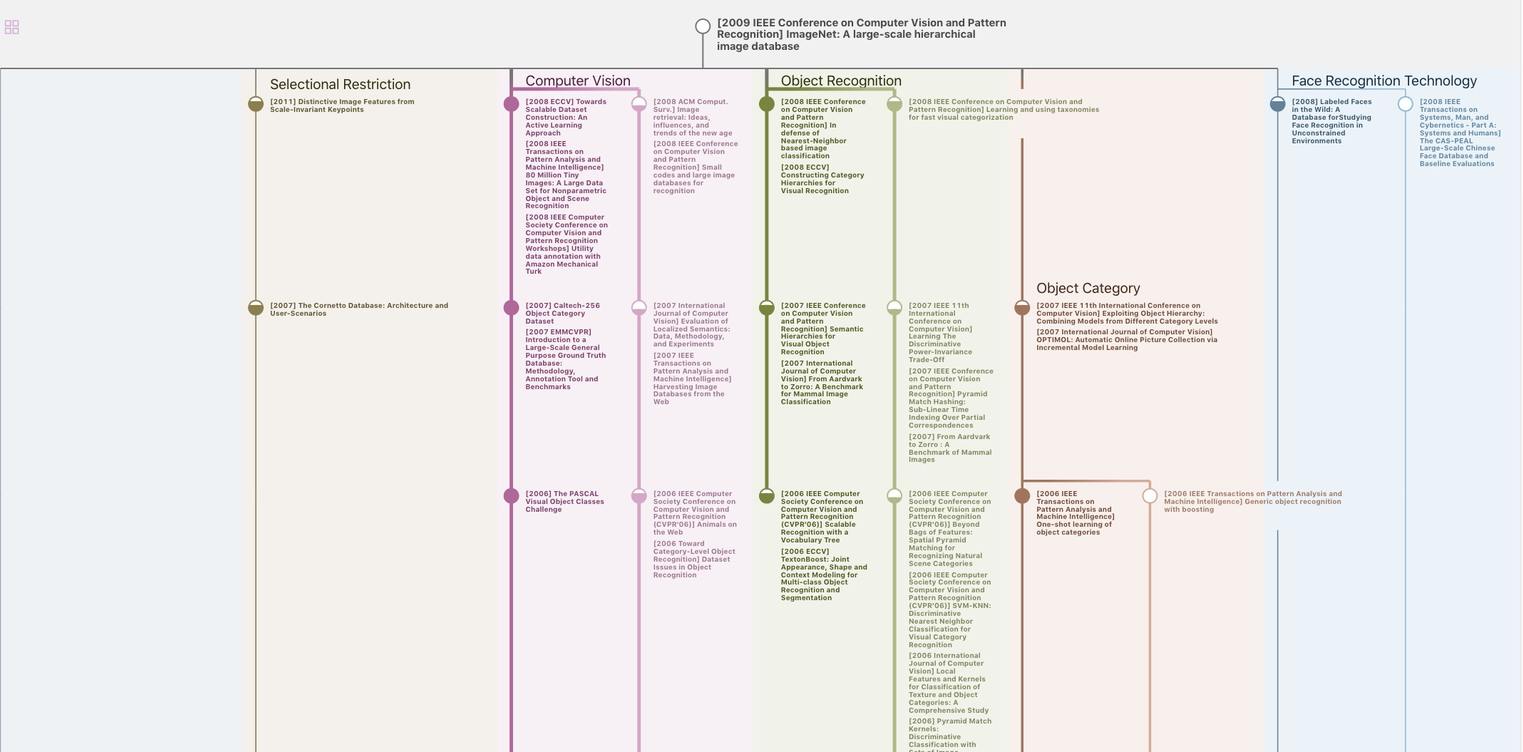
生成溯源树,研究论文发展脉络
Chat Paper
正在生成论文摘要