A Multimodal Feature Selection Method for Remote Sensing Data Analysis Based on Double Graph Laplacian Diagonalization
IEEE JOURNAL OF SELECTED TOPICS IN APPLIED EARTH OBSERVATIONS AND REMOTE SENSING(2021)
摘要
When dealing with multivariate remotely sensed records collected by multiple sensors, an accurate selection of information at the data, feature, or decision level is instrumental in improving the scenes' characterization. This will also enhance the system's efficiency and provide more details on modeling the physical phenomena occurring on the Earth's surface. In this article, we introduce a flexible and efficient method based on graph Laplacians for information selection at different levels of data fusion. The proposed approach combines data structure and information content to address the limitations of existing graph-Laplacian-based methods in dealing with heterogeneous datasets. Moreover, it adapts the selection to each homogenous area of the considered images according to their underlying properties. Experimental tests carried out on several multivariate remote sensing datasets show the consistency of the proposed approach.
更多查看译文
关键词
Laplace equations,Dimensionality reduction,Sensor phenomena and characterization,Kernel,Feature extraction,Earth,Data analysis,Gaussian kernel (GK),graph Laplacians,multimodal remote sensing,mutual information (MI),unsupervised information selection
AI 理解论文
溯源树
样例
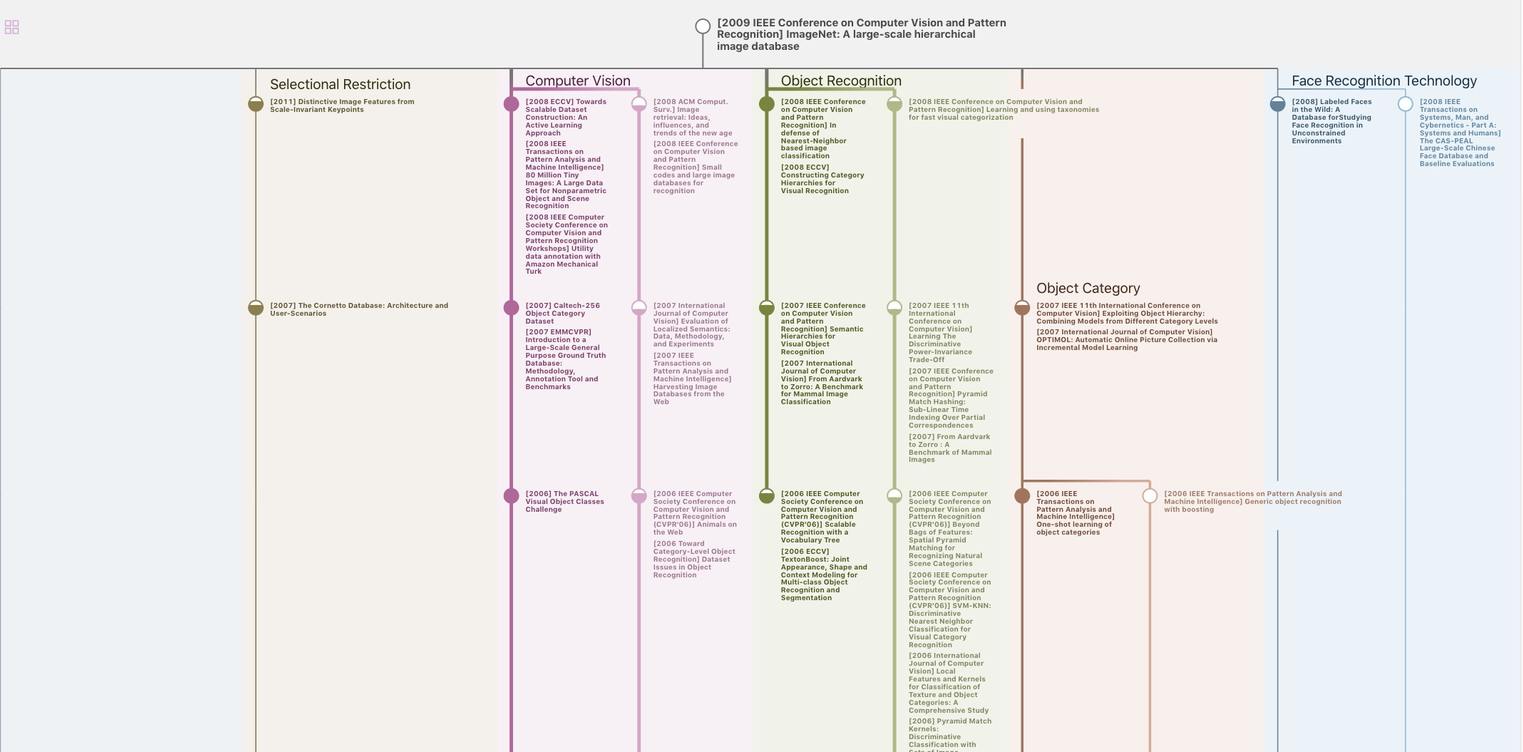
生成溯源树,研究论文发展脉络
Chat Paper
正在生成论文摘要