Efficient Black-Box Combinatorial Optimization
semanticscholar(2020)
Abstract
We consider the problem of black-box function optimization over combinatorial domains. Despite the vast literature on black-box function optimization over continuous domains, not much attention has been paid to learning models for optimization over combinatorial domains until recently. Nonetheless, optimization of such functions using state-of-the-art Bayesian optimization techniques, specifically designed for combinatorial domains, still remains challenging due to the associated computational complexity. To address this problem, we propose a computationally efficient model learning algorithm based on exponential weight updates. In particular, we use multilinear polynomials as surrogate model for functions over the Boolean hypercube. We further extend this representation via a group-theoretic Fourier expansion to address functions over categorical variables. Numerical experiments over synthetic benchmarks as well as real-world biological sequence design problems demonstrate the competitive or superior performance of the proposed algorithms versus a state-of-the-art Bayesian optimization algorithm while reducing the computational cost by multiple orders of magnitude.
MoreTranslated text
AI Read Science
Must-Reading Tree
Example
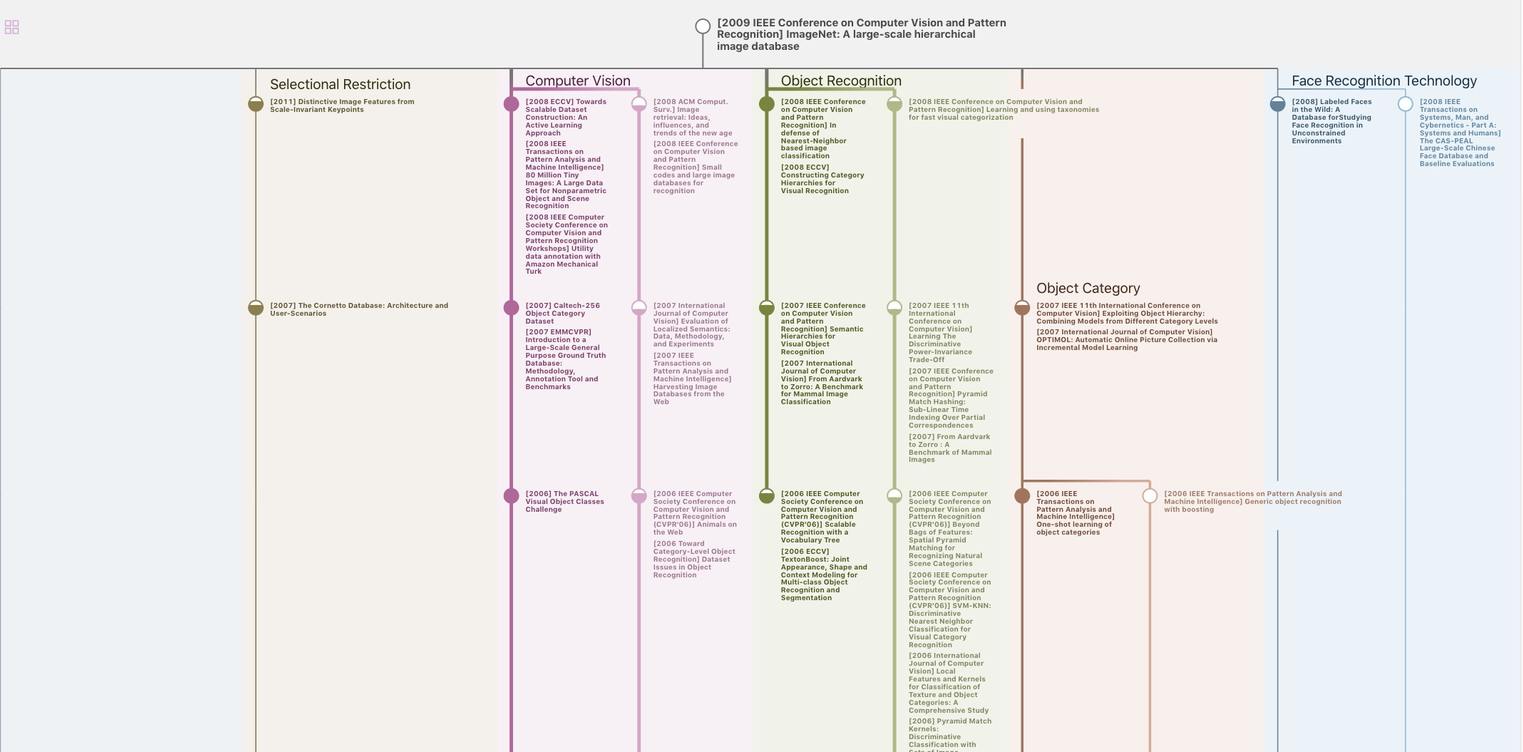
Generate MRT to find the research sequence of this paper
Chat Paper
Summary is being generated by the instructions you defined