Tractable approximation of hard uncertain optimization problems
semanticscholar(2020)
摘要
Robust Optimization is a widespread approach to treat uncertainty in optimization problems. Finding a computationally tractable formulation of the robust counterpart of an uncertain optimization problem is a key step in applying this approach. Techniques for finding a computationally tractable robust counterpart are available for constraints concave in the uncertain parameters. In many problems, however, the uncertain parameters appear in a convex way, which is problematic as no general techniques exist for such problems. In this paper, we provide a systematic way to construct conservative approximations to such problems. Specifically, we reformulate the original problem as an adjustable robust optimization problem in which the nonlinearity of the original problem is captured by the new uncertainty set. This adjustable robust optimization problem is linear whenever the original uncertainty set is polyhedral, which allows for the application of a multitude of techniques from adjustable robust optimization. Additionally, we prove that preprocessing a constraint with a concave transformation that preserves its convexity can tighten the conservative approximation obtained. We subsequently apply our theory to quadratic constraints, constraints that are the sum of maxima and the sum of maxima squared, as well as constraints from geometric programming. We demonstrate the quality of the approximations with a study of geometric programming problems and numerical examples from radiotherapy optimization, which contain a constraint of the sum of maxima squared type.
更多查看译文
AI 理解论文
溯源树
样例
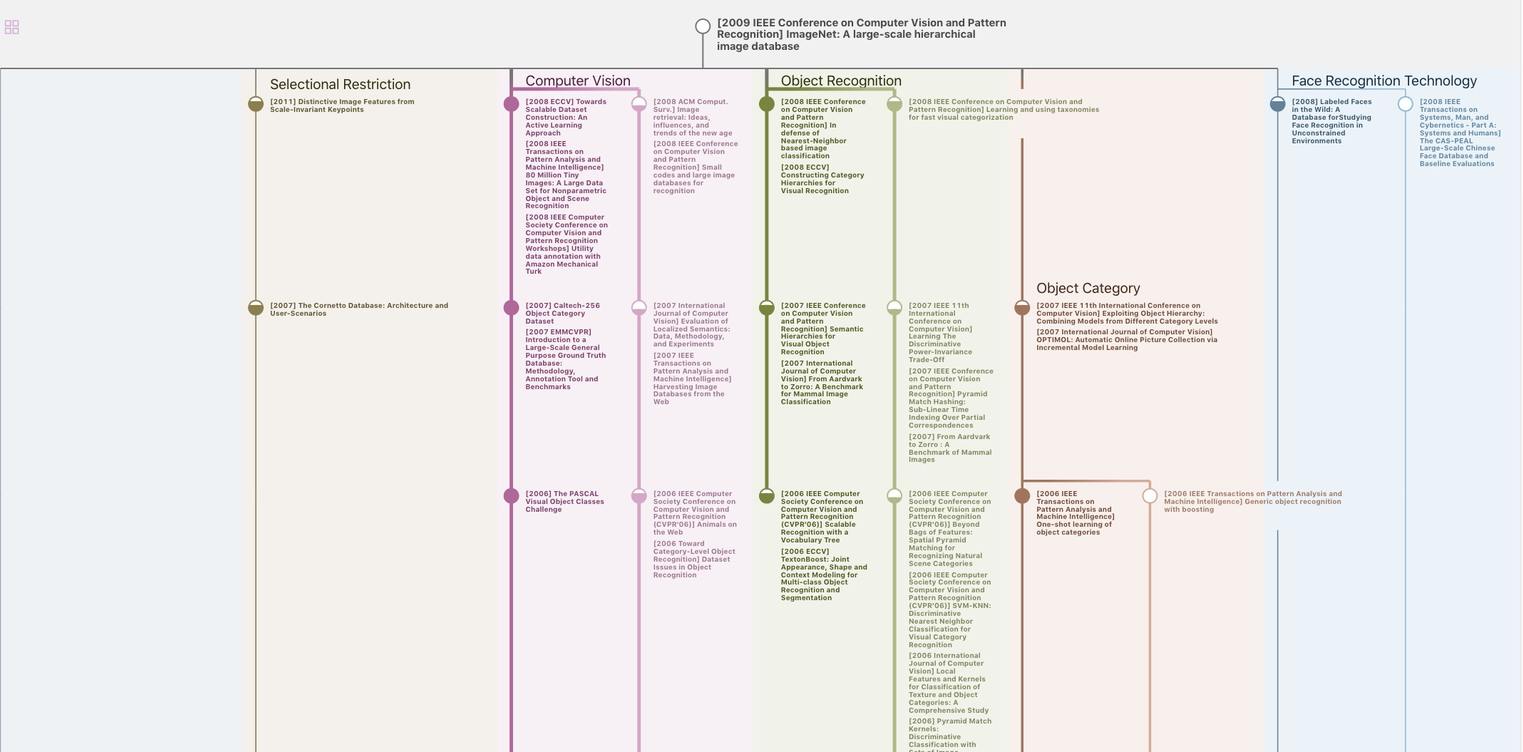
生成溯源树,研究论文发展脉络
Chat Paper
正在生成论文摘要