Can Expressive Posterior Approximations Improve Variational Continual Learning?
semanticscholar(2020)
摘要
Mean field variational inference (MFVI) has been successfully used in the past for continual learning. However, Gaussian mean field approximation has been shown to be inferior to more expressive forms of posterior approximation for training latent variable models and single task Bayesian neural networks (BNNs). In this paper, we examine whether expressive posterior approximations obtained with normalizing flows (NF) can result in improved continual learning compared to the mean field approach. Results from our preliminary experiments on the Permuted MNIST benchmark indicate that with longer training durations, over all tasks, BNNs with NF perform marginally better than BNNs with MFVI. Additionally, BNNs with NF are superior to BNNs with MFVI at remembering more recent tasks, while the performance on older tasks is similar between the two methods.
更多查看译文
AI 理解论文
溯源树
样例
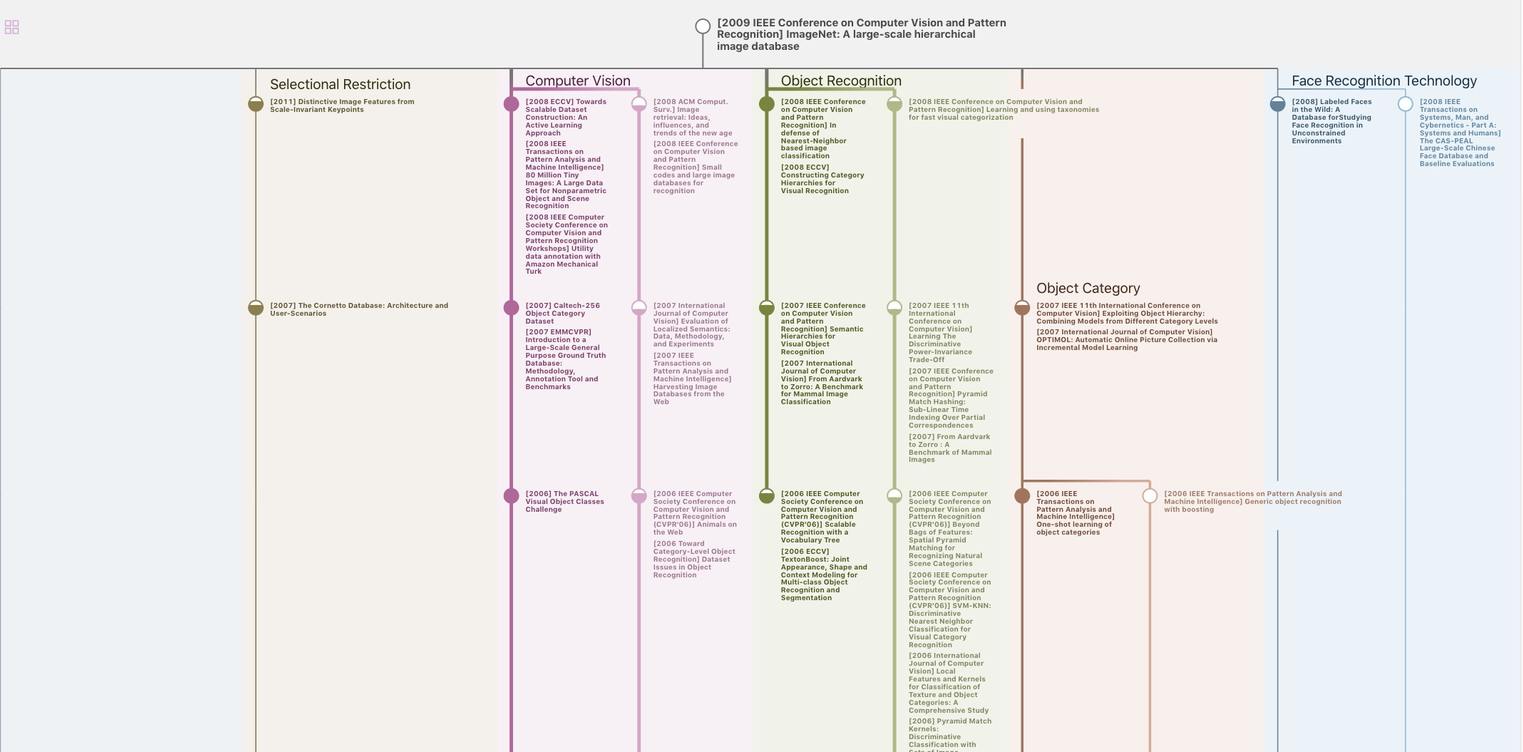
生成溯源树,研究论文发展脉络
Chat Paper
正在生成论文摘要