On using Focal Loss for Neural Network Calibration
semanticscholar(2020)
Abstract
Miscalibration – a mismatch between a model’s confidence and its correctness – of Deep Neural Networks (DNNs) makes their predictions hard to rely on. Ideally, we want networks to be accurate and calibrated. In this work, we study focal loss as an alternative to the conventional crossentropy loss and show that, focal loss allows us to learn models that are comparitively well calibrated while preserving accuracy. We provide a thorough analysis of the factors causing miscalibration, and use the insights we glean from this to justify the superior performance of focal loss. Finally, we perform extensive experiments on a variety of datasets, and with a wide variety of network architectures, and show that focal loss indeed achieves excellent calibration without compromising on accuracy in almost all cases.
MoreTranslated text
AI Read Science
Must-Reading Tree
Example
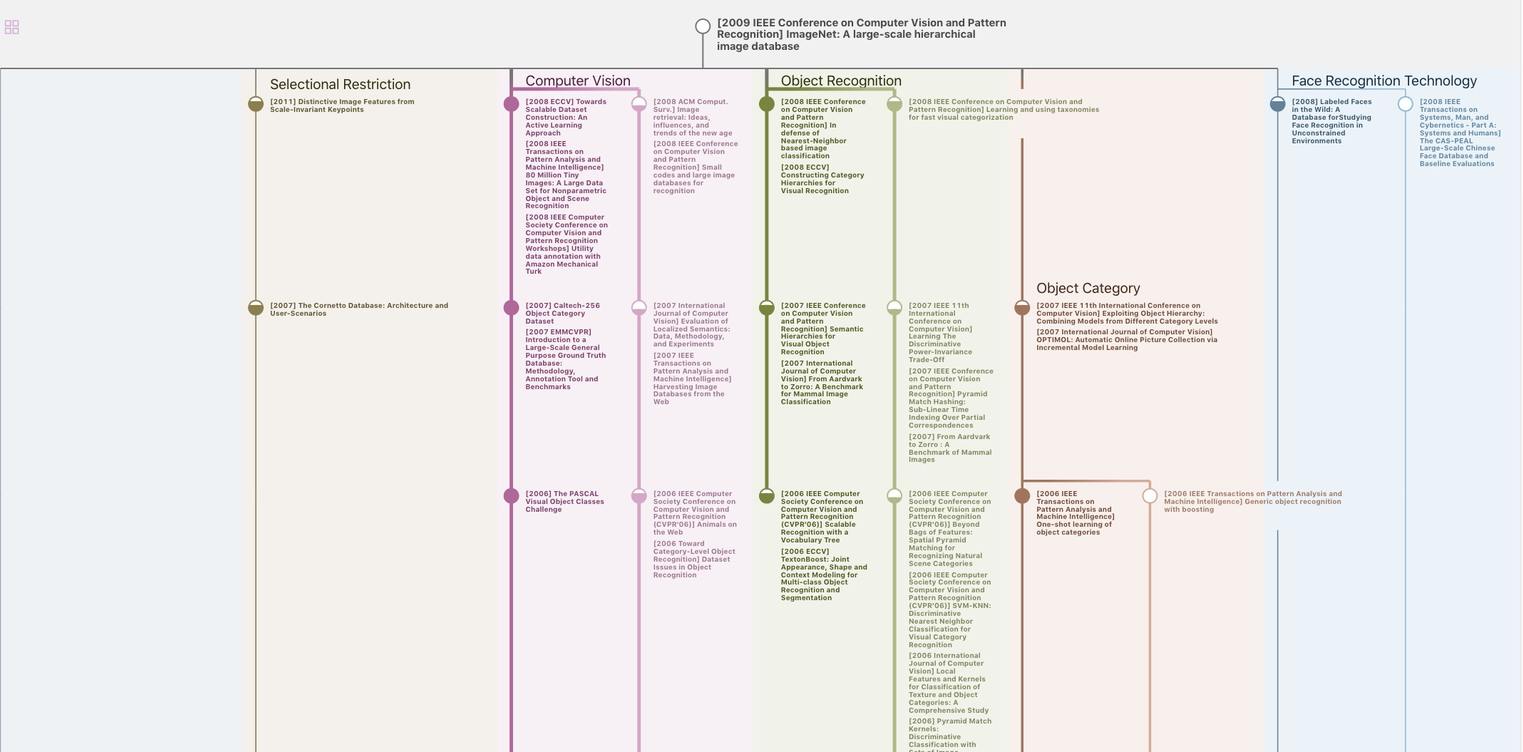
Generate MRT to find the research sequence of this paper
Chat Paper
Summary is being generated by the instructions you defined