Multi-resolution deep learning pipeline for dense large scale point clouds
ArXiv(2021)
Abstract
Recent development of 3D sensors allows the acquisition of extremely dense 3D point clouds of large-scale scenes. The main challenge of processing such large point clouds remains in the size of the data, which induce expensive computational and memory cost. In this context, the full resolution cloud is particularly hard to process, and details it brings are rarely exploited. Although fine-grained details are important for detection of small objects, they can alter the local geometry of large structural parts and mislead deep learning networks. In this paper, we introduce a new generic deep learning pipeline to exploit the full precision of large scale point clouds, but only for objects that require details. The core idea of our approach is to split up the process into multiple sub-networks which operate on different resolutions and with each their specific classes to retrieve. Thus, the pipeline allows each class to benefit either from noise and memory cost reduction of a sub-sampling or from fine-grained details. CCS Concepts • Computing methodologies → Supervised learning by classification; Neural networks; • Applied computing → Architecture (buildings);
MoreTranslated text
Key words
dense large scale point
AI Read Science
Must-Reading Tree
Example
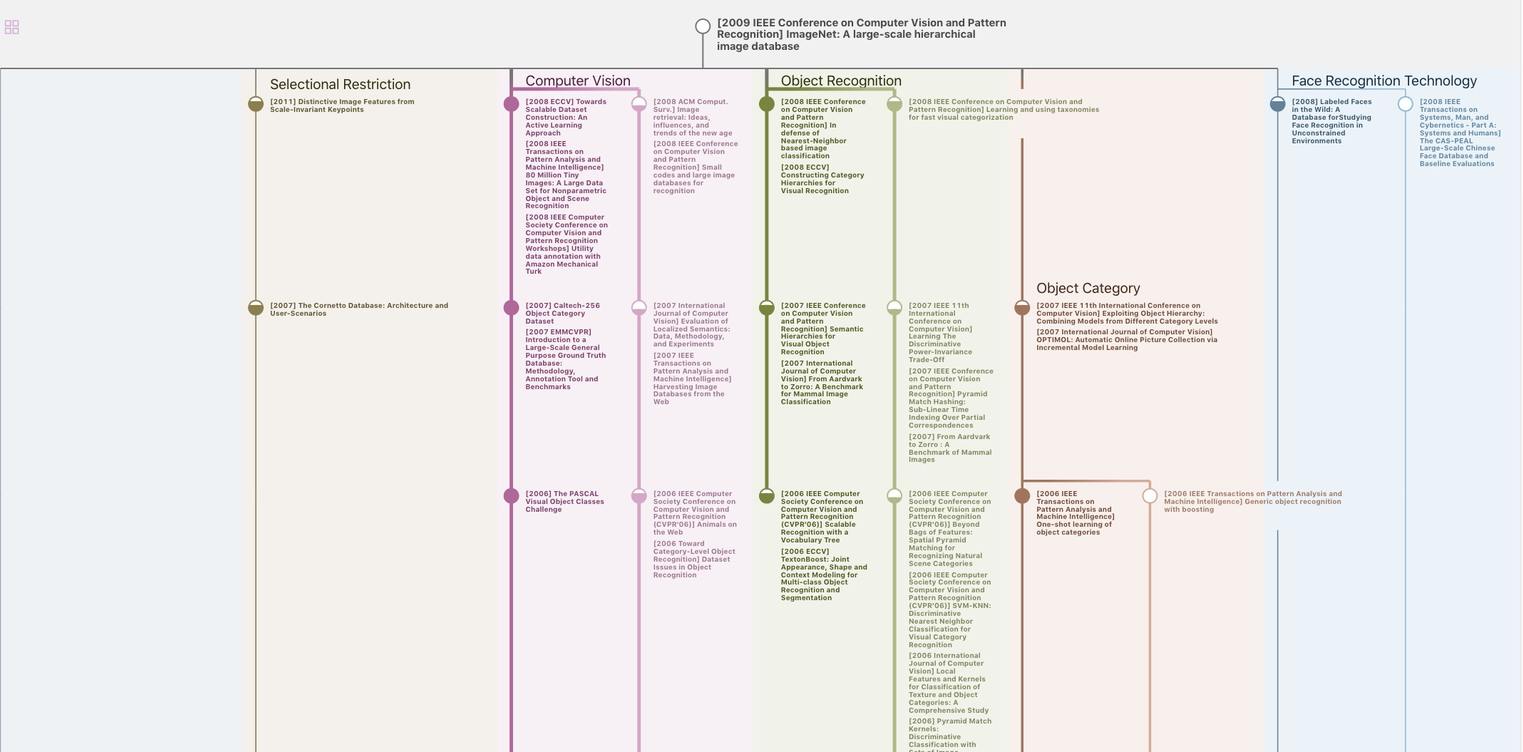
Generate MRT to find the research sequence of this paper
Chat Paper
Summary is being generated by the instructions you defined