A Crowdsourcing Approach to Develop Machine Learning Models to Quantify Radiographic Joint Damage in Rheumatoid Arthritis
medRxiv(2021)
摘要
To develop machine learning methods to quantify joint damage in patients with rheumatoid arthritis (RA), we developed the RA2 DREAM Challenge, a crowdsourced competition that utilized existing radiographic images and "gold-standard" scores on 674 sets of films from 562 patients. Training and leaderboard sets were provided to participants to develop methods to quantify joint space narrowing and erosions. In the final round, participants submitted containerized codes on a test set; algorithms were evaluated using weighted root mean square error (RMSE). In the leaderboard round, there were 173 submissions from 26 teams in 7 countries. Of the 13 submissions in the final round, four top-performing teams were identified. Robustness of results was assessed using Bayes factor and validated using an independent set of radiographs. The top-performing algorithms, which consisted of different styles of deep learning models, provided accurate and robust quantification of joint damage in RA. Ultimately, these methods lay the groundwork to accelerate research and help clinicians to optimize treatments to minimize joint damage.
更多查看译文
关键词
crowdsourcing approach,machine learning models,radiographic joint damage,machine learning,rheumatoid arthritis
AI 理解论文
溯源树
样例
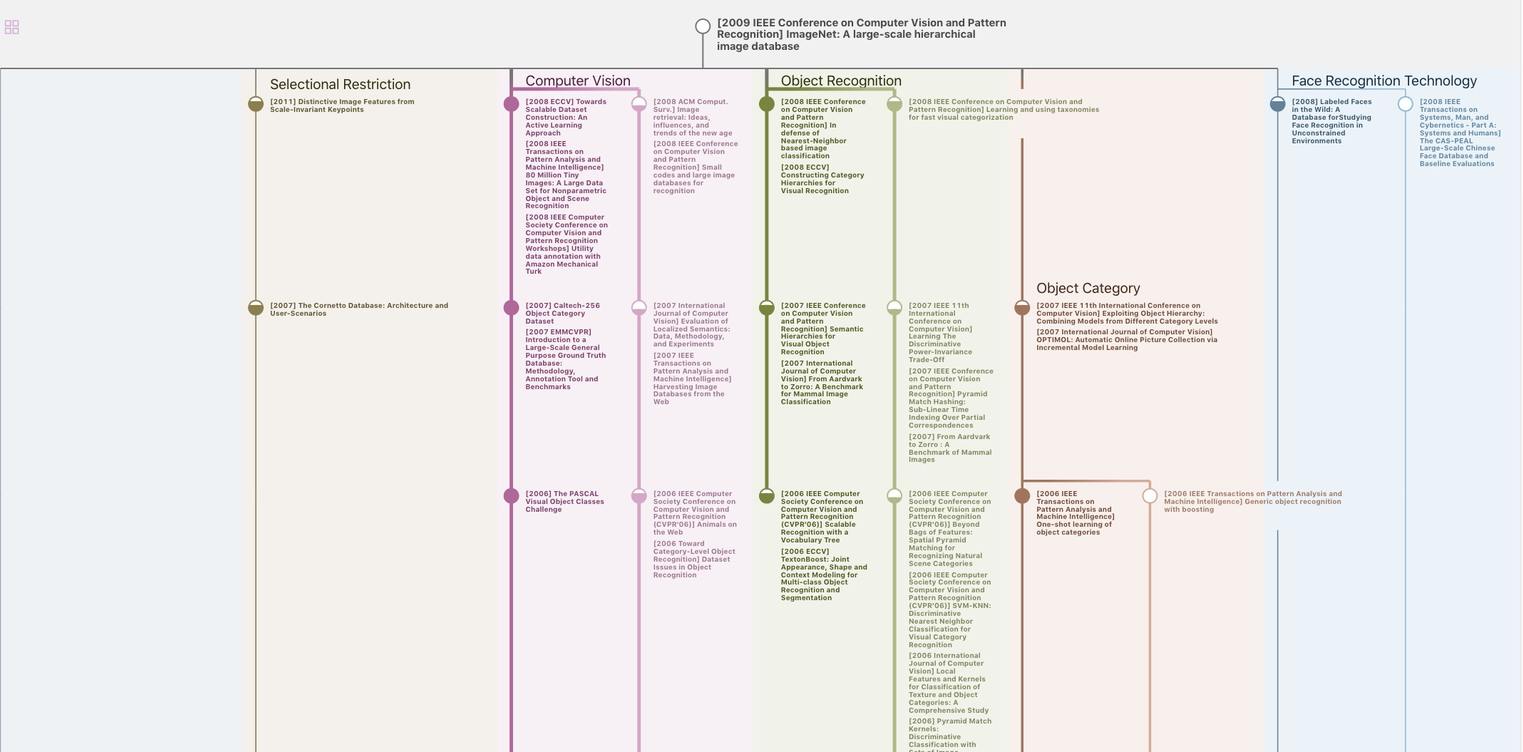
生成溯源树,研究论文发展脉络
Chat Paper
正在生成论文摘要