A Comparative Study of Energy Big Data Analysis for Product Management in a Smart Factory
JOURNAL OF ORGANIZATIONAL AND END USER COMPUTING(2022)
摘要
Energy is one of the key inputs for a country's economic growth and social development. Analysis and modeling of industrial energy are currently time-intensive processes because more and more energy is consumed for economic growth in a smart factory. This study aims to present and analyse the predictive models of the data-driven system to be used by appliances and find the most significant product item. With repeated cross-validation, three statistical models were trained and tested in a test set: 1) general linear regression model (GLM), 2) support vector machine (SVM), and 3) boosting tree (BT). The performance of prediction models were measured by R2 error, root mean squared error (RMSE), mean absolute error (MAE), and coefficient of variation (CV). The best model from the study is the support vector machine (SVM) that has been able to provide R2 of 0.86 for the training data set and 0.85 for the testing data set with a low coefficient of variation, and the most significant product of this smart factory is Skelp.
更多查看译文
关键词
Big Data Analysis,Boosting Tree,Correlation,Data Mining,Energy Consumption,General Linear Regression,Principal Component Analysis,Product Management,Support Vector Regression
AI 理解论文
溯源树
样例
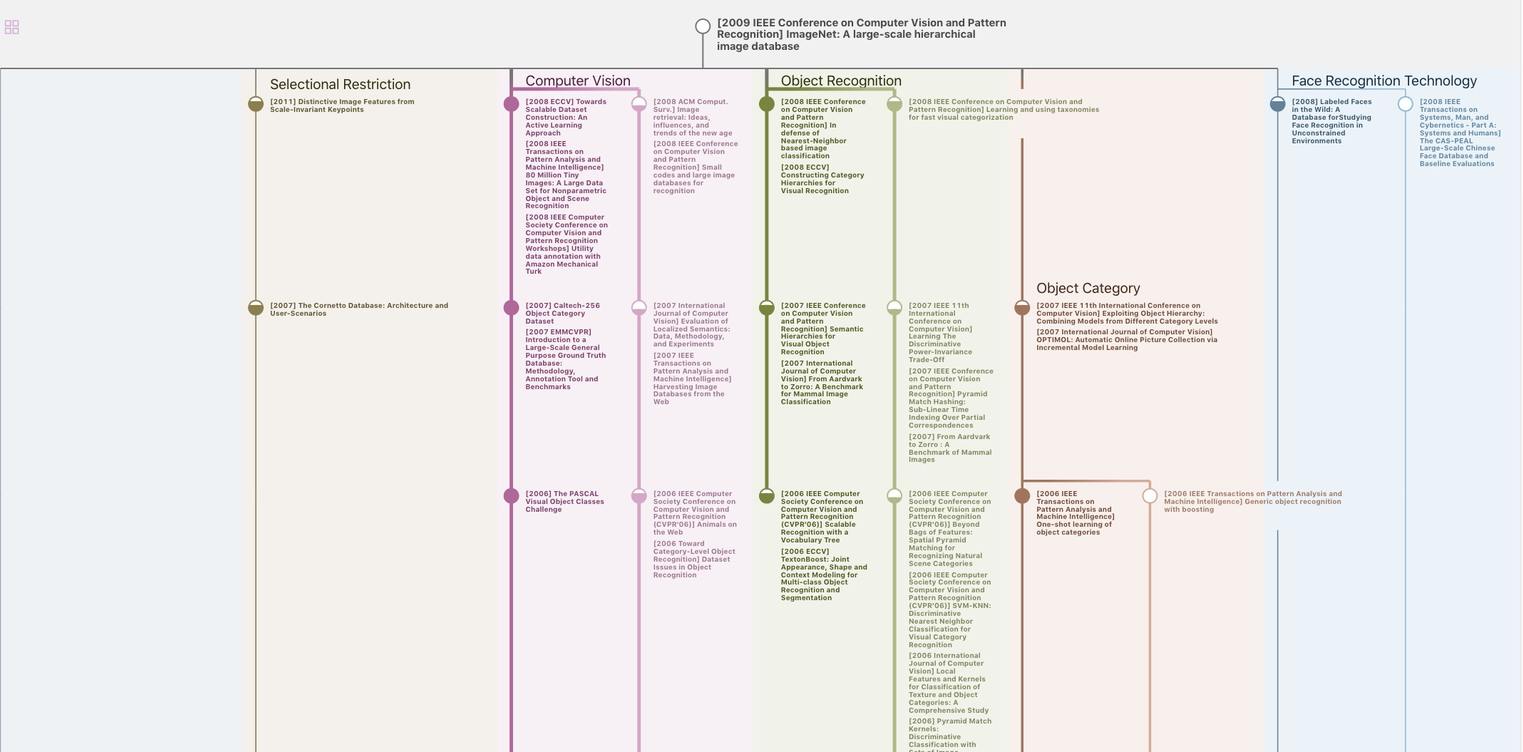
生成溯源树,研究论文发展脉络
Chat Paper
正在生成论文摘要