Challenges on Real-World Skin Lesion Classification: Comparing Fine-tuning Strategies for Domain Adaptation using Deep Learning
semanticscholar(2021)
摘要
Skin lesion diagnosis is a challenging task even for experimented dermatologists. By using a computer-assisted diagnostic tool, misdiagnosed skin lesions are likely to decrease. Deep neural networks have emerged in recent years due to the increased computational power and their generalization capacity for new data. The major drawback of training a network is that it requires large amounts of data, often difficult to obtain. In this work, we introduce a real-world dataset of single lesions cases composed by clinical images, particularly challenging due to image variations (scale, size, point of view, acquisition device) and data imbalance. To tackle these challenges, we propose a domain adaptation approach by pre-training on large, general, datasets, such as ImageNet and fine-tuning on public datasets of clinical dermatological images. This approach is also compared with one where the target dataset is enriched with samples of public datasets. The overall performance obtained for this realworld dataset was not ideal, with F1-scores below 45%. However, interesting conclusions could be drawn on how fine-tuning generally yields better aggregated results (marginal increase of F1score), although some specific categories benefit from increased training samples in a merged dataset. These results pave the way for new strategies towards the real-world application of skin lesion classification models, moving forward from more controlled settings, where results are typically impressive, however not yet translatable into practice.
更多查看译文
AI 理解论文
溯源树
样例
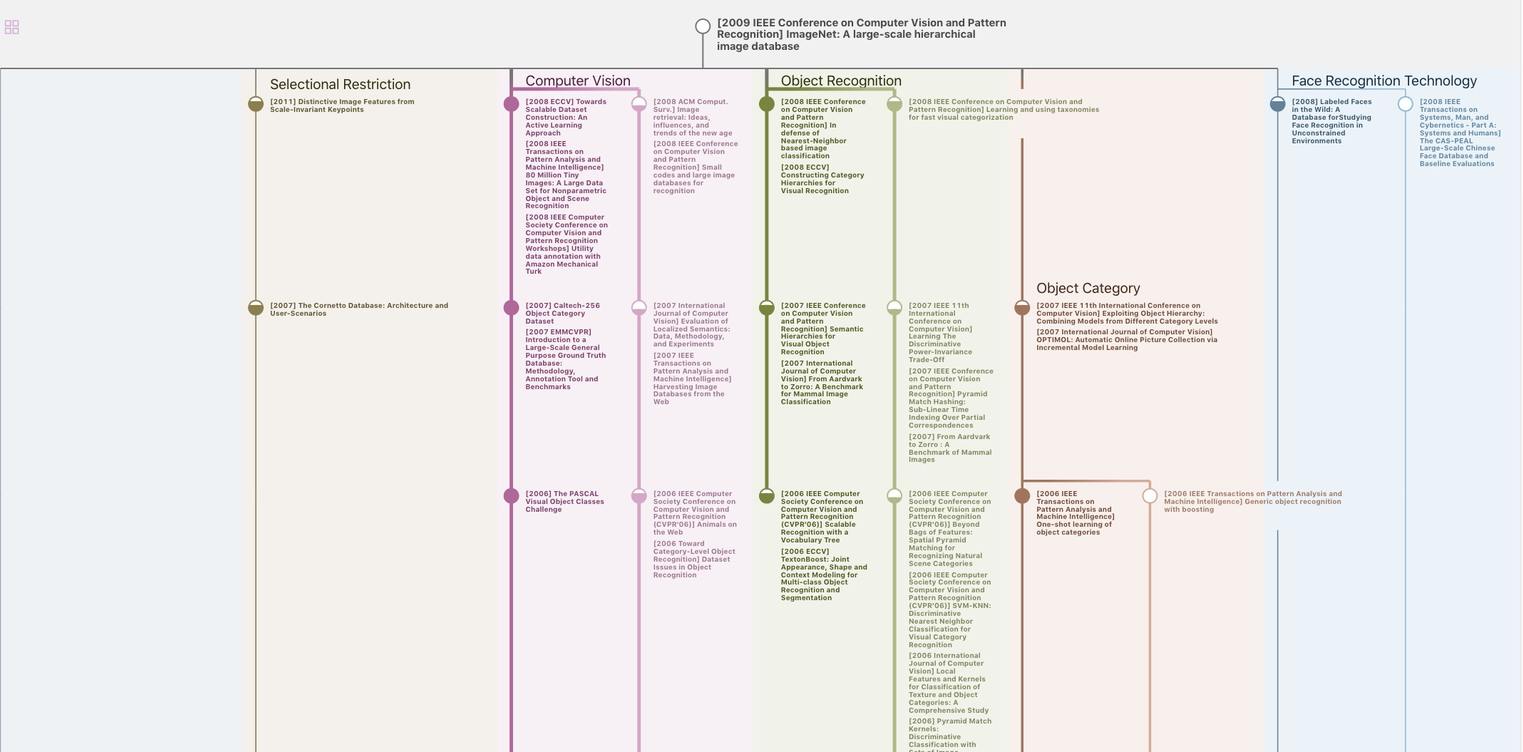
生成溯源树,研究论文发展脉络
Chat Paper
正在生成论文摘要