A Study of the Effect of Dropout on Imbalanced Data Classification using Deep Neural Networks
semanticscholar(2018)
摘要
Learning from real-world data and classifying them according to their characteristics is an active area of research in machine learning. However, the imbalanced nature of the real-world data is still a challenging task whether it is a binary class or a multi-class problem. In recent years, the use of Deep Neural Network (DNN) for classification has been very popular among different fields. Although DNN is suitable to handle complex classification tasks, coadaptation among the units of the DNN model leads to overfitting. Therefore, various regularization techniques have been introduced to reduce overfitting and improve generalization. In this paper, a study is conducted to investigate the impact of the Dropout rates of the DNN with imbalanced data. To our best knowledge, there is not much prior study on this. In this study, the imbalanced data is balanced using three popular techniques, and thereafter the Dropout regularization technique is applied. With the use of the evaluation metric ROC AUC (Receiver Operating Characteristic Area Under Curve), this study has identified the impact of the dropout rates for imbalanced data classification using DNNs. Keywords— Deep Neural Networks, Dropout, Imbalanced data
更多查看译文
AI 理解论文
溯源树
样例
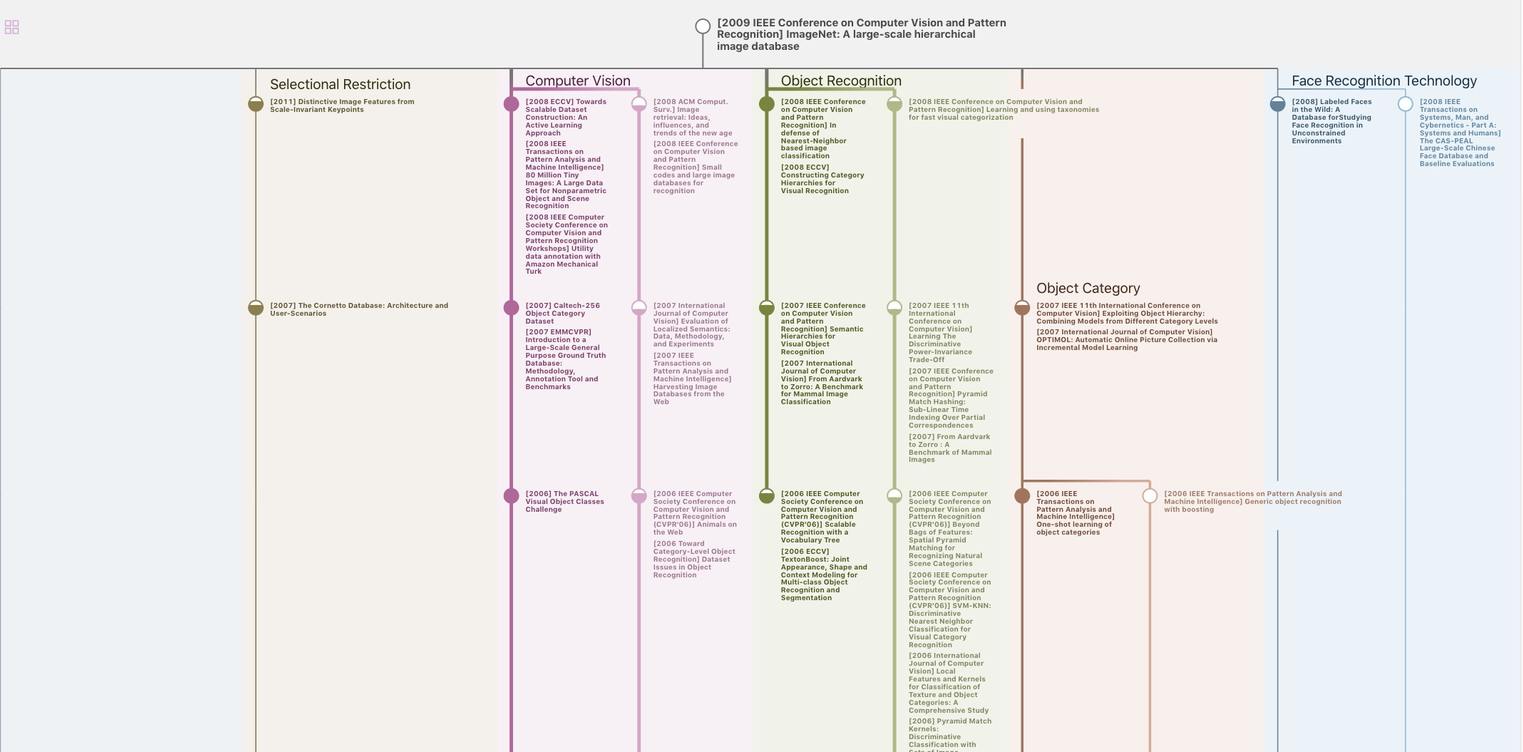
生成溯源树,研究论文发展脉络
Chat Paper
正在生成论文摘要