On Quasi-Deterministic mmWave Channel Modeling using Raytracer: Performance Analysis with Deterministic and Random Rays
semanticscholar(2020)
摘要
With the advent of next generation wireless technologies, Quasi-Deterministic (Q-D) channel modeling is essential to accurately represent the unique propagation characteristics of millimeter Wave (mmWave) links (i.e., severe propagation losses, almost no diffraction, etc.) in both indoor as well as outdoor scenarios. In Q-D model, the channel is characterized with strong Deterministic rays (D-rays) coming from the reflections of the common objects (e.g., ground) in the environment, and relatively weaker Random rays (R-rays) caused by the random objects present in the environment. In order to model the random objects in a given environment, the IEEE 802.11ay channel modeling group proposed two different methods, namely, statistical and deterministic approach. The deterministic approach treats random objects the same as main objects by generating the specular reflections using the geometrical optics-based raytracing and the diffused paths from the statistical parameters extracted from channel measurements. Whereas, the statistical approach generates R-rays purely statistically without the knowledge of the random objects. In this study, we consider both R-ray modeling approaches to generate R-rays along with D-rays for three scenarios: indoor lecture-room, outdoor parking-lot and streetcanyon using real measurement based Q-D models. Further, we systemically compare the performance of these two approaches with respect to computation complexity, channel statistics, and the corresponding link-level performance. This extensive performance analysis also provides several important insights that are lacking in the existing literature. Our evaluation shows that on the expense of computation complexity, the deterministic approach can model the channel closer to a specific scenario and provide more accurate link-level performance, while the statistical approach has significantly lower computation complexity but results in different Physical Layer (PHY) performance.
更多查看译文
AI 理解论文
溯源树
样例
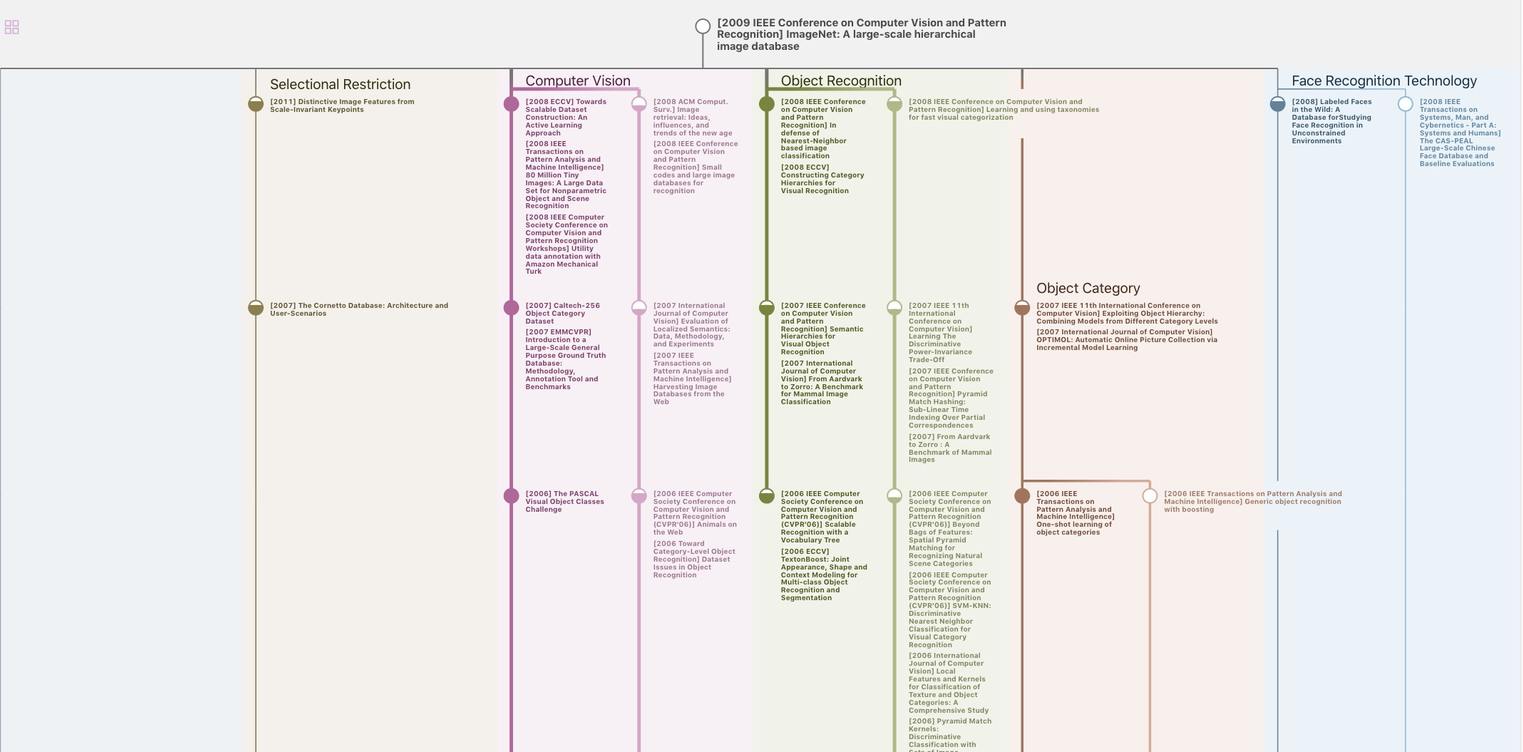
生成溯源树,研究论文发展脉络
Chat Paper
正在生成论文摘要