of : Median bed-material sediment particle size across rivers in the contiguous U
EARTH SYSTEM SCIENCE DATA(2021)
摘要
The authors have attempted to solve an important problem of increasing resolution of available sediment D50 in contiguous USA. The authors should be lauded for attempting to tackle this problem using a unique approach of predicting D50 for regions that lacked measurements using a Gradient-Boosting based machine learning method. The paper is well written, though the presentation can be improved. The approach taken by the authors is unique, though I don’t think the resultant synthetic dataset generated meets the standards of the measured datasets usually published in this journal. I say this because, it is well known that a ML model’s predictive capability is constrained by the range of values present within the training data-set. Even if a trained model shows decent prediction capability for the testing data-set, there is no guarantee that the model will be able to predict correctly for cases that have input parameter values beyond the range of the training data. When the authors synthetically generated the data for the whole US using a model trained using (extremely sparse) 2577 spatial locations, they didn’t mention about the range of values for the input parameters in the 26000 flowlines, which were later used to generate the synthetic dataset. I would recommend the authors to submit this paper to a different journal, e.g. WRR, Advances in Water Resources, Geoscientific Model Development, JGRE, etc., and increase the discussion about why the 13 parameters that SHAP value indicate to be the ones most responsible for determining the D50 of sediment at a stream location.
更多查看译文
AI 理解论文
溯源树
样例
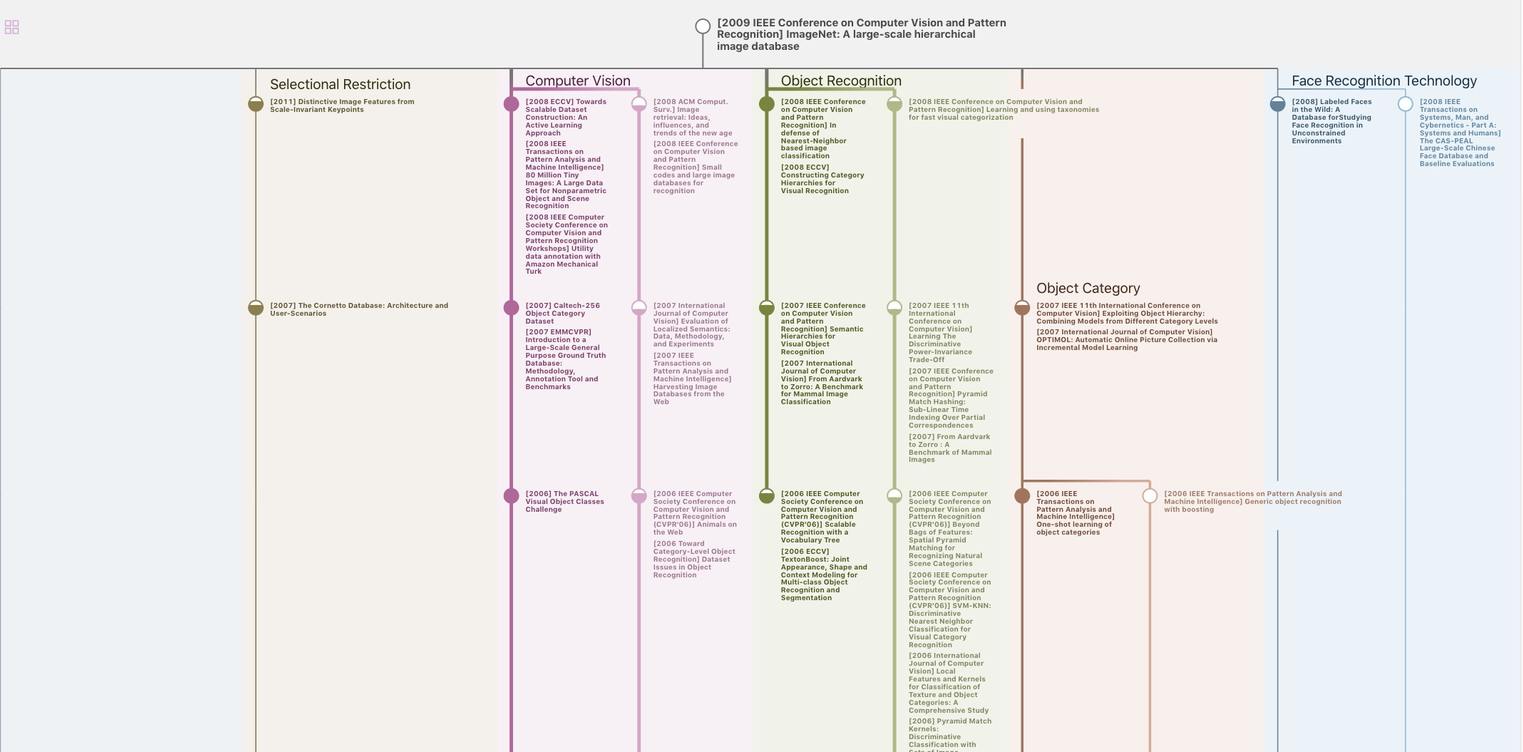
生成溯源树,研究论文发展脉络
Chat Paper
正在生成论文摘要