Effect of PCA Feature Reduction on Ventricular Ectopic Beat Classification
International Journal of Engineering and Advanced Technology(2019)
Abstract
Due to the cardiac diseases called Ventricular Tachycardia (VT) and Ventricular Fibrillation (VF) causes sudden cardiac death, it is crucial to recognize Ventricular Ectopic Beats (VEB) in Electrocardio- gram for the early diagnosis. There are many algorithms proposed earlier to classify the VEBs. Even though those algorithms achieved good accu- racy, the size of the feature set is large and not precise. In addition, earlier algorithms used feature reduction methods for reducing the feature set. Therefore, in this paper, we extracted only five features namely, Pre RR interval, post RR interval, QRS duration, QR slope, and RS slope. Later, we applied the Principle Component Analysis (PCA) for reducing the size of the feature set to observe the effect of Feature Reduction (FR) on the accuracy of VEB Classification. We applied different classifiers for classifying the cardiac beats in to normal and VEBs. Finally, using K-means Nearest Neighborhood (KNN) classifier and cubic Support Vec- tor Machine (SVM) classifier, we achieved 97.4%classification accuracy, 98.38%, 88.89% & 98.37% sensitivity, specificity & positive predictivity respectively. In addition, it is observed that by applying PCA-FR, the classification accuracy was reduced by a maximum of 3.7%.
MoreTranslated text
AI Read Science
Must-Reading Tree
Example
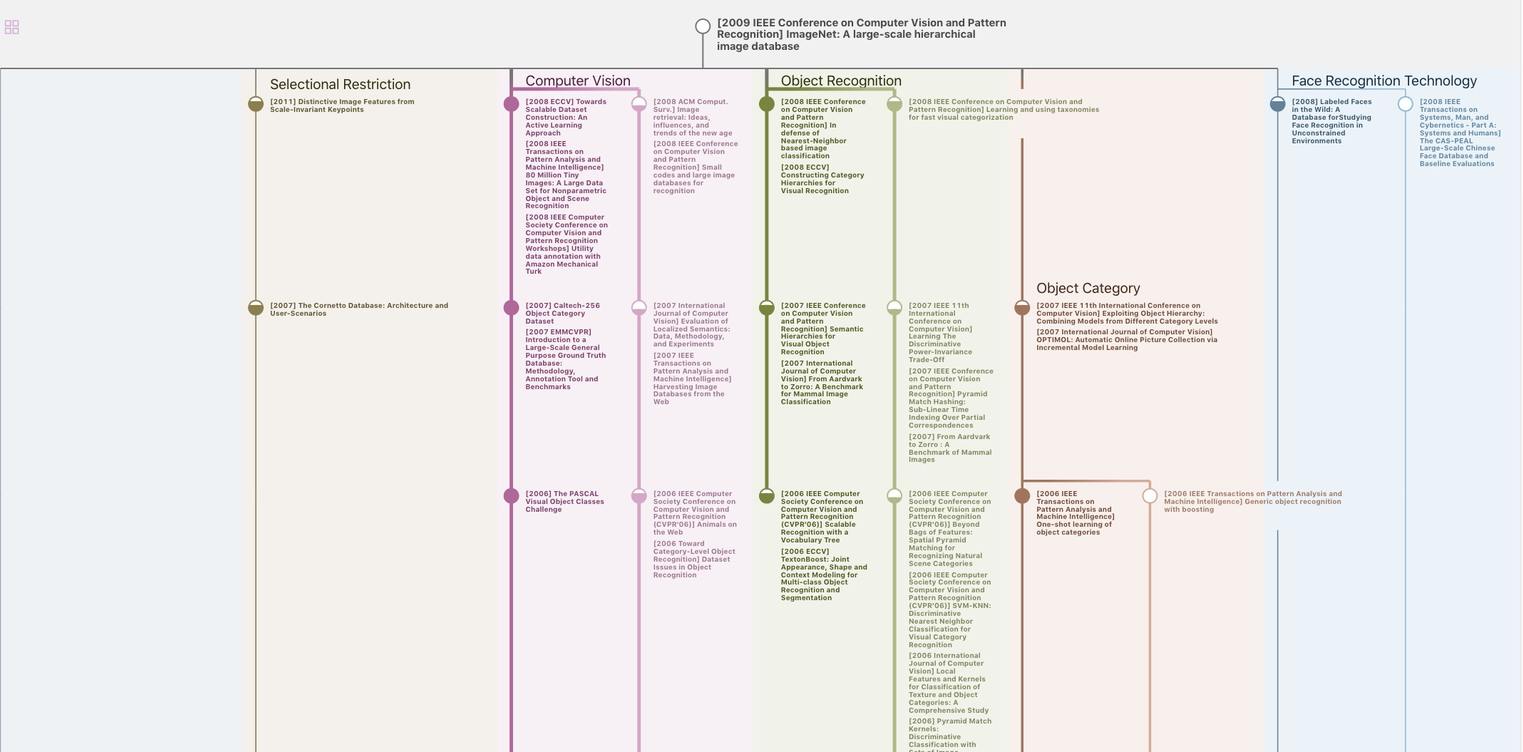
Generate MRT to find the research sequence of this paper
Chat Paper
Summary is being generated by the instructions you defined