Method of oil and gas reservoir detection based on geological knowledge distillation learning
Scientia Sinica Informationis(2020)
Abstract
Oil and gas reservoir detection is one of the major tasks of petroleum energy companies in the exploration and production process. The oil and gas industry has long relied on the expert manual analysis of massive logging data to perform qualitative analyses of oil and gas reservoirs. Although experts’ interpretations are highly accurate, the time and economic costs are considerably high. With the rapid development of artificial intelligence technologies such as deep learning in recent years, intelligent oil and gas reservoir detection methods have become a focus in the academia and industry. However, sensor data in real industrial scenarios present serious inconsistencies, which bring great challenges to traditional supervised learning models. This paper presents a focused study on the oil and gas reservoir detection task in the context of sensor inconsistencies and proposes a geological knowledge distillation multiscale network approach. This method proposes a multiscale feature fusion mechanism based on self-attention to learn the multiscale dynamic representation of geological information. Then, the model designs a geological knowledge distillation learning framework to learn additional geological knowledge from inconsistent sensor data. This step further improves the model’s accuracy. A large number of experiments on real industrial datasets are subsequently performed. The results fully prove the effectiveness and robustness of the proposed model in oil and gas reservoir detection.
MoreTranslated text
AI Read Science
Must-Reading Tree
Example
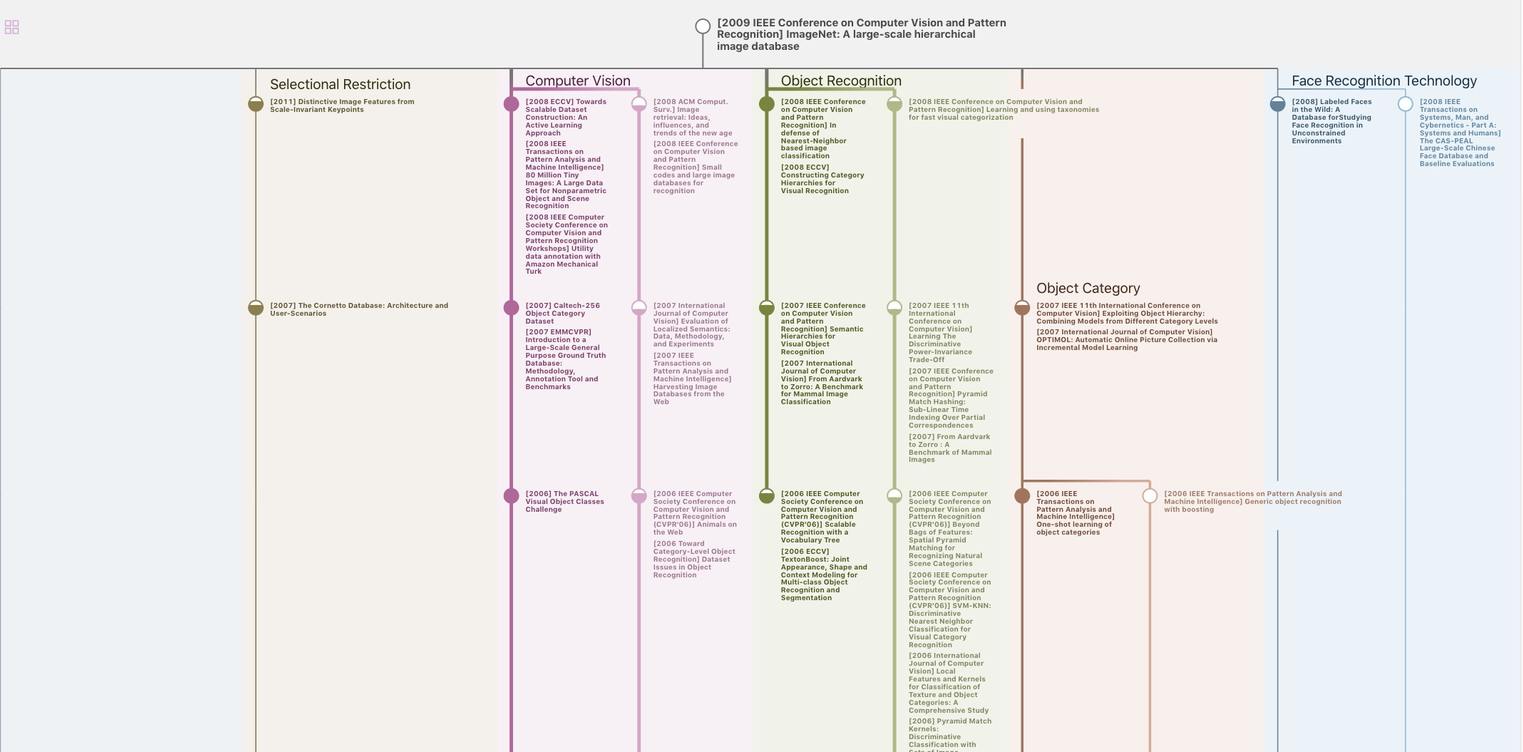
Generate MRT to find the research sequence of this paper
Chat Paper
Summary is being generated by the instructions you defined