Online and transparent self-adaptation of stream parallel patterns
COMPUTING(2021)
摘要
Several real-world parallel applications are becoming more dynamic and long-running, demanding online (at run-time) adaptations. Stream processing is a representative scenario that computes data items arriving in real-time and where parallel executions are necessary. However, it is challenging for humans to monitor and manually self-optimize complex and long-running parallel executions continuously. Moreover, although high-level and structured parallel programming aims to facilitate parallelism, several issues still need to be addressed for improving the existing abstractions. In this paper, we extend self-adaptiveness for supporting autonomous and online changes of the parallel pattern compositions. Online self-adaptation is achieved with an online profiler that characterizes the applications, which is combined with a new self-adaptive strategy and a model for smooth transitions on reconfigurations. The solution provides a new abstraction layer that enables application programmers to define non-functional requirements instead of hand-tuning complex configurations. Hence, we contribute with additional abstractions and flexible self-adaptation for responsiveness at run-time. The proposed solution is evaluated with applications having different processing characteristics, workloads, and configurations. The results show that it is possible to provide additional abstractions, flexibility, and responsiveness while achieving performance comparable to the best static configuration executions.
更多查看译文
关键词
Autonomic systems, Parallel programming, Parallelism abstractions, Self-adaptive software, Stream processing
AI 理解论文
溯源树
样例
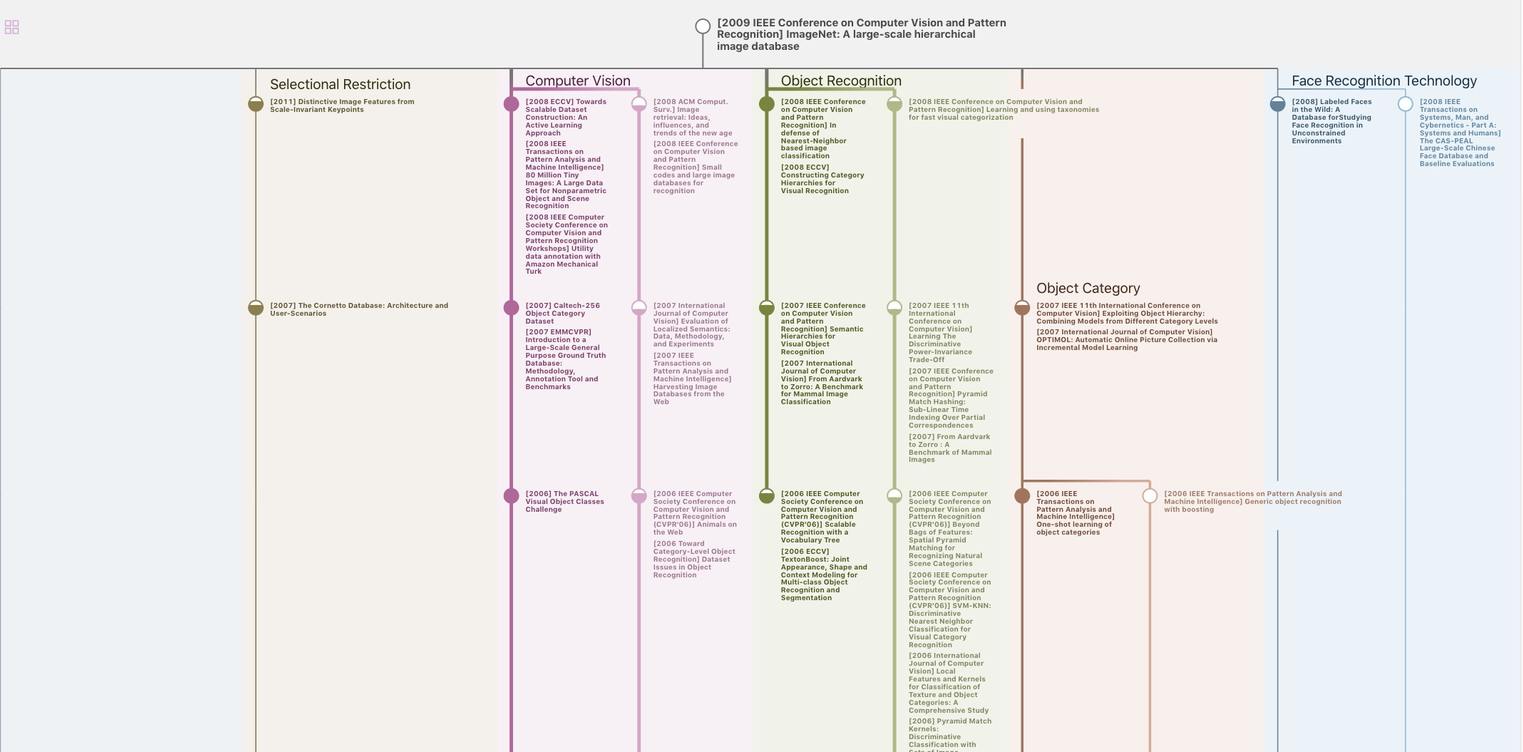
生成溯源树,研究论文发展脉络
Chat Paper
正在生成论文摘要